Automated Lease Operating Statements for Cost Optimization and Reserve Evaluation Using Artificial Intelligence
Day 3 Wed, October 28, 2020(2020)
摘要
Abstract The objective of the present work is to streamline the analysis of Lease Operating Statements (LOS) with advanced learning paradigms from artificial intelligence (AI). The proposed approach aims at the: (a) consolidation of disparate expenses data; (b) timely expense assessment at field, pad or well level; (c) prevention and quick identification of negative cash flow trends (d) robust LOS predictions; and (e) optimal budget planning under uncertainty. To achieve this objective, a LOS acceleration platform was developed for the automatic integration of volume estimation with Lease Operating Expenses (LOE). Historical data from production volumes, revenues, price differentials, LOEs, marketing and transportation costs, taxes, CAPEX, P&A costs and others considered to strengthen the accuracy of individual expense categories and the overall LOS predictive model. The predictive model consists of a combined blend of analytical and machine learning models that allows to reliably forecast trends and proactively detect anomalies that may be negatively affecting the operational cash flow. Intuitive and portable visualizations allow a quick interpretation and communication of results among engineers and managers. The implemented platform fills a gap between traditional LOS analysis and preemptive expense planning involving many wells and expense categories that are hard to track daily. It is shown that the proposed approach can lead to savings of the order of 30% in incurred expenses.
更多查看译文
AI 理解论文
溯源树
样例
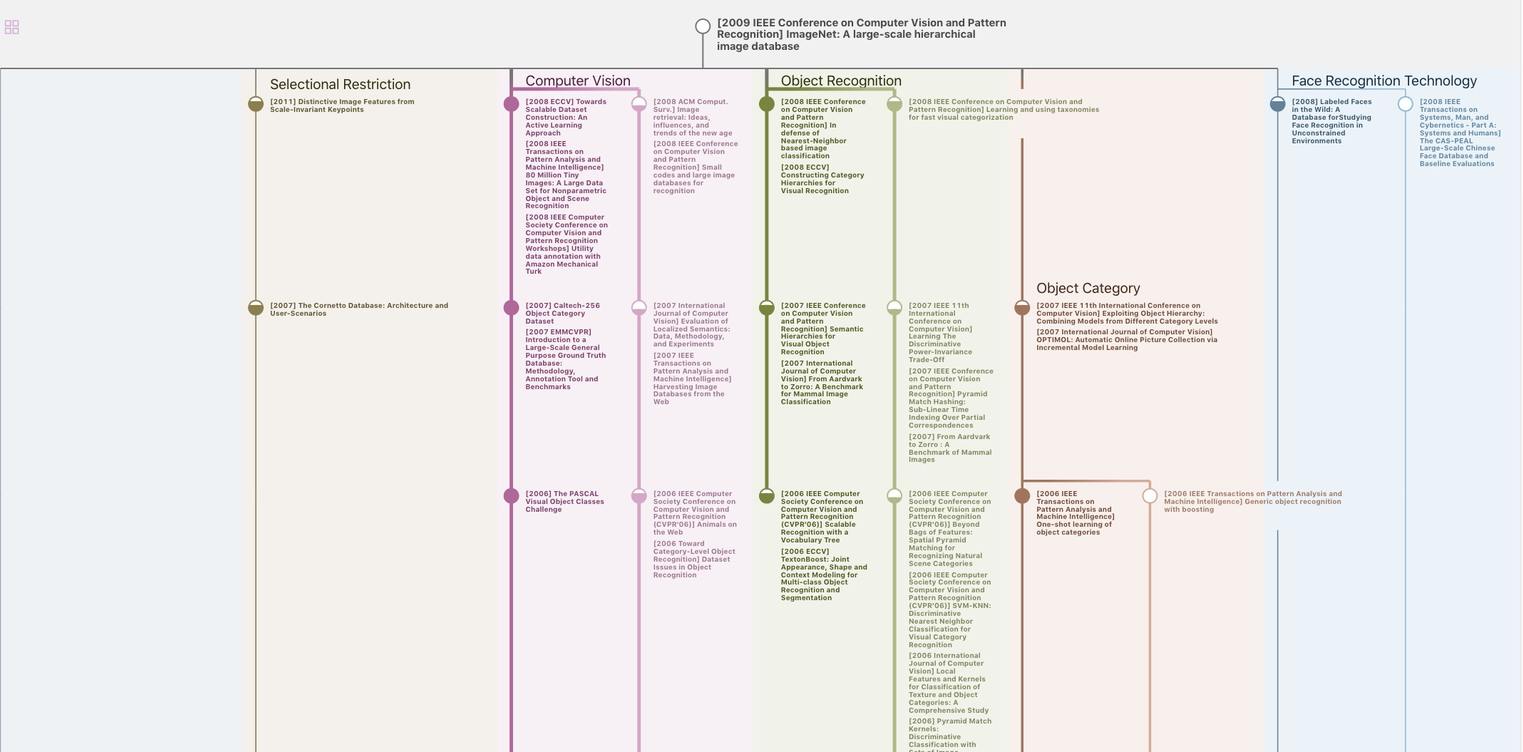
生成溯源树,研究论文发展脉络
Chat Paper
正在生成论文摘要