Augmentation based unsupervised domain adaptation
arxiv(2022)
摘要
The insertion of deep learning in medical image analysis had lead to the development of state-of-the art strategies in several applications such a disease classification, as well as abnormality detection and segmentation. However, even the most advanced methods require a huge and diverse amount of data to generalize. Because in realistic clinical scenarios, data acquisition and annotation is expensive, deep learning models trained on small and unrepresentative data tend to outperform when deployed in data that differs from the one used for training (e.g data from different scanners). In this work, we proposed a domain adaptation methodology to alleviate this problem in segmentation models. Our approach takes advantage of the properties of adversarial domain adaptation and consistency training to achieve more robust adaptation. Using two datasets with white matter hyperintensities (WMH) annotations, we demonstrated that the proposed method improves model generalization even in corner cases where individual strategies tend to fail.
更多查看译文
关键词
adaptation,augmentation,domain
AI 理解论文
溯源树
样例
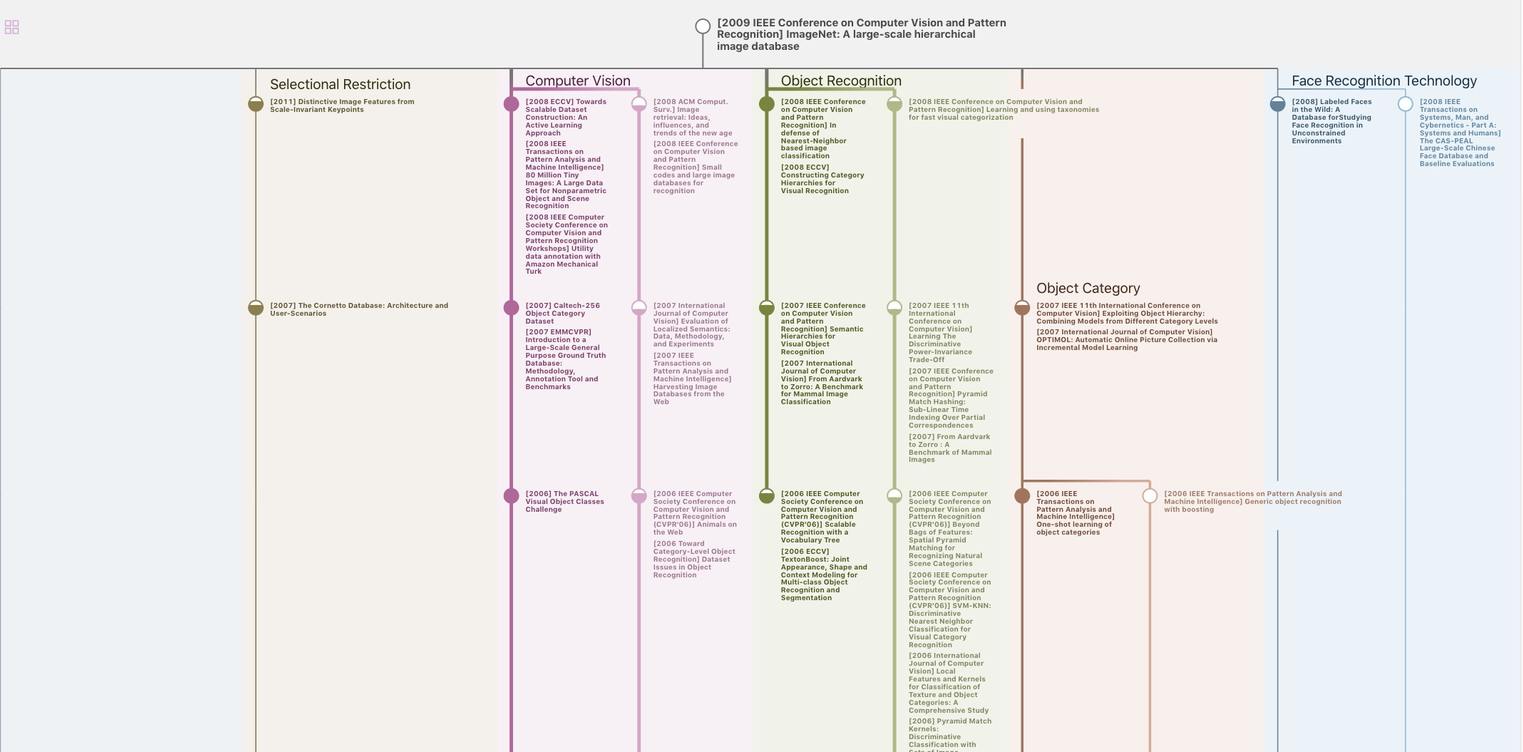
生成溯源树,研究论文发展脉络
Chat Paper
正在生成论文摘要