Using Graph Attention Network and Graph Convolutional Network to Explore Human CircRNA-Disease Associations Based on Multi-Source Data
FRONTIERS IN GENETICS(2022)
摘要
Cumulative research studies have verified that multiple circRNAs are closely associated with the pathogenic mechanism and cellular level. Exploring human circRNA-disease relationships is significant to decipher pathogenic mechanisms and provide treatment plans. At present, several computational models are designed to infer potential relationships between diseases and circRNAs. However, the majority of existing approaches could not effectively utilize the multisource data and achieve poor performance in sparse networks. In this study, we develop an advanced method, GATGCN, using graph attention network (GAT) and graph convolutional network (GCN) to detect potential circRNA-disease relationships. First, several sources of biomedical information are fused via the centered kernel alignment model (CKA), which calculates the corresponding weight of different kernels. Second, we adopt the graph attention network to learn latent representation of diseases and circRNAs. Third, the graph convolutional network is deployed to effectively extract features of associations by aggregating feature vectors of neighbors. Meanwhile, GATGCN achieves the prominent AUC of 0.951 under leave-one-out cross-validation and AUC of 0.932 under 5-fold cross-validation. Furthermore, case studies on lung cancer, diabetes retinopathy, and prostate cancer verify the reliability of GATGCN for detecting latent circRNA-disease pairs.
更多查看译文
关键词
circRNA-disease associations, deep learning, graph attention network, graph convolutional network, centered kernel alignment
AI 理解论文
溯源树
样例
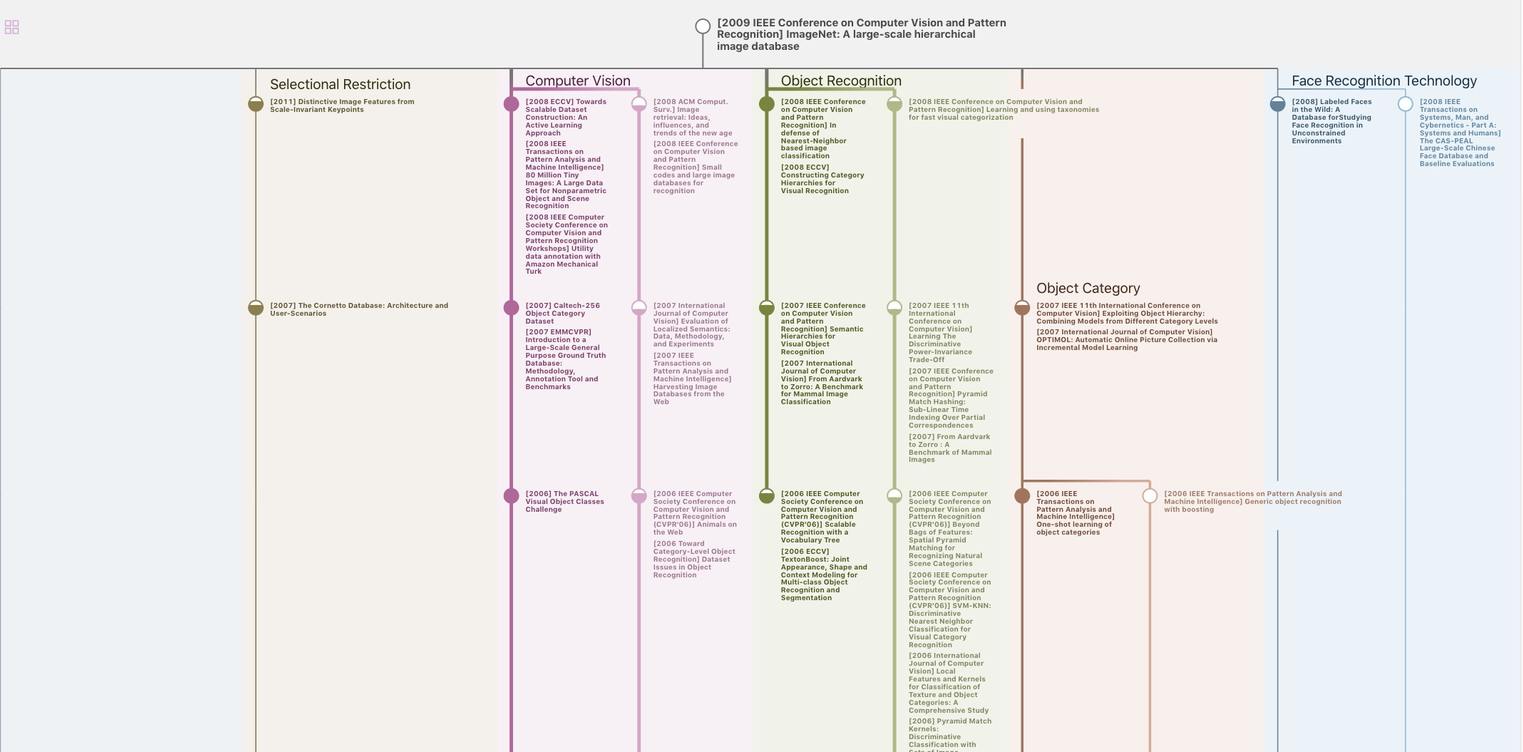
生成溯源树,研究论文发展脉络
Chat Paper
正在生成论文摘要