Extension of Dynamic Mode Decomposition for dynamic systems with incomplete information based on t-model of optimal prediction
arxiv(2022)
摘要
The Dynamic Mode Decomposition has proved to be a very efficient technique to study dynamic data. This is entirely a data-driven approach that extracts all necessary information from data snapshots which are commonly supposed to be sampled from measurement. The application of this approach becomes problematic if the available data is incomplete because some dimensions of smaller scale either missing or unmeasured. Such setting occurs very often in modeling complex dynamical systems such as power grids, in particular with reduced-order modeling. To take into account the effect of unresolved variables the optimal prediction approach based on the Mori-Zwanzig formalism can be applied to obtain the most expected prediction under existing uncertainties. This effectively leads to the development of a time-predictive model accounting for the impact of missing data. In the present paper we provide a detailed derivation of the considered method from the Liouville equation and finalize it with the optimization problem that defines the optimal transition operator corresponding to the observed data. In contrast to the existing approach, we consider a first-order approximation of the Mori-Zwanzig decomposition, state the corresponding optimization problem and solve it with the gradient-based optimization method. The gradient of the obtained objective function is computed precisely through the automatic differentiation technique. The numerical experiments illustrate that the considered approach gives practically the same dynamics as the exact Mori-Zwanzig decomposition, but is less computationally intensive.
更多查看译文
关键词
Dynamic mode decomposition,Mori-Zwanzig decomposition,Optimal prediction,t-model
AI 理解论文
溯源树
样例
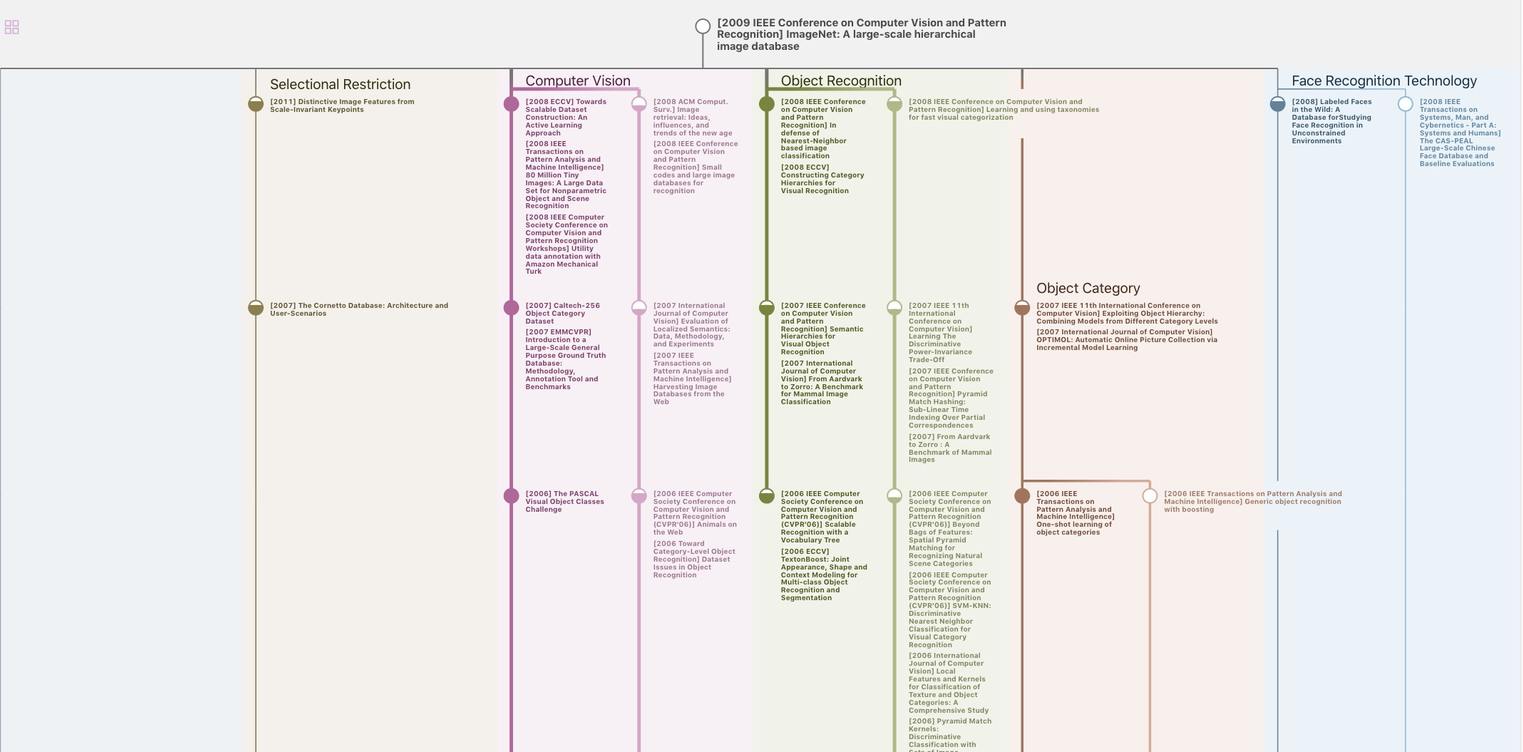
生成溯源树,研究论文发展脉络
Chat Paper
正在生成论文摘要