Fault Diagnosis of MVB Based on Random Forest and Ensemble Pruning
Lecture Notes in Electrical EngineeringProceedings of the 4th International Conference on Electrical and Information Technologies for Rail Transportation (EITRT) 2019(2020)
摘要
Due to the complex working environment and lack of network management protocol, it is difficult to comprehensively monitor the operating states and diagnose the faults of the Multifunction Vehicle Bus (MVB). In this paper, an MVB fault diagnostic method based on physical waveform features and ensemble pruning has been proposed. Firstly, MVB waveforms of the bus administrator node (BA) in normal and fault conditions are sampled by an MVB analyzer based on high-speed A/D sampling technology. Network features are extracted from the waveforms, and a random forest (RF) classifier has been trained to classify different MVB faults. An ensemble pruning method based on diversity index and the k-mean algorithm has been proposed to reduce the number of decision trees and improve the ensemble performance. The experimental results show that the proposed feature extraction method and ensemble pruning classifier can recognize the MVB faults correctly, reduce the number of base classifiers, and improve the accuracy of the subforest compared with the original RF ensemble.
更多查看译文
关键词
Fault diagnosis,Multiple vehicle bus,Ensemble pruning
AI 理解论文
溯源树
样例
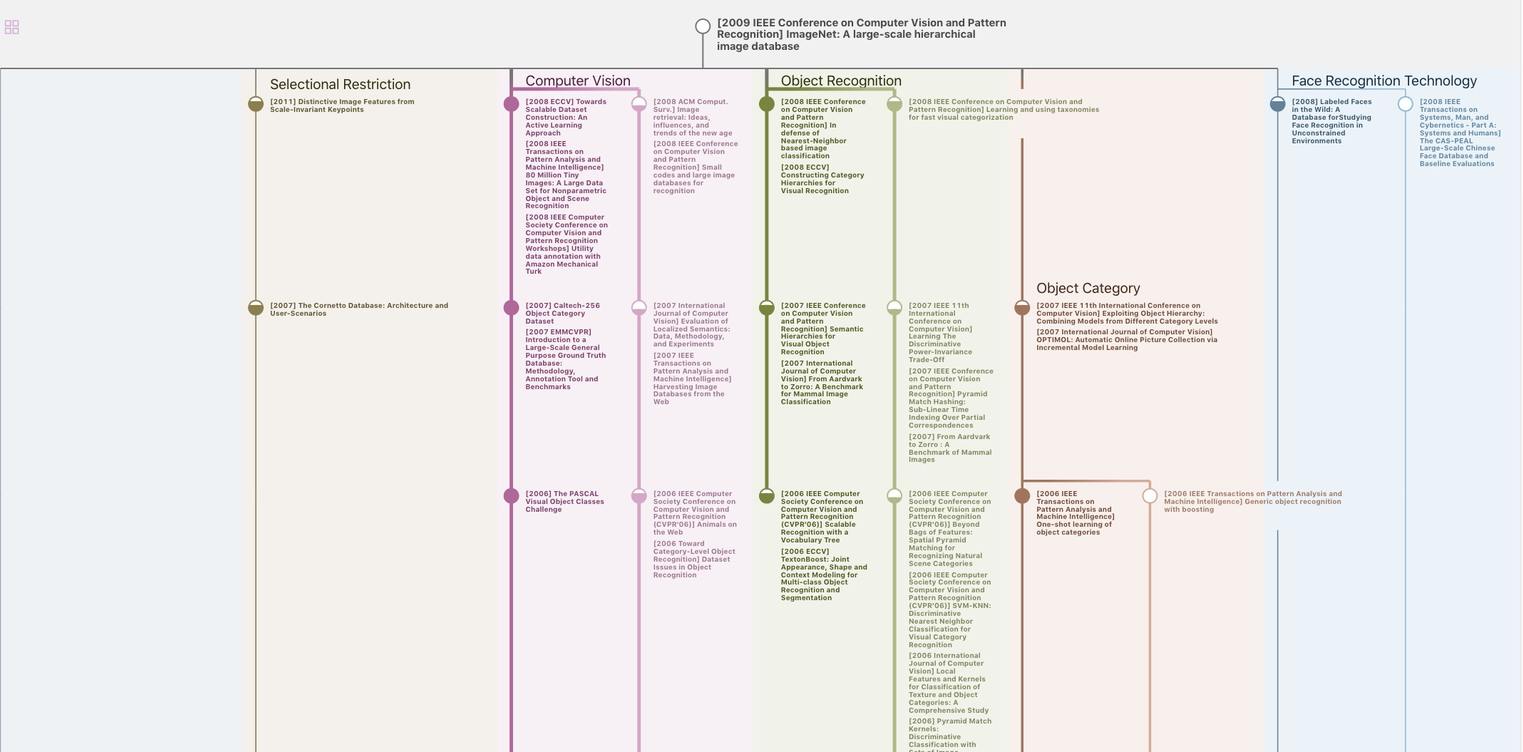
生成溯源树,研究论文发展脉络
Chat Paper
正在生成论文摘要