Deriving Permeability and Reservoir Rock Typing Supported with Self-Organized Maps SOM and Artificial Neural Networks ANN - Optimal Workflow for Enabling Core-Log Integration
Day 2 Wed, September 18, 2019(2019)
摘要
Abstract Permeability and rock typing are two of the main outputs generated from the petrophysical domain and are particularly contributors to the highest degree of uncertainty during the history matching process in reservoir modeling, with the subsequent high impact in field development decisions. Detailed core analysis is the preferred main source of information to estimate permeability and to assign rock types; however, since there are generally more un-cored than cored wells, logs are the most frequently applied source of information to predict permeability and rock types in each data point of the reservoir model. The approach of this investigation is to apply data analytics and machine learning to move from the core domain to the log domain and to determine relationships to then generate properties for the three-dimensional reservoir model with proper simulation for history matching. All wells have a full set of logs (Gamma Ray, Resistivity, Density and Neutron) and few have routine core analysis (Permeability, Porosity and MICP). On a first pass, logs from selected wells are classified into Self Organizing Maps (SOM) without analytical supervision. Then, core data is used to define petrophysical groups (PG), followed by linking the PG's to NMR pore-size distribution analysis results into pre-determined standard pore geometry groups, in this step supervised PGs are generated from the log response constrained by the relationship between pore-throat geometry (MICP) and pose-size distribution (NMR). Permeability-porosity core relationships are reviewed by sorting and eliminating the outliers or inconsistent samples (damaged or chipped, fractures or with local features). After that, the supervised PGs are used to train and calibrate a supervised neural network (NN) and permeability and rock type's relationships can be captured at log scale. Using dimensionality reduction improves the neural network relationships and thus data population into the petrophysical wells. The result is a more robust model capable to capture over 80% of the core relationships and able to predict permeability and rock types while preserving the geological features of the reservoir. The application of this method makes possible to determine the relevance of core and log data sources to address rock typing and permeability prediction uncertainties. The applied workflows also show how to break the autocorrelation of variables and maximize the usage of logs. This work demonstrates that the introduced data-driven methods are useful for rock typing determination and address several of the challenges related to core to log properties derivation.
更多查看译文
AI 理解论文
溯源树
样例
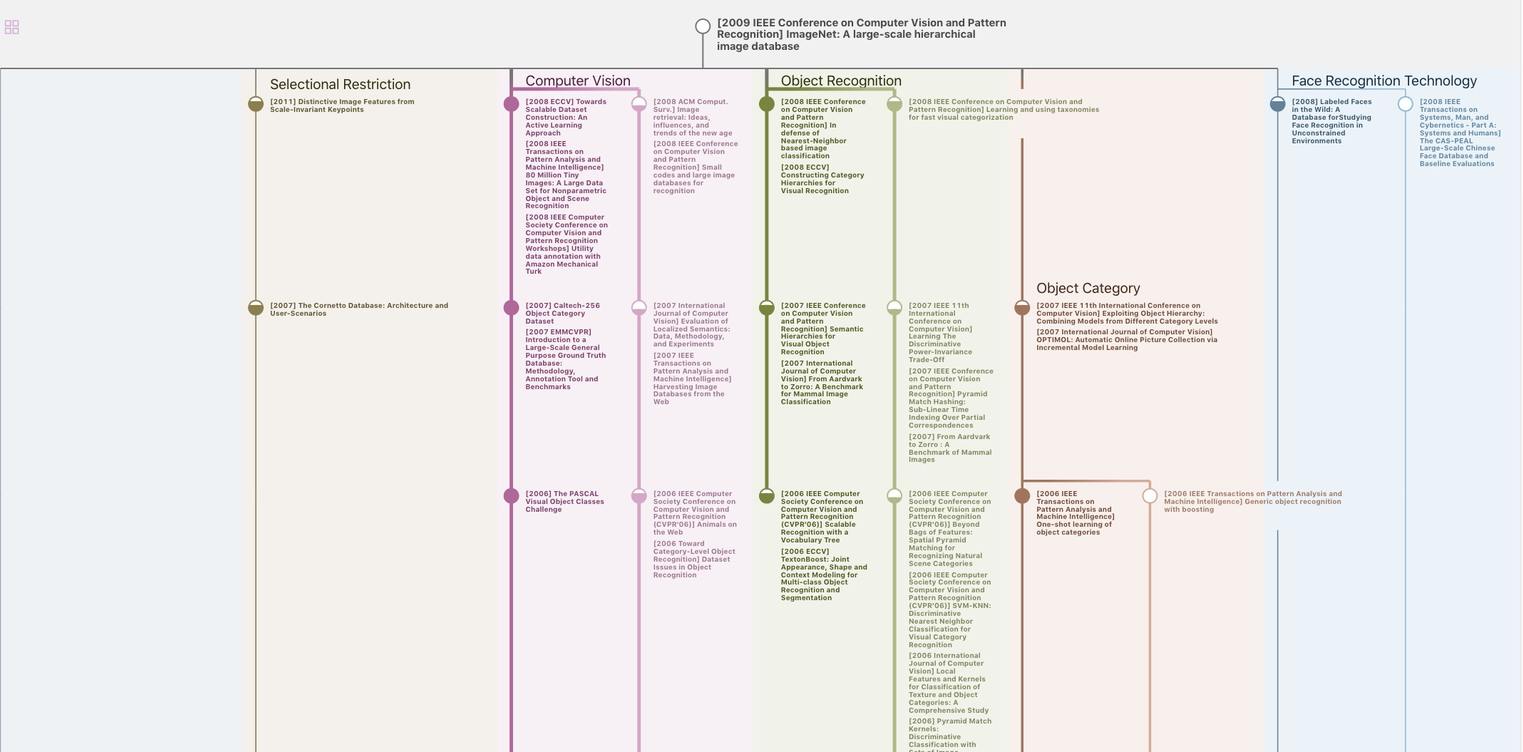
生成溯源树,研究论文发展脉络
Chat Paper
正在生成论文摘要