No-Regret Learning with Unbounded Losses: The Case of Logarithmic Pooling.
CoRR(2022)
摘要
For each of $T$ time steps, $m$ experts report probability distributions over $n$ outcomes; we wish to learn to aggregate these forecasts in a way that attains a no-regret guarantee. We focus on the fundamental and practical aggregation method known as logarithmic pooling -- a weighted average of log odds -- which is in a certain sense the optimal choice of pooling method if one is interested in minimizing log loss (as we take to be our loss function). We consider the problem of learning the best set of parameters (i.e. expert weights) in an online adversarial setting. We assume (by necessity) that the adversarial choices of outcomes and forecasts are consistent, in the sense that experts report calibrated forecasts. Our main result is an algorithm based on online mirror descent that learns expert weights in a way that attains $O(\sqrt{T} \log T)$ expected regret as compared with the best weights in hindsight.
更多查看译文
关键词
unbounded losses,learning,pooling,no-regret
AI 理解论文
溯源树
样例
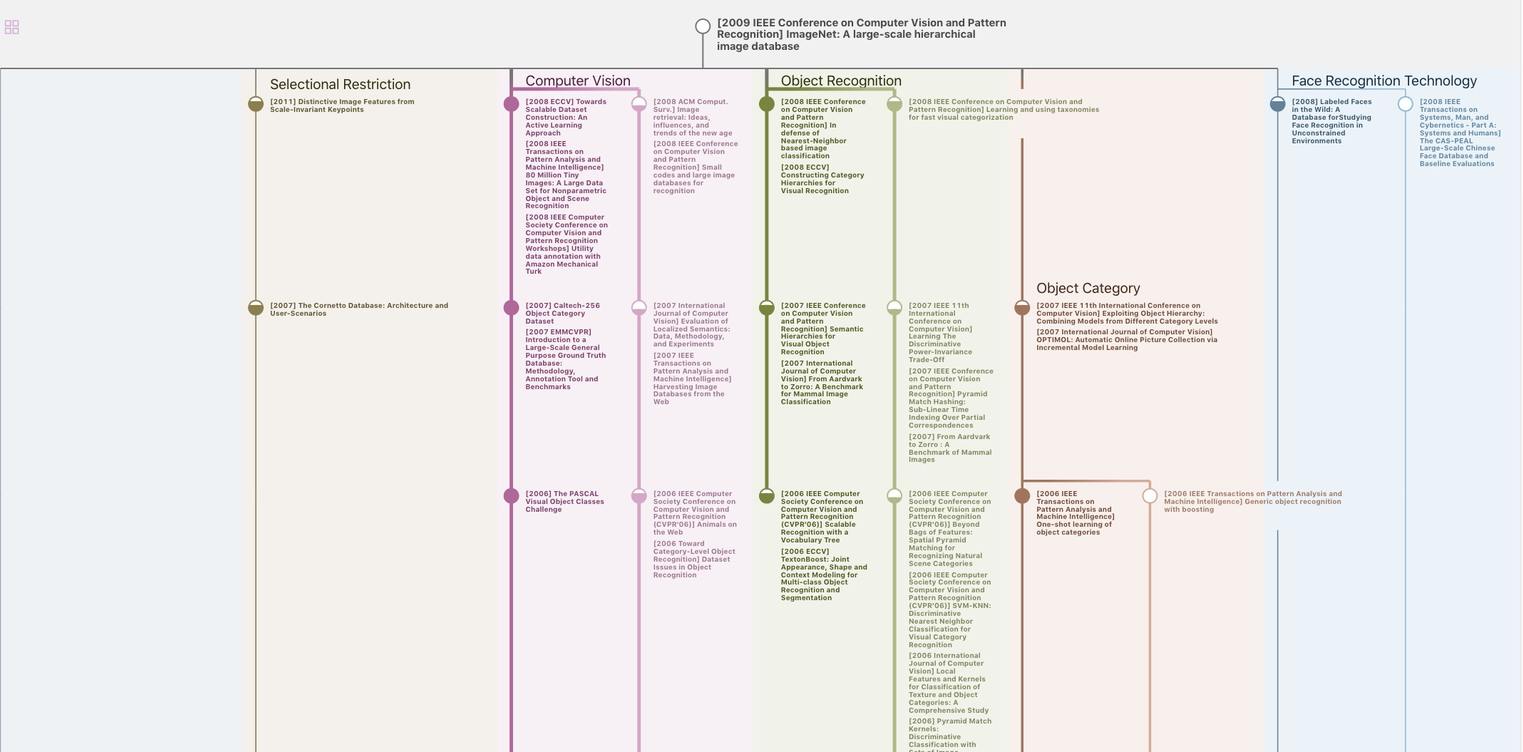
生成溯源树,研究论文发展脉络
Chat Paper
正在生成论文摘要