Modeling the bandwidth of perceptual experience using deep convolutional neural networks
crossref(2022)
摘要
When observers glance upon a natural scene, which aspects of that scene ultimately reach perceptual awareness? To answer this question, we showed observers images of scenes that had been altered in numerous ways in the periphery (e.g., scrambling, rotating, filtering, etc.) and measured how often these different alterations were noticed in an inattentional blindness paradigm. Then, we screened a wide range of deep convolutional neural network architectures and asked which layers and features best predict the rates at which observers noticed these alterations. We found that higher-level layers/features predicted how often observers noticed different alterations with extremely high accuracy (i.e., at the noise ceiling). This suggests that the extent to which observers noticed alterations to a scene depend on the extent to which higher-level features of a scene were preserved or changed. These findings suggest that perceptual awareness is limited by higher-level features of the human visual system.
更多查看译文
AI 理解论文
溯源树
样例
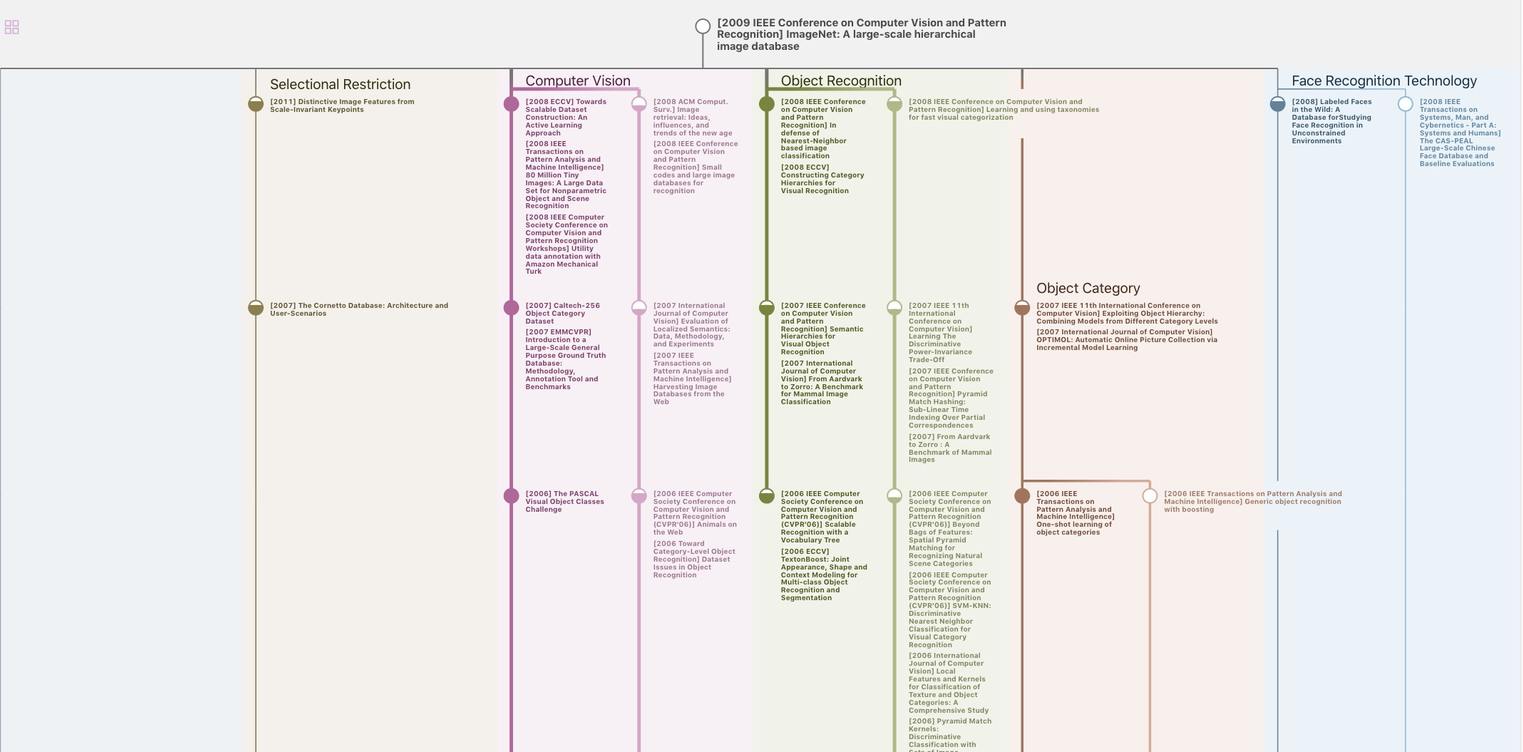
生成溯源树,研究论文发展脉络
Chat Paper
正在生成论文摘要