In Silico Strategy for Diagnosis of Chronic Kidney Disease
International Journal of Quantitative Structure-Property Relationships(2017)
摘要
Chronic kidney disease (CKD) is the third deadliest reason for mortality worldwide. An early detection of CKD would help to decelerate the loss of kidney function. Computational approaches provide opportunities to screen large populations for diagnosis of CKD. In this study, qualitative and quantitative models were developed to discriminate CKD and non-CKD subjects and to predict serum creatinine (SC) levels in populations using three simple clinical attributes as the predictors. The models were rigorously validated using stringent statistical coefficients, and applicability domains were also determined. The qualitative models yielded a binary classification accuracy >94% in test data, whereas, the quantitative models rendered a correlation (R2) of >0.94 in the test data. Values of all the statistical checks were within their respective thresholds, thus putting a high confidence in the proposed models. The proposed models can be used as the tools for screening large populations for their renal status.
更多查看译文
AI 理解论文
溯源树
样例
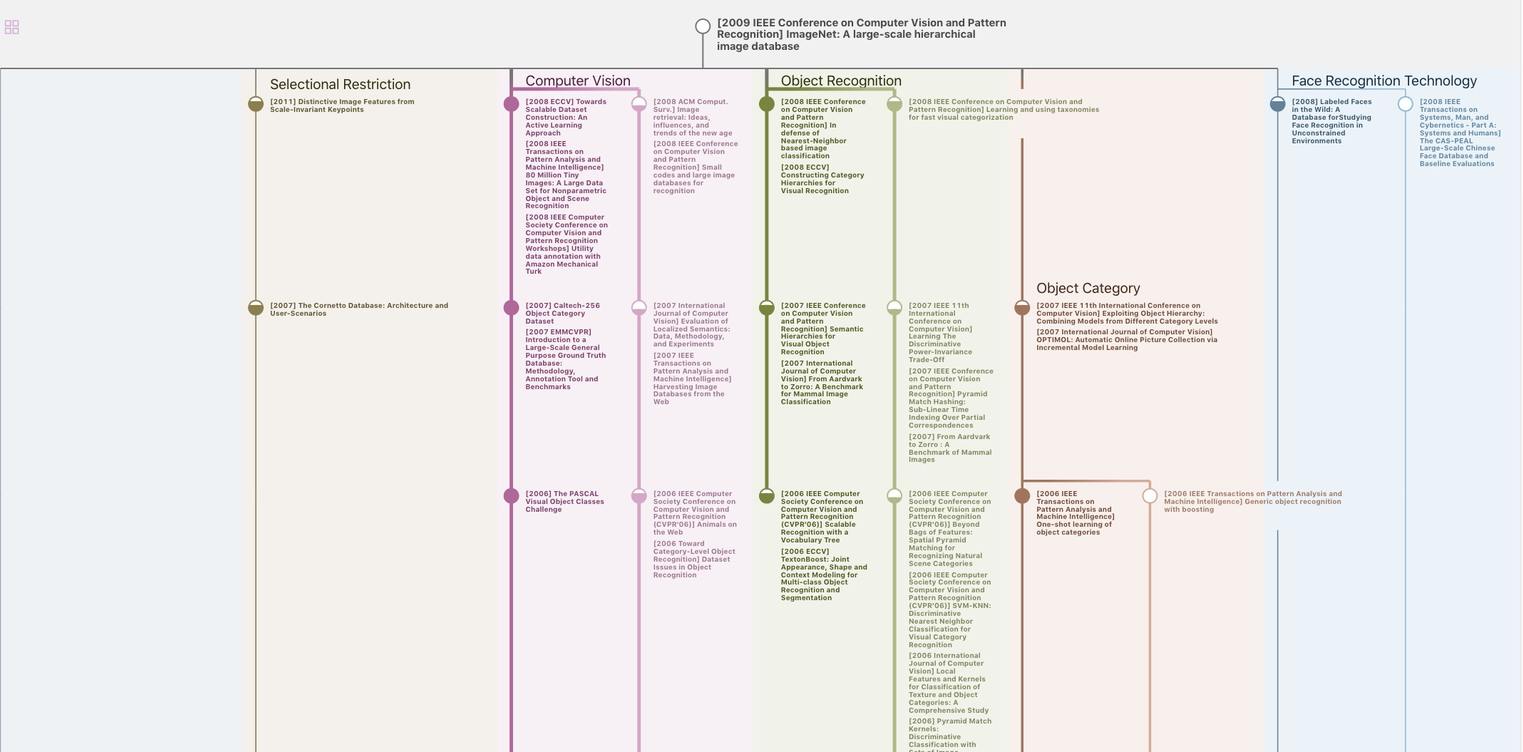
生成溯源树,研究论文发展脉络
Chat Paper
正在生成论文摘要