Augmentation-Agnostic Regularization for Unsupervised Contrastive Learning with Its Application to Speaker Verification
2021 ASIA-PACIFIC SIGNAL AND INFORMATION PROCESSING ASSOCIATION ANNUAL SUMMIT AND CONFERENCE (APSIPA ASC)(2021)
摘要
This paper presents a regularization method for unsupervised contrastive learning and its application to speaker verification. The proposed method, called Augmentation-Agnostic Regularization, enhances the training of speaker embeddings in an adversarial manner. Our main idea is to use an augmentation seed classifier, which learns to classify the randomization seeds used in data augmentation methods, and to train an embedding network with a regularization term to fool the classifier. This method prevents the characteristics of the augmentation procedure from remaining in the embed-dings, facilitating the extraction of speaker characteristics. In experiments, we demonstrate the effectiveness of the proposed regularization in two challenging data-deficient conditions, namely a small-sample training condition and a short-utterance testing condition, and show performance improvements over the conventional augmented adversarial training method. The unsupervised model trained with our method achieved compa-rable performance with the supervised x-vector baseline model.
更多查看译文
关键词
Contrastive Learning,Unsupervised Learning,Text-Independent Speaker Verification,Data Augmentation
AI 理解论文
溯源树
样例
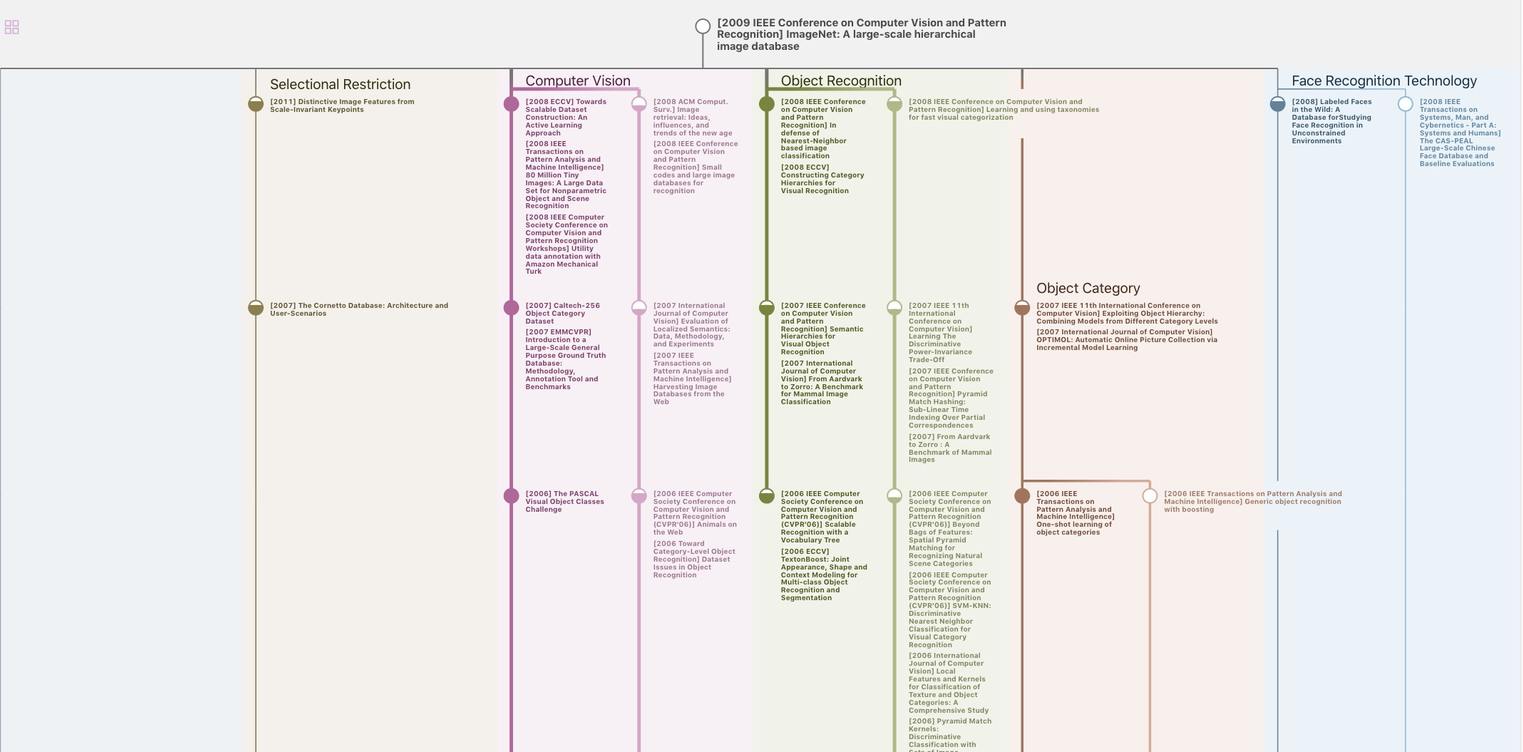
生成溯源树,研究论文发展脉络
Chat Paper
正在生成论文摘要