Learning stochastic dynamics with statistics-informed neural network
Journal of Computational Physics(2023)
摘要
We introduce a machine-learning framework named statistics-informed neural network (SINN) for learning stochastic dynamics from data. This new architecture was theoretically inspired by a universal approximation theorem for stochastic systems, which we introduce in this paper, and the projection-operator formalism for stochastic modeling. We devise mechanisms for training the neural network model to reproduce the correct statistical behavior of a target stochastic process. Numerical simulation results demonstrate that a well-trained SINN can reliably approximate both Markovian and non-Markovian stochastic dynamics. We demonstrate the applicability of SINN to coarse-graining problems and the modeling of transition dynamics. Furthermore, we show that the obtained reduced-order model can be trained on temporally coarse-grained data and hence is well suited for rare-event simulations.
更多查看译文
关键词
Scientific machine learning,Recurrent neural network,Reduced-order stochastic modeling,Rare events
AI 理解论文
溯源树
样例
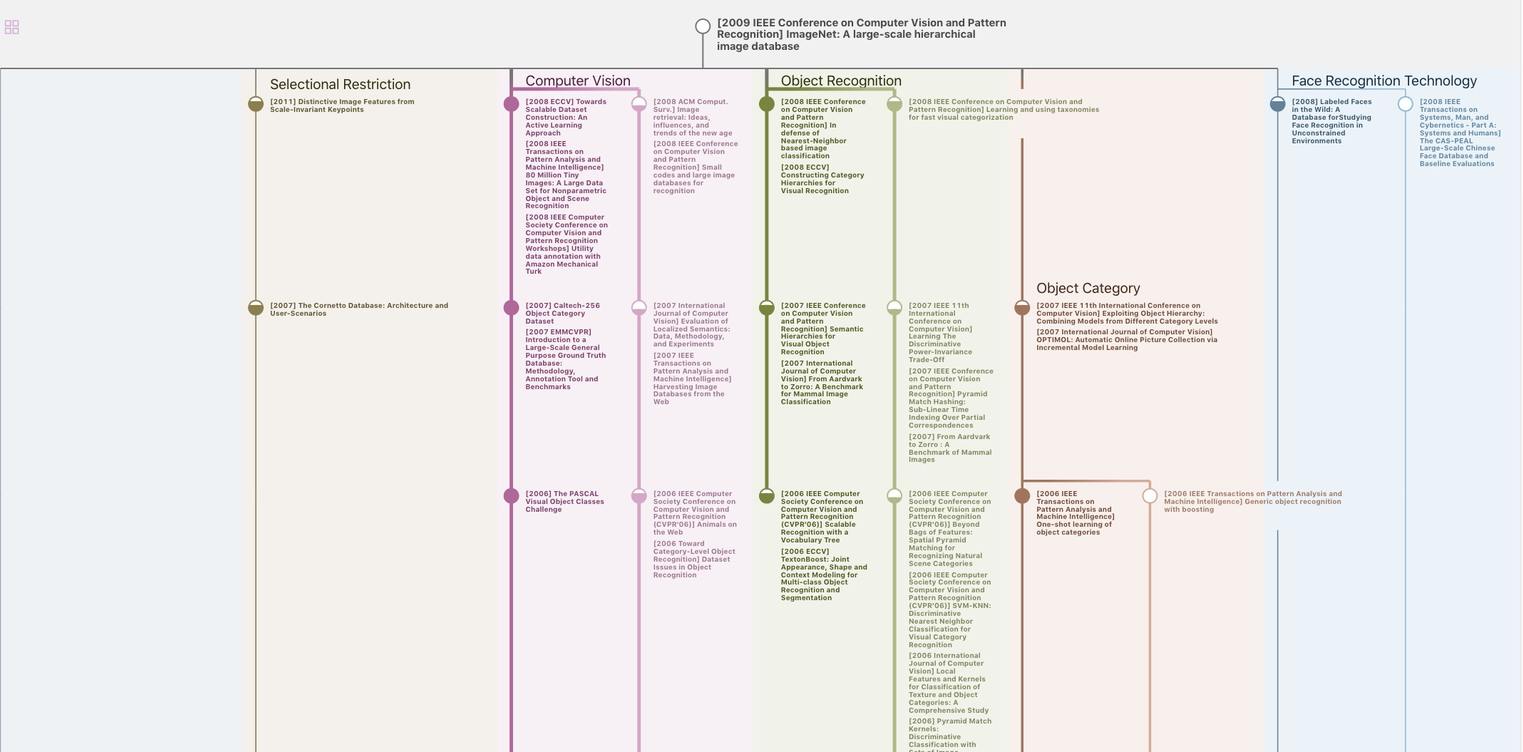
生成溯源树,研究论文发展脉络
Chat Paper
正在生成论文摘要