On the Posterior Distribution of a Random Process Conditioned on Empirical Frequencies of a Finite Path: the i.i.d and finite Markov chain case
arxiv(2022)
摘要
We obtain the posterior distribution of a random process conditioned on observing the empirical frequencies of a finite sample path. We find under a rather broad assumption on the "dependence structure" of the process, {\em c.f.} independence or Markovian, the posterior marginal distribution of the process at a given time index can be identified as certain empirical distribution computed from the observed empirical frequencies of the sample path. We show that in both cases of discrete-valued i.i.d. sequence and finite Markov chain, a certain "conditional symmetry" given by the observation of the empirical frequencies leads to the desired result on the posterior distribution. Results for both finite-time observations and its asymptotic infinite-time limit are connected via the idea of Gibbs conditioning. Finally, since our results demonstrate a central role of the empirical frequency in understanding the information content of data, we use the Large Deviations Principle (LDP) to construct a general notion of "data-driven entropy", from which one can apply a formalism from the recent study of statistical thermodynamics to data.
更多查看译文
关键词
finite markov chain
AI 理解论文
溯源树
样例
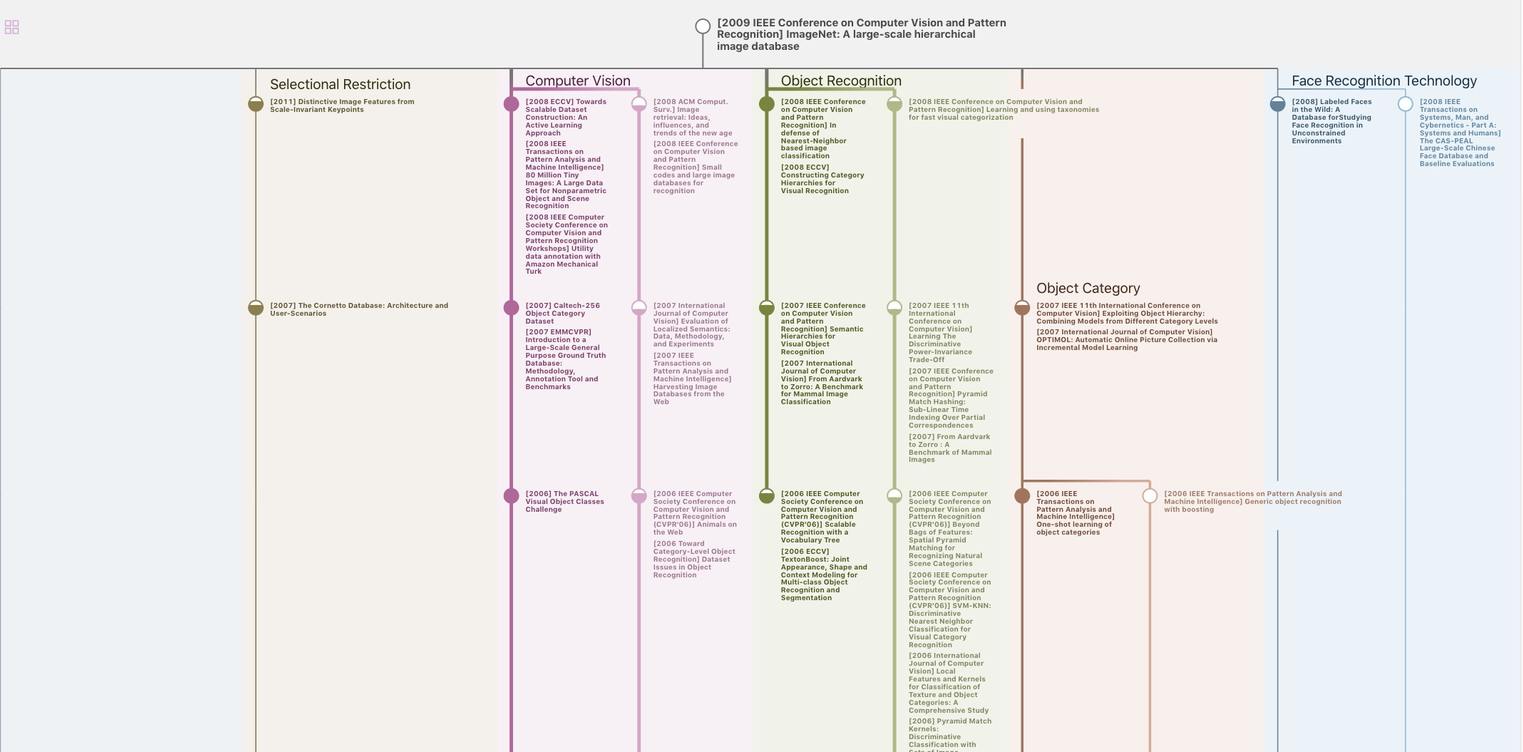
生成溯源树,研究论文发展脉络
Chat Paper
正在生成论文摘要