Segmentation-Based vs. Regression-Based Biomarker Estimation: A Case Study of Fetus Head Circumference Assessment from Ultrasound Images
JOURNAL OF IMAGING(2022)
摘要
The fetus head circumference (HC) is a key biometric to monitor fetus growth during pregnancy, which is estimated from ultrasound (US) images. The standard approach to automatically measure the HC is to use a segmentation network to segment the skull, and then estimate the head contour length from the segmentation map via ellipse fitting, usually after post-processing. In this application, segmentation is just an intermediate step to the estimation of a parameter of interest. Another possibility is to estimate directly the HC with a regression network. Even if this type of segmentation-free approaches have been boosted with deep learning, it is not yet clear how well direct approach can compare to segmentation approaches, which are expected to be still more accurate. This observation motivates the present study, where we propose a fair, quantitative comparison of segmentation-based and segmentation-free (i.e., regression) approaches to estimate how far regression-based approaches stand from segmentation approaches. We experiment various convolutional neural networks (CNN) architectures and backbones for both segmentation and regression models and provide estimation results on the HC18 dataset, as well agreement analysis, to support our findings. We also investigate memory usage and computational efficiency to compare both types of approaches. The experimental results demonstrate that even if segmentation-based approaches deliver the most accurate results, regression CNN approaches are actually learning to find prominent features, leading to promising yet improvable HC estimation results.
更多查看译文
关键词
head circumference, ultrasound imaging, segmentation CNN, regression CNN, biomarker estimation
AI 理解论文
溯源树
样例
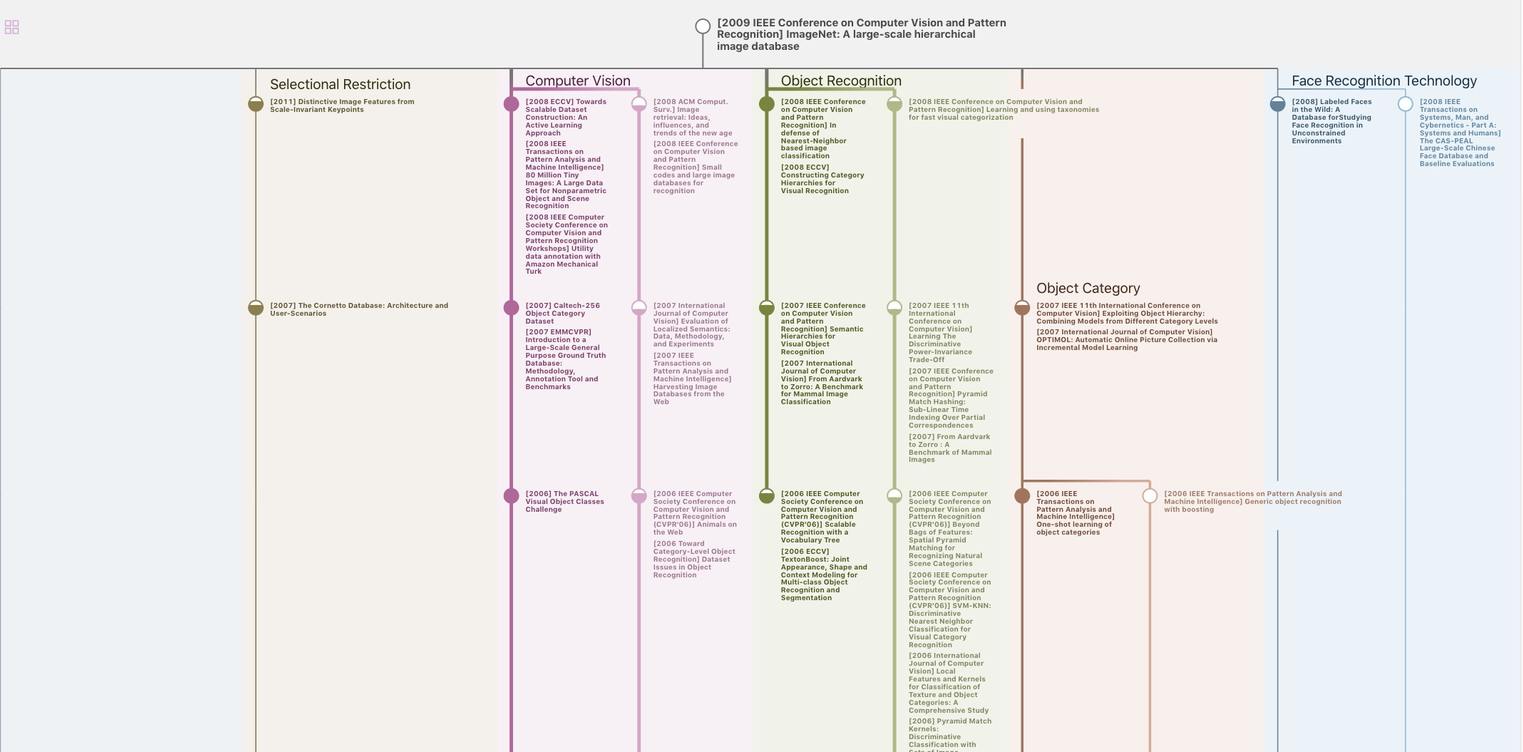
生成溯源树,研究论文发展脉络
Chat Paper
正在生成论文摘要