Multi-View Variational Autoencoder for Robust Classification against Irrelevant Data
2021 ASIA-PACIFIC SIGNAL AND INFORMATION PROCESSING ASSOCIATION ANNUAL SUMMIT AND CONFERENCE (APSIPA ASC)(2021)
摘要
Multi-view variational autoencoders (MVAEs) can extract latent variables with high discriminative power for classification by considering correlations among multi-view data, i.e., multiple kinds of data. However, if we input irrelevant data, i.e., multiple kinds of data that capture the different object, to MVAEs, discriminative power is reduced. To solve this problem, we propose an MVAE including a novel objective function. Our proposed MVAE reconstructs multi-view data without the negative effect of irrelevant data. Specifically, we derive an objective function that focuses on latent variables of relevant data, i.e., multiple kinds of data that capture the same object. Experimental results show that the proposed method improved the discriminative power of latent variables even if irrelevant data are input.
更多查看译文
关键词
objective function,MVAE,latent variables,multiview variational autoencoder,robust classification,high discriminative power
AI 理解论文
溯源树
样例
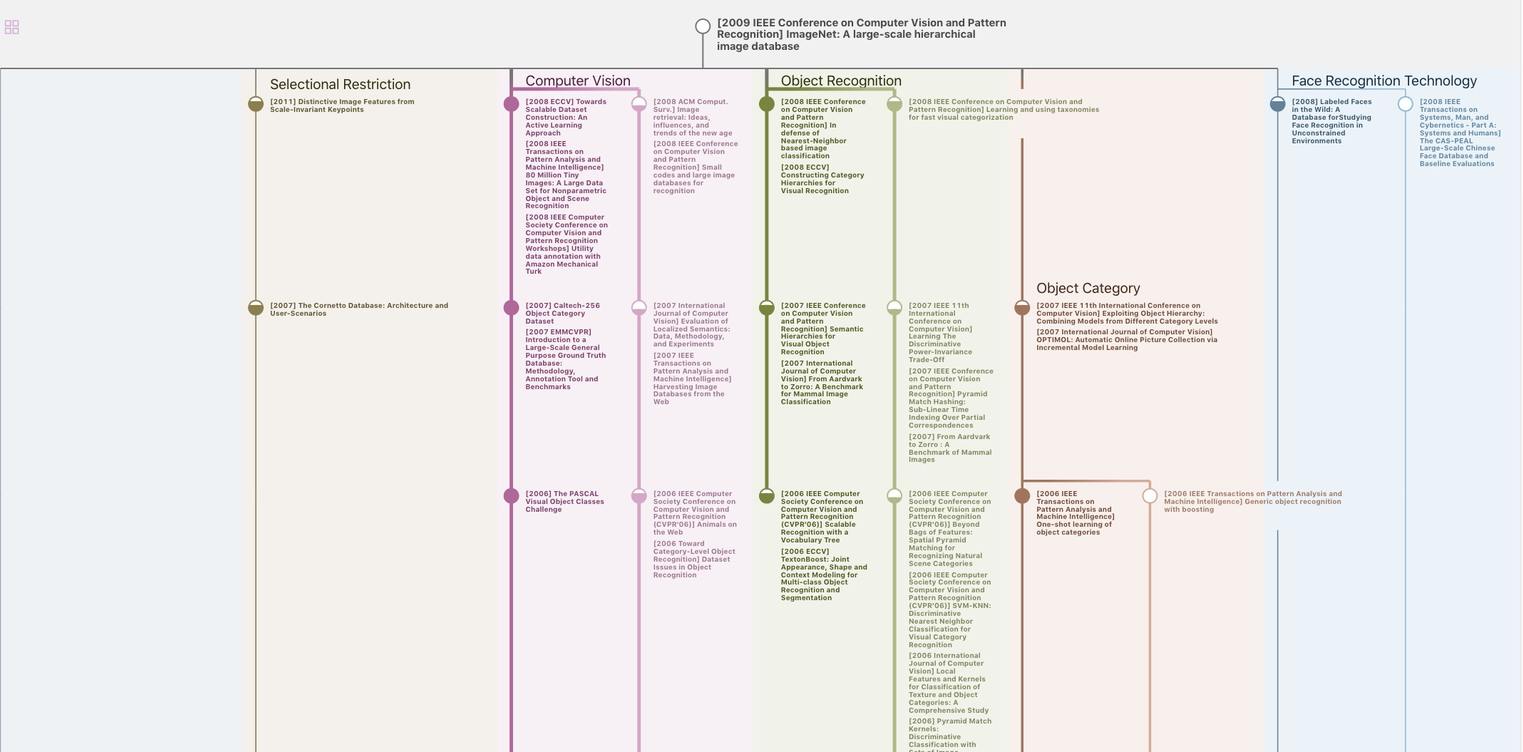
生成溯源树,研究论文发展脉络
Chat Paper
正在生成论文摘要