Estimating Large-Scale Tree Logit Models
SSRN Electronic Journal(2023)
摘要
We describe an efficient estimation method for large-scale tree logit models, using a novel change-of-variables transformation that allows us to express the negative log-likelihood as a strictly convex function in the leaf node parameters and a difference of strictly convex functions in the nonleaf node parameters. Exploiting this representation, we design a fast iterative method that computes a sequence of parameter estimates using simple closed-form updates. Our algorithm relies only on first-order information (function and gradients values), but unlike other first-order methods, it does not require any step size tuning or costly projection steps. The sequence of parameter estimates yields increasing likelihood values, and we establish sublinear convergence to a stationary point of the maximum likelihood problem. Numerical results on both synthetic and real data show that our algorithm outperforms state-of-the-art optimization methods, especially for largescale tree logit models with thousands of nodes.
更多查看译文
关键词
tree,models,large-scale
AI 理解论文
溯源树
样例
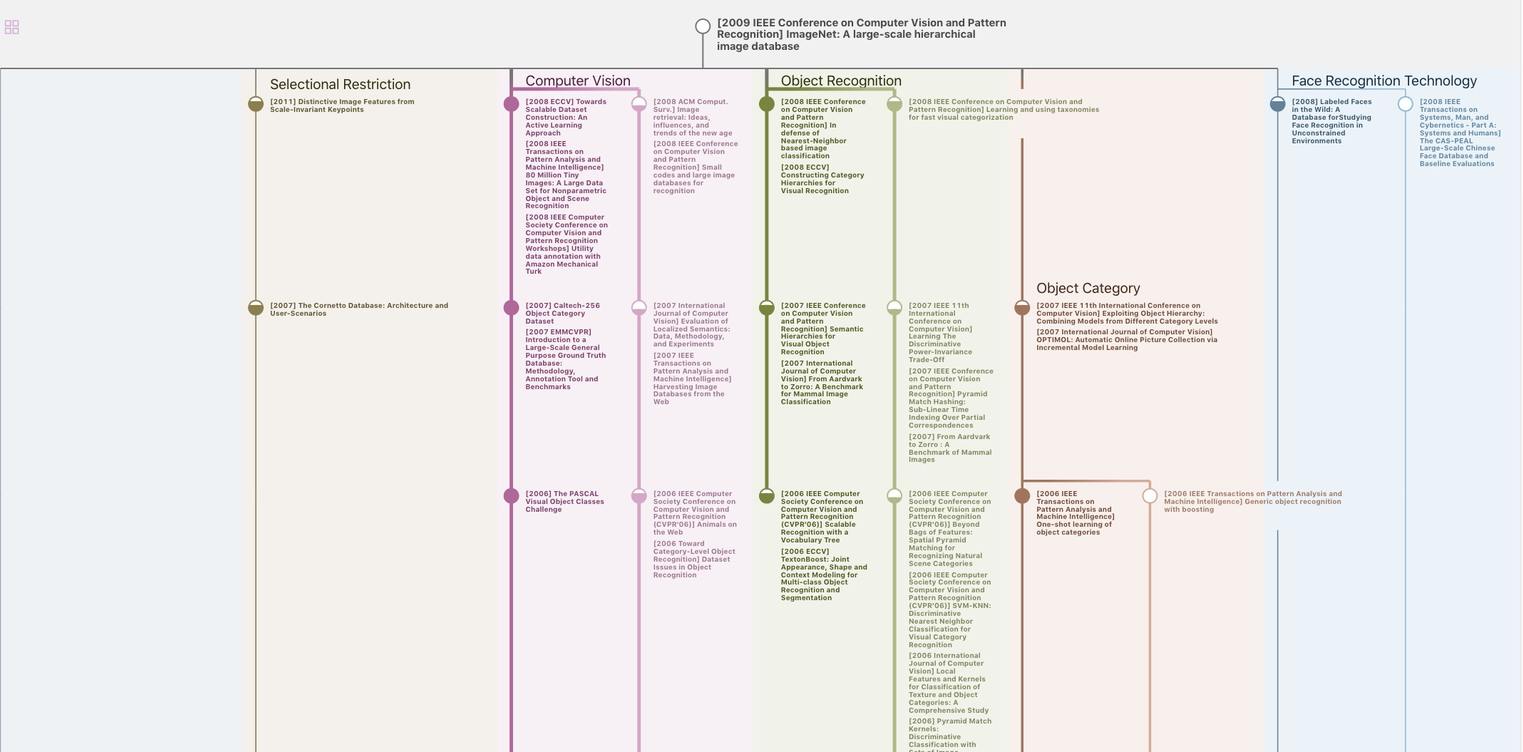
生成溯源树,研究论文发展脉络
Chat Paper
正在生成论文摘要