Global Convergence Rate of Proximal Incremental Aggregated Gradient Methods.
SIAM JOURNAL ON OPTIMIZATION(2018)
摘要
We focus on the problem of minimizing the sum of smooth component functions (where the sum is strongly convex) and a nonsmooth convex function, which arises in regularized empirical risk minimization in machine learning and distributed constrained optimization in wireless sensor networks and smart grids. We consider solving this problem using the proximal incremental aggregated gradient (PIAG) method, which at each iteration moves along an aggregated gradient (formed by incrementally updating gradients of component functions according to a deterministic order) and takes a proximal step with respect to the nonsmooth function. While the convergence properties of this method with randomized orders (in updating gradients of component functions) have been investigated, this paper, to the best of our knowledge, is the first study that establishes the convergence rate properties of the PIAG method for any deterministic order. In particular, we show that the PIAG algorithm is globally convergent with a linear rate provided that the step size is sufficiently small. We explicitly identify the rate of convergence and the corresponding step size to achieve this convergence rate. Our results improve upon the best known condition number and gradient delay bound dependence of the convergence rate of the incremental aggregated gradient methods used for minimizing a sum of smooth functions.
更多查看译文
关键词
convex optimization,nonsmooth optimization,proximal incremental aggregated gradient method
AI 理解论文
溯源树
样例
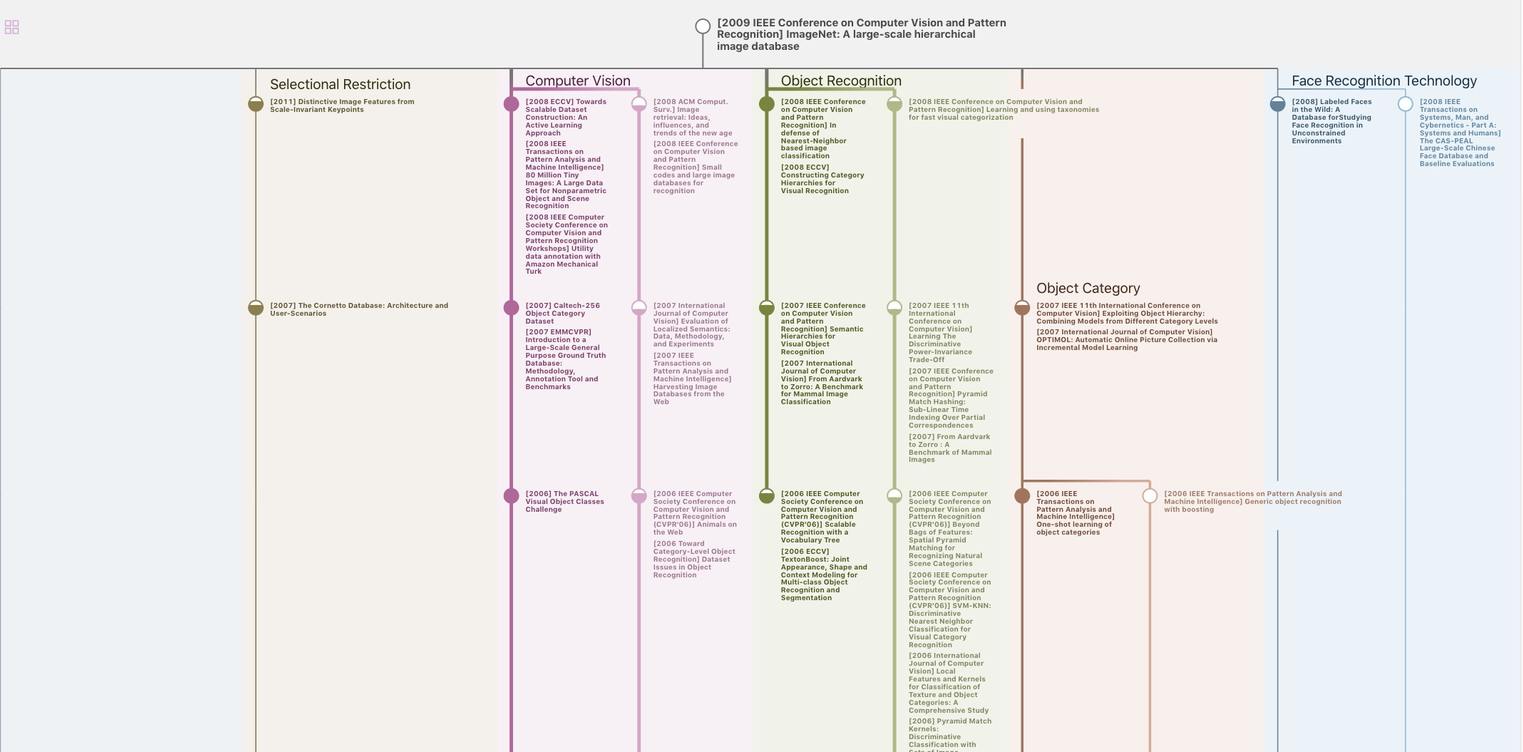
生成溯源树,研究论文发展脉络
Chat Paper
正在生成论文摘要