Deep learning for compressive hyperspectral sensing
Imaging Spectrometry XXIV: Applications, Sensors, and Processing(2020)
摘要
The application of compressive sensing (CS) techniques for the hyperspectral (HS) imaging is very appealing since the acquisition of HS images is demanding in terms hardware and acquisition time, and since the application of CS framework matches well the HS imaging task, which involves capturing huge amount of typically very redundant data. During the last decade, we developed several CS HS imaging systems, which have demonstrated orders of magnitude reduction of the acquisition time and of storage requirements, improved signal-to-noise ratio, and reduction of the systems’ size and weight. In this paper we demonstrate how these systems can further benefit from employing deep learning (DL) tools for post-processing of the compressively sensed hyperspectral data. We overview some DL techniques that we have developed for improving the HS image reconstruction and target detection.
更多查看译文
AI 理解论文
溯源树
样例
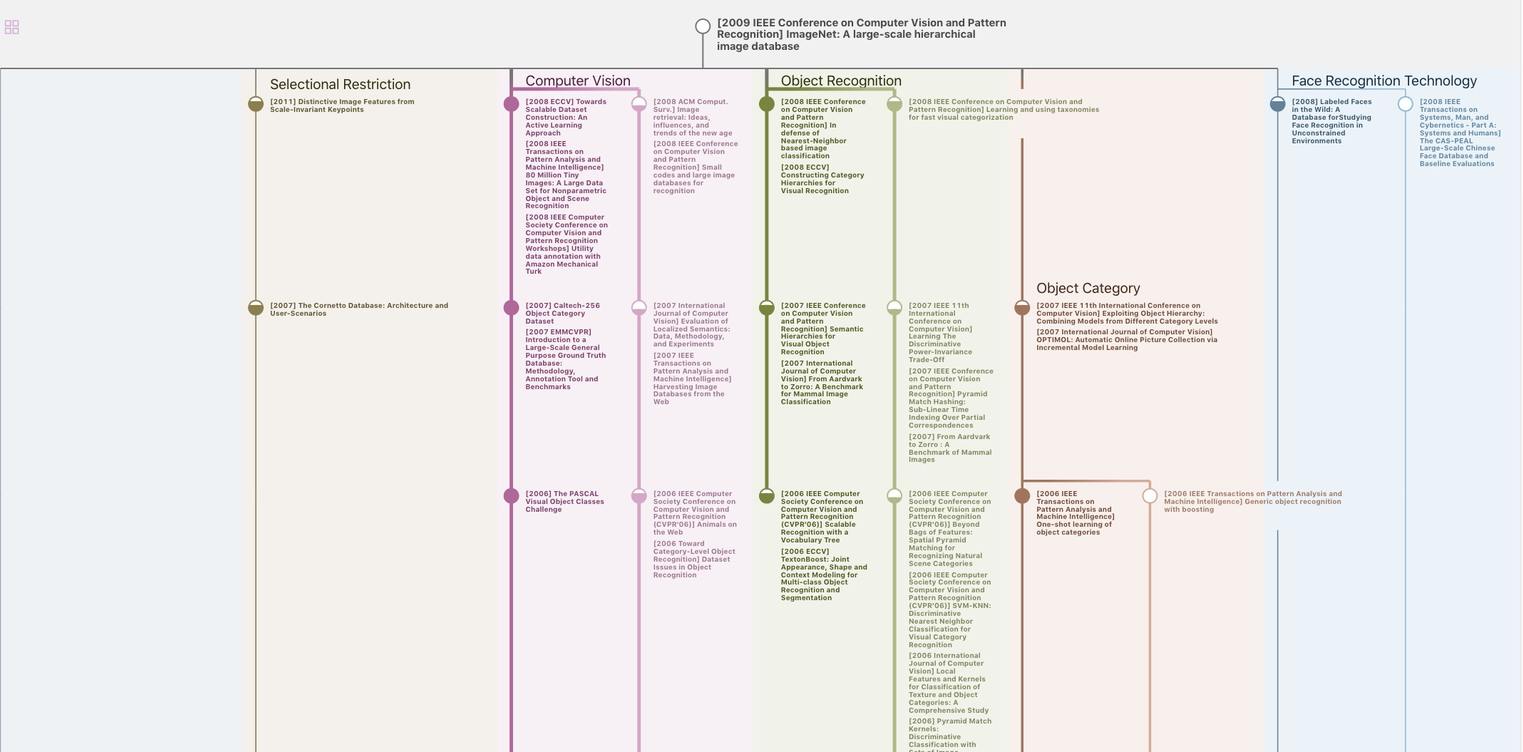
生成溯源树,研究论文发展脉络
Chat Paper
正在生成论文摘要