Modeling and Predicting Spatio-temporal Dynamics of PM$_{2.5}$ Concentrations Through Time-evolving Covariance Models
arxiv(2022)
摘要
Fine particulate matter (PM$_{2.5}$) has become a great concern worldwide due to its adverse health effects. PM$_{2.5}$ concentrations typically exhibit complex spatio-temporal variations. Both the mean and the spatio-temporal dependence evolve with time due to seasonality, which makes the statistical analysis of PM$_{2.5}$ challenging. In geostatistics, Gaussian process is a powerful tool for characterizing and predicting such spatio-temporal dynamics, for which the specification of a spatio-temporal covariance function is the key. While the extant literature offers a wide range of choices for flexible stationary spatio-temporal covariance models, the temporally evolving spatio-temporal dependence has received scant attention only. To this end, we propose a time-varying spatio-temporal covariance model for describing the time-evolving spatio-temporal dependence in PM$_{2.5}$ concentrations. For estimation, we develop a composite likelihood-based procedure to handle large spatio-temporal datasets.The proposed model is shown to outperform traditionally used models through simulation studies in terms of predictions. We apply our model to analyze the PM$_{2.5}$ data in the state of Oregon, US. Therein, we show that the spatial scale and smoothness exhibit periodicity. The proposed model is also shown to be beneficial over traditionally used models on this dataset for predictions.
更多查看译文
关键词
concentrations,spatio-temporal,time-evolving
AI 理解论文
溯源树
样例
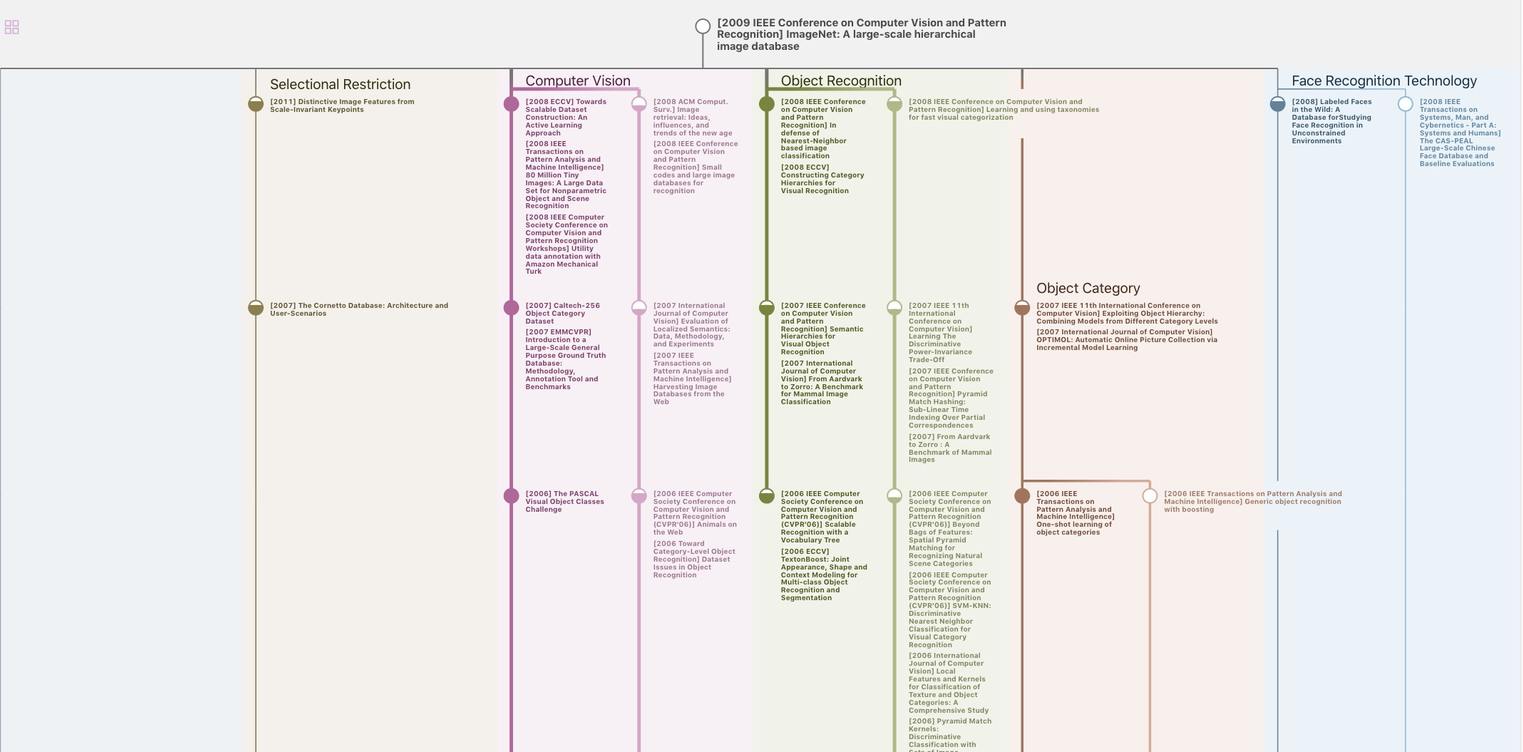
生成溯源树,研究论文发展脉络
Chat Paper
正在生成论文摘要