Learning Time Reduction Using Warm-Start Methods for a Reinforcement Learning-Based Supervisory Control in Hybrid Electric Vehicle Applications
IEEE Transactions on Transportation Electrification(2021)
摘要
Reinforcement learning (RL) is gradually being implemented in the hybrid electric vehicle (HEV) supervisory control. Even though RL exhibits significant fuel consumption saving, the long learning time makes it hardly applicable in real-world vehicles. This study aims to reduce the learning iterations of Q-learning in HEV application utilizing warm-start methods. Different from previous studies, which initiated Q-learning with zero or random Q values, this study initiates the Q-learning with different supervisory controls, and the detailed analysis is given. The results show that the proposed warm-start Q-learning requires 68.8% fewer iterations than cold-start Q-learning and improves 10%–16% MPG compared with equivalent consumption minimization strategy control. The results of this study can be used to facilitate the deployment of RL in vehicle applications.
更多查看译文
关键词
learning time reduction,supervisory control,warm-start,learning-based
AI 理解论文
溯源树
样例
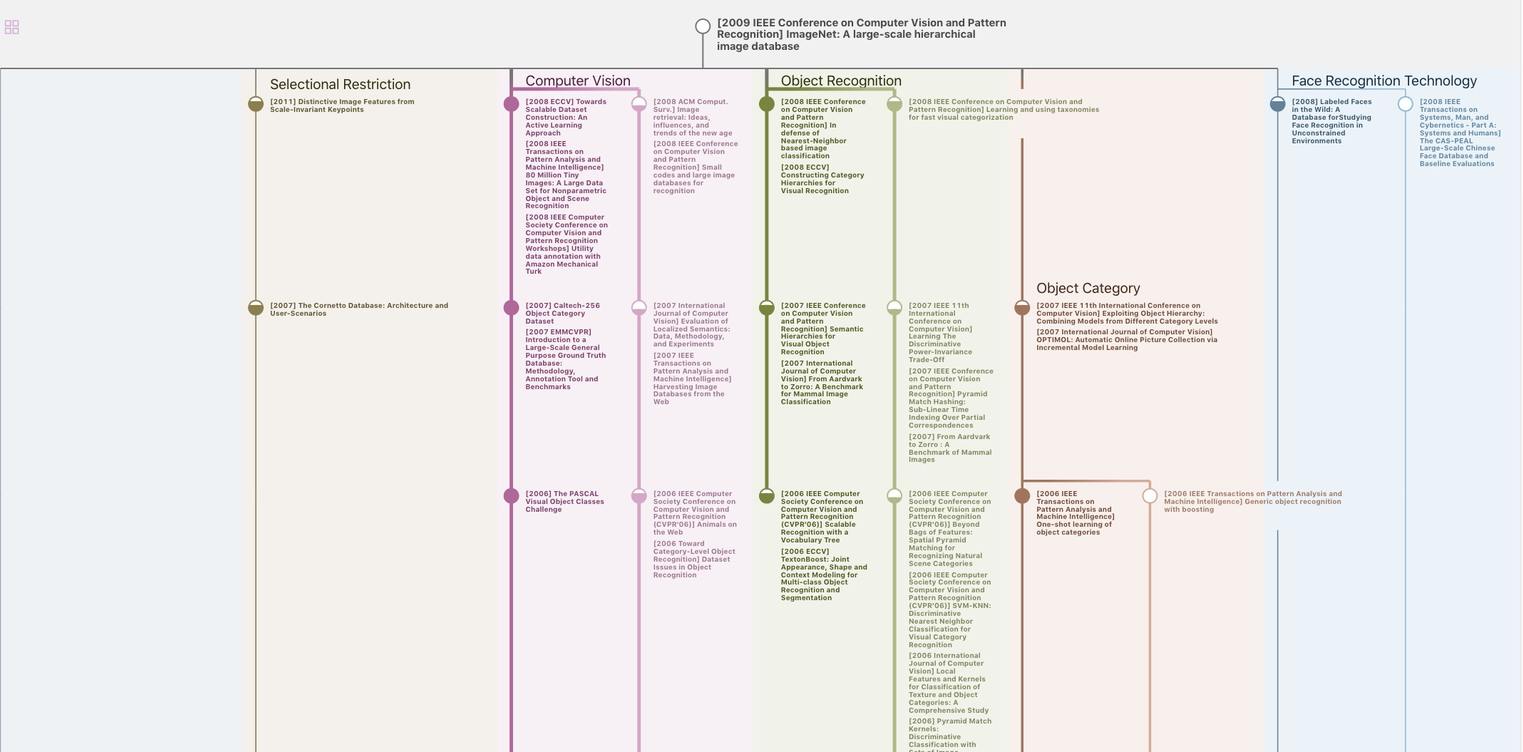
生成溯源树,研究论文发展脉络
Chat Paper
正在生成论文摘要