Deep Learning for Well Data History Analysis
Day 1 Mon, September 30, 2019(2019)
摘要
Abstract The rapid development of machine learning algorithms and the massive accumulation of well data from continuous monitoring has enabled new applications in the oil and gas industries. Data gathered from well sensors are a foundation of the oilfield digitization and data-driven analysis. Here, we describe a deep learning approach to predict the long-term well performance based on a moderate duration of well monitoring data. In this study, we first developed the data processing procedures for oilfield time series data and determined the proper selection of data sampling frequency, parameter combinations and data structures for deep learning models. Then we explored how Deep Learning (DL) models can be employed for well data analysis and how can we combine physics and DL models. Recurrent Neural Network (RNN) is a type of sequential DL model, which can be utilized for time series data analysis. This approach preserves preceding information and yields current response with memory of prior well behavior. Two candidate RNN models were tried to determine how well they were able to improve the accuracy and stability of well performance estimates. These two methods are Gated Recurrent Unit (GRU) and Long Short Term Memory (LSTM). In addition, a novel combination of RNN with Convolutional Neural Networks (CNNs), Long- and Short-term Time-series network (LSTNet), was also investigated. These various models were tested and compared based on the public production datasets from Volve Field. Both GRU and LSTM achieved higher accuracy in performance prediction compared to the simple RNN. In the case of frequent well shut-in and opening, the failure in capturing fast pressure responses and the extreme fluctuations with the simple RNN ultimately leads to high error. In contrast, LSTNet is more stable to frequent or significant well variations. With advanced deep learning structures, engineers can interpret long-term reservoir performance information from responses estimated by deep learning models, instead of performing costly well tests or shut-ins.
更多查看译文
AI 理解论文
溯源树
样例
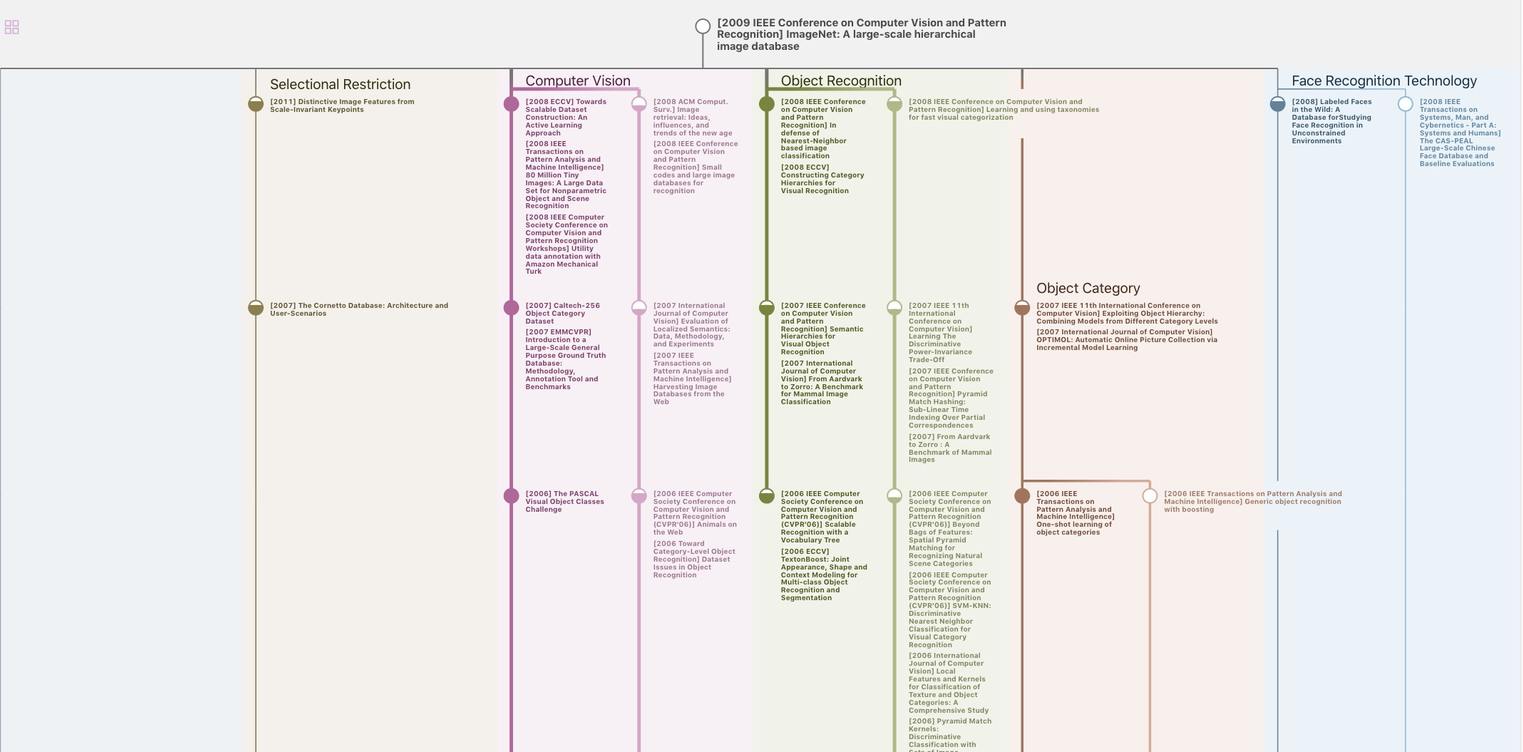
生成溯源树,研究论文发展脉络
Chat Paper
正在生成论文摘要