Recommendations from cold starts in big data
Computing(2020)
摘要
AbstractThis paper examines the challenging problem of new user cold starts in subset labelled and extremely sparsely labelled big data. We introduce a new Isle of Wight Supply Chain (IWSC) dataset demonstrating these characteristics. We also introduce a new technique addressing these challenges, the Transitive Semantic Relationships (TSR) model, which infers potential relationships from user and item text content and few labelled examples. We perform both implicit and explicit evaluation of TSR as a recommender system and from new user cold starts we achieve a hit-rate@10 of 77% on a collection of 630 items with only 376 supply-chain consumer labels, and 67% with only 142 supply-chain supplier labels, demonstrating a high level of performance even with extremely few labels in challenging cold-start scenarios. TSR is suitable for any dataset featuring few labels and user and item content, where similarity of content indicates similar relationship forming capability. TSR can be used as a standalone recommender system or to complement existing high-performance recommender models that require more labels or do not support cold starts.
更多查看译文
关键词
cold starts,recommendations,data
AI 理解论文
溯源树
样例
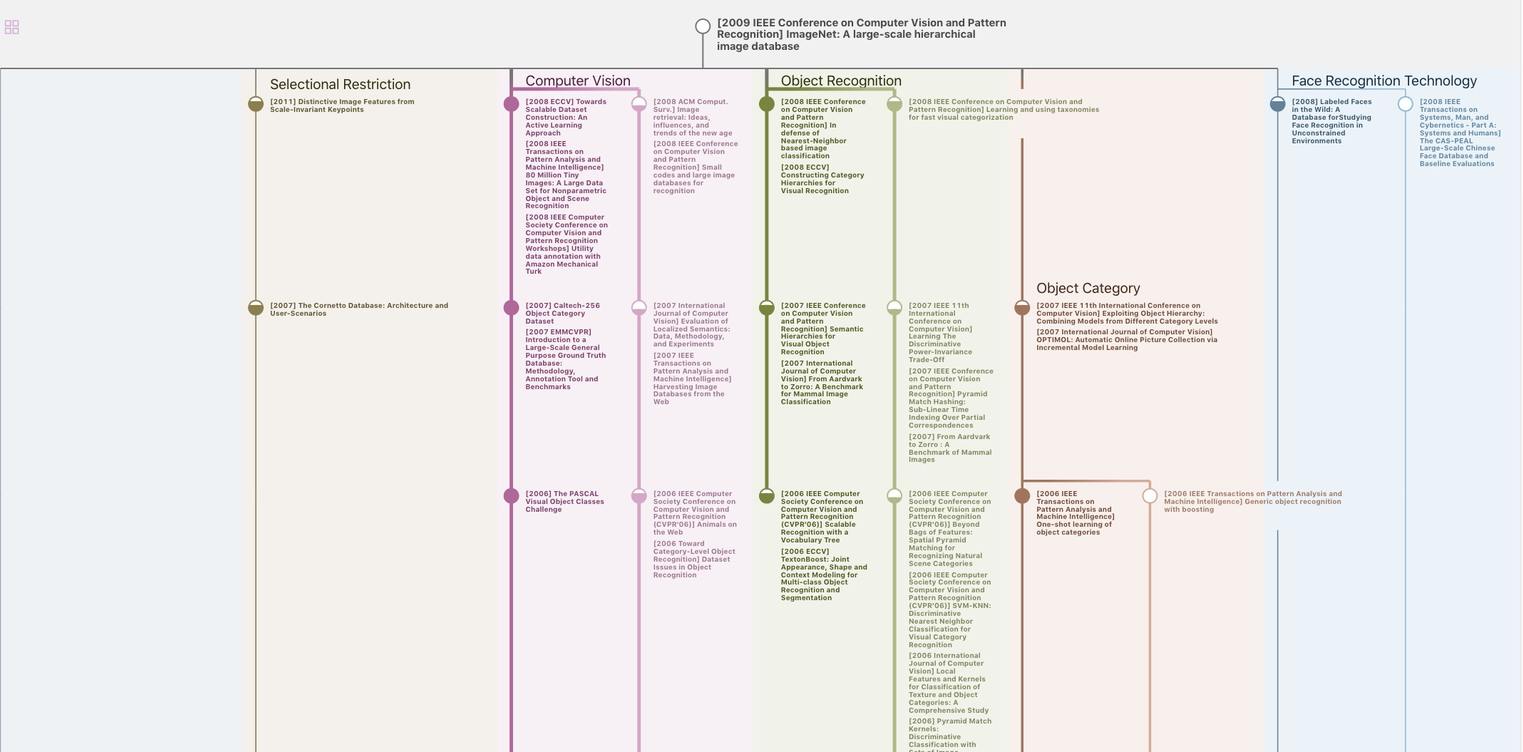
生成溯源树,研究论文发展脉络
Chat Paper
正在生成论文摘要