A CLAIM Approach to Understanding the PointNet
Proceedings of the 2019 2nd International Conference on Algorithms, Computing and Artificial Intelligence(2019)
摘要
Recently, a series of works directly process point clouds and have achieved superior performance in various 3D tasks. However, they are ignorant of the understanding and interpretation of decisions made by these 3D deep learning models. In this work, we focus on explaining the PointNet [1], the first deep learning framework to directly handle 3D point clouds. We propose a class attentive interpretable mapping (CLAIM) approach to visualize and understand what has been learned inside the PointNet. CLAIM quantifies the impact of each point during the decision-making process and indicates the discriminative point set regions used by the PointNet to identify the category. To conduct such a quantitative explanation of the PointNet, CLAIM adds a global average pooling layer after the per-point feature extraction module of PointNet and pass the output to a fully-connected layer that produces the categorical output. As such, CLAIM can associate the global feature directly with categorical scores so that to explore the decision-making process of the PointNet. The experiments on ModelNet40 demonstrate the efficacy of our work for getting better understanding the decision-making process of PointNet and the verification experiments further confirm the interpretability of the proposed method.
更多查看译文
AI 理解论文
溯源树
样例
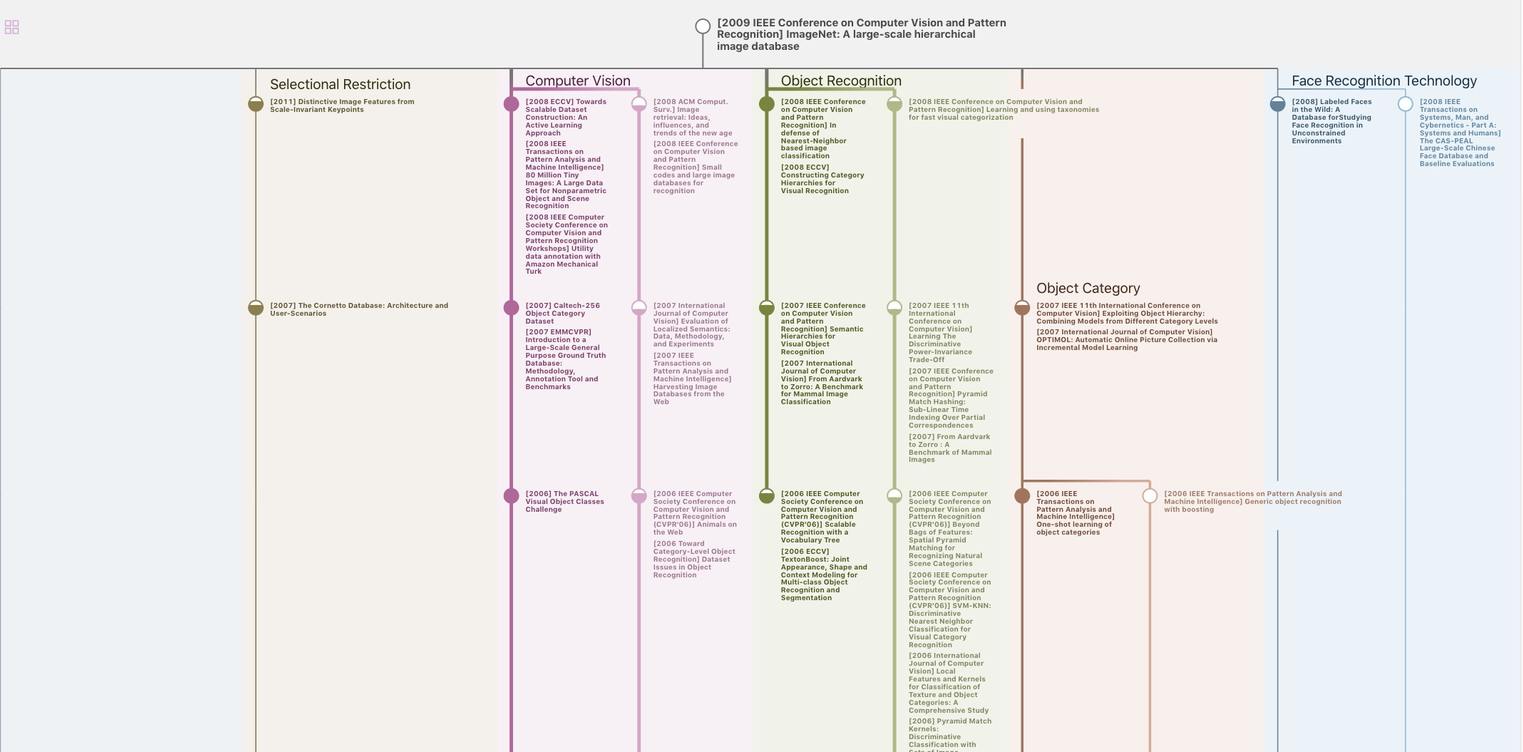
生成溯源树,研究论文发展脉络
Chat Paper
正在生成论文摘要