Explore-Bench: Data Sets, Metrics and Evaluations for Frontier-based and Deep-reinforcement-learning-based Autonomous Exploration
IEEE International Conference on Robotics and Automation(2022)
摘要
Autonomous exploration and mapping of unknown terrains employing single or multiple robots is an essential task in mobile robotics and has therefore been widely investigated. Nevertheless, given the lack of unified data sets, metrics, and platforms to evaluate the exploration approaches, we develop an autonomous robot exploration benchmark en-titled Explore-Bench. The benchmark involves various explo-ration scenarios and presents two types of quantitative metrics to evaluate exploration efficiency and multi-robot cooperation. Explore-Bench is extremely useful as, recently, deep rein-forcement learning (DRL) has been widely used for robot exploration tasks and achieved promising results. However, training DRL-based approaches requires large data sets, and additionally, current benchmarks rely on realistic simulators with a slow simulation speed, which is not appropriate for training exploration strategies. Hence, to support efficient DRL training and comprehensive evaluation, the suggested Explore-Bench designs a 3-level platform with a unified data flow and 12 × speed-up that includes a grid-based simulator for fast evaluation and efficient training, a realistic Gazebo simulator, and a remotely accessible robot testbed for high-accuracy tests in physical environments. The practicality of the proposed benchmark is highlighted with the application of one DRL-based and three frontier-based exploration approaches. Fur-thermore, we analyze the performance differences and provide some insights about the selection and design of exploration methods. Our benchmark is available at https://github.com/efc-robot/Explore-Bench.
更多查看译文
关键词
autonomous exploration,deep-reinforcement-learning-based deep-reinforcement-learning-based deep-reinforcement-learning-based,explore-bench,frontier-based
AI 理解论文
溯源树
样例
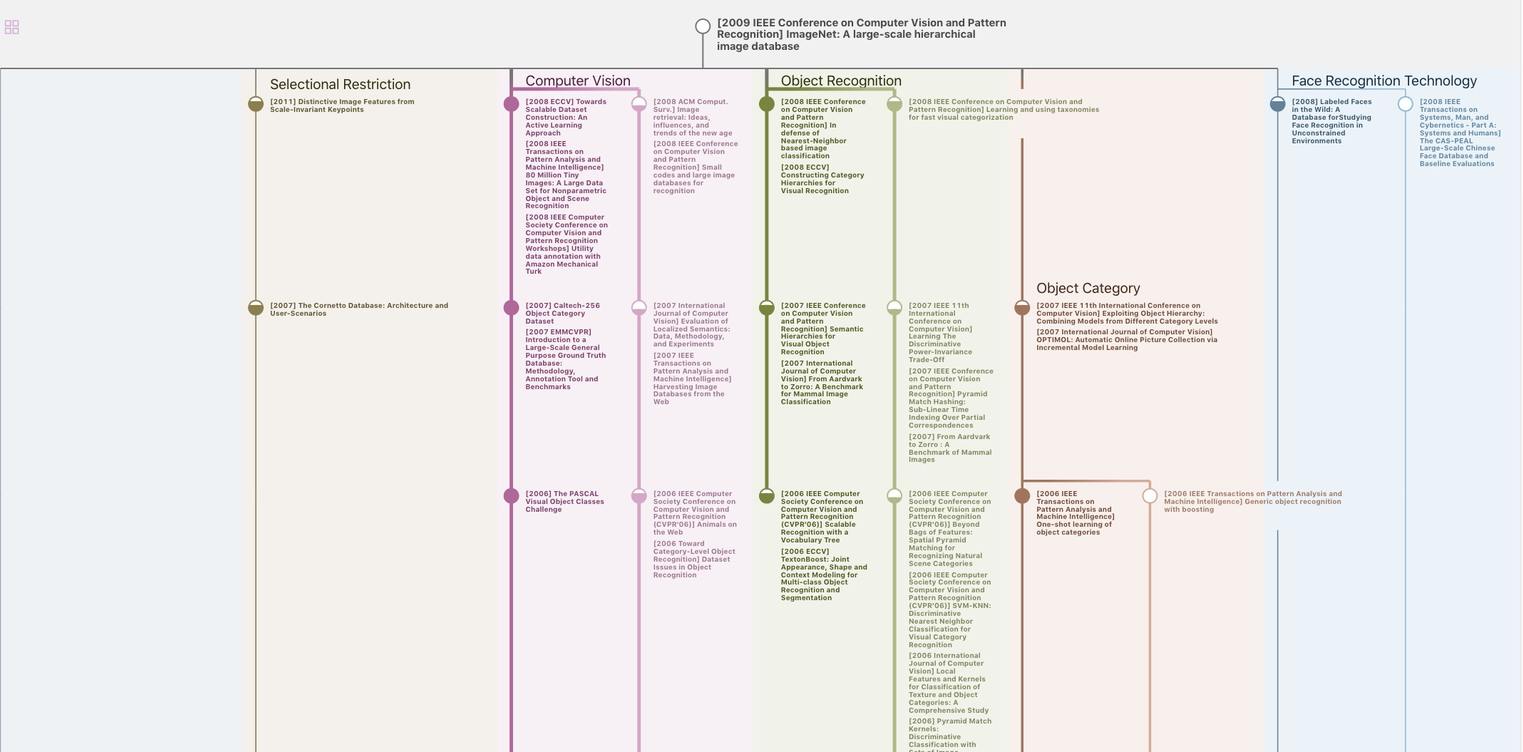
生成溯源树,研究论文发展脉络
Chat Paper
正在生成论文摘要