Regional to Local Machine-Learning Analysis for Unconventional Formation Reserve Estimation: Eagle Ford Case Study
Day 2 Tue, October 27, 2020(2020)
摘要
Abstract Unconventional tight reservoirs currently make up more than 60% of domestic oil and gas production in the United States. However, developing unconventional formations requires intensive drilling and completion campaigns to maintain steady production of a field. Therefore, the prediction of estimated ultimate recovery, which measures the producible reserve from a well, is demanding, particularly as operators becomes more rational under the current volatile market conditions. Despite unconventional reservoirs being considered a resource play with low geological risks, their economic appraisal is challenged by unknown stimulation outcomes and intricate producing mechanisms. Therefore, this work aimed to leverage machine-learning techniques with big data to analyze the multivariant relationship of geological and engineering parameters with unconventional reservoir production and to improve the prediction of estimated ultimate recovery in unconventional formations. In this case study, a multiscale machine-learning workflow was deliberated and applied to a big data set from the Eagle Ford shale. First, quality control and feature selection were performed on a data set consisting of 4,067 wells with 30+ geophysical, petrophysical, drilling and completion, and production features. Then, a regional inferencing model, based on a K-nearest neighbor with bagging algorithm, was trained to obtain the spatial trend of estimated ultimate recovery across the Eagle Ford formation. The last part of the analysis was to build a local-scale prediction model. With the study area confined to East Texas, a random forest regression was performed to rigorously predict oil and gas estimated ultimate recoveries. The selected training features were finalized based on the results of a higher-dimension regression, as well as domain knowledge. Overall, the data-driven model trained with physically controlled data captured the production behavior of the Eagle Ford shale. The application of the proposed workflow on the Eagle Ford shale demonstrates a progressive building of the machine-learning model. The quality control of data allows global inspection of the data set and, more importantly, confirms the statistical distribution of training data. This study emphasizes the philosophy of multiscale data analytics. The large-scale model portraying sweet spots using location variables grants direct guidance for acreage acquisition and development across the basin; the small-scale model trained with reduced dimensionality generates quantitative prediction of oil and gas estimated ultimate recoveries for an area of interest. Compared with our previous work using higher dimensionality and extensive spatial interest, this progressive learning maintains similar explained variance in out-bag model check, but grants 26% and 52% reductions in mean square error for predicting oil and gas estimated ultimate recoveries in the Eagle Ford formation. In the end, prediction validation is performed by revisiting the data set. Overall, the proposed workflow demonstrates successful application in the Eagle Ford formation such that it can be directly implemented for other unconventional resource plays.
更多查看译文
AI 理解论文
溯源树
样例
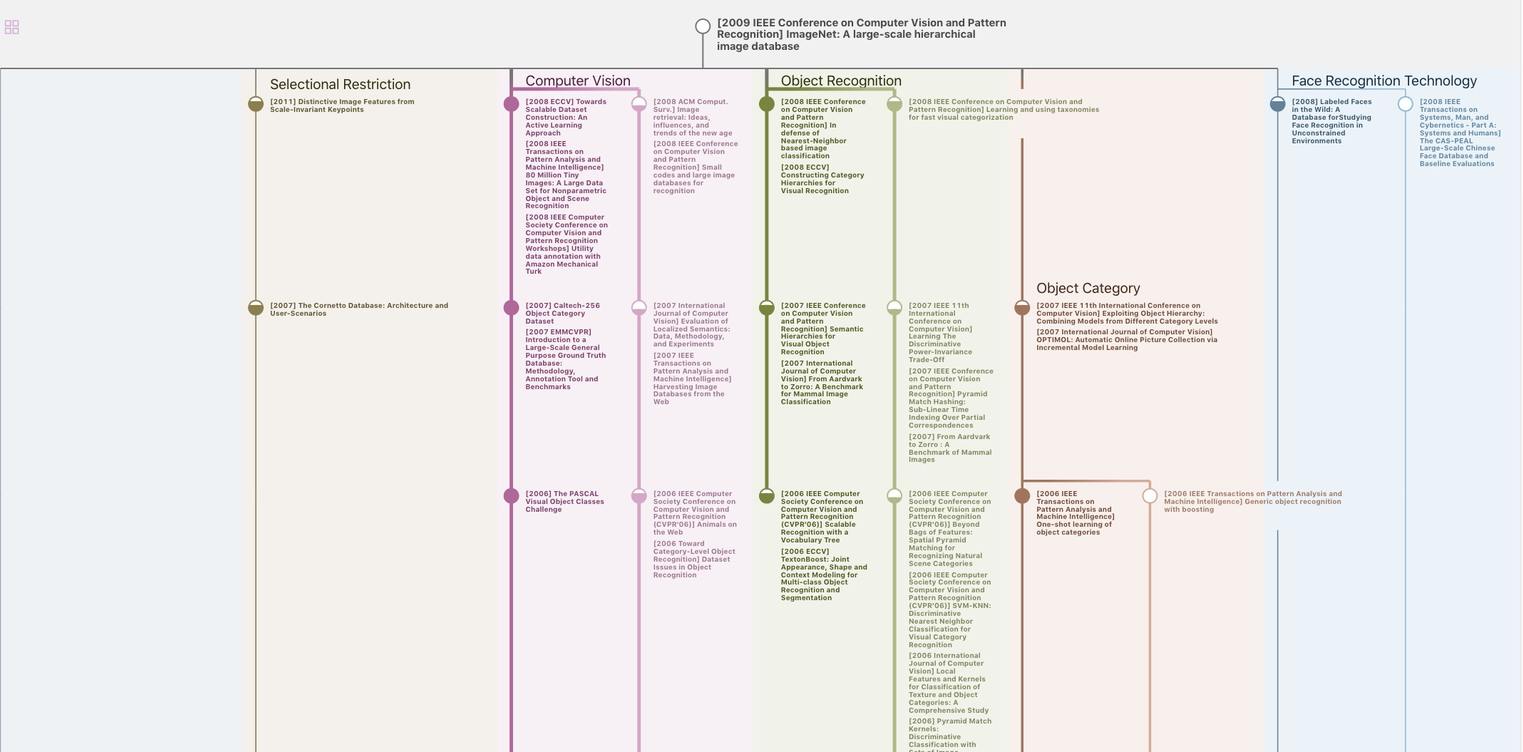
生成溯源树,研究论文发展脉络
Chat Paper
正在生成论文摘要