Multi-Resolution Convolutional Recurrent Networks
2021 ASIA-PACIFIC SIGNAL AND INFORMATION PROCESSING ASSOCIATION ANNUAL SUMMIT AND CONFERENCE (APSIPA ASC)(2021)
摘要
In sequential learning tasks, recurrent neural network (RNN) has been successfully developed for many years. RNN has achieved a great success in a variety of applications in presence of audio, video, speech and text data. On the other hand, temporal convolutional network (TCN) has recently drawn high attention in different works. TCN basically achieves comparable performance with RNN, but attractively TCN could work more efficient than RNN due to the parallel computation of one-dimensional convolution. A fundamental issue in sequential learning is to capture the temporal dependencies with different time scales. In this paper, we present a new sequential learning machine called the multi-resolution convolutional recurrent network (MR-CRN), which is a hybrid model of TCN encoder and RNN decoder. Utilizing the representation learned by TCN encoder in different layers with various temporal resolutions, RNN decoder can summarize the contextual information with different resolutions and time scales without modifying the original architecture. In the experiments on language modeling and action recognition, the merit of MR-CRN is illustrated for sequential learning and prediction in terms of latent representation, model perplexity and recognition accuracy.
更多查看译文
关键词
TCN encoder,temporal resolutions,RNN decoder,multiresolution convolutional recurrent network,sequential learning tasks,neural network,text data,temporal convolutional network,one-dimensional convolution,sequential learning machine
AI 理解论文
溯源树
样例
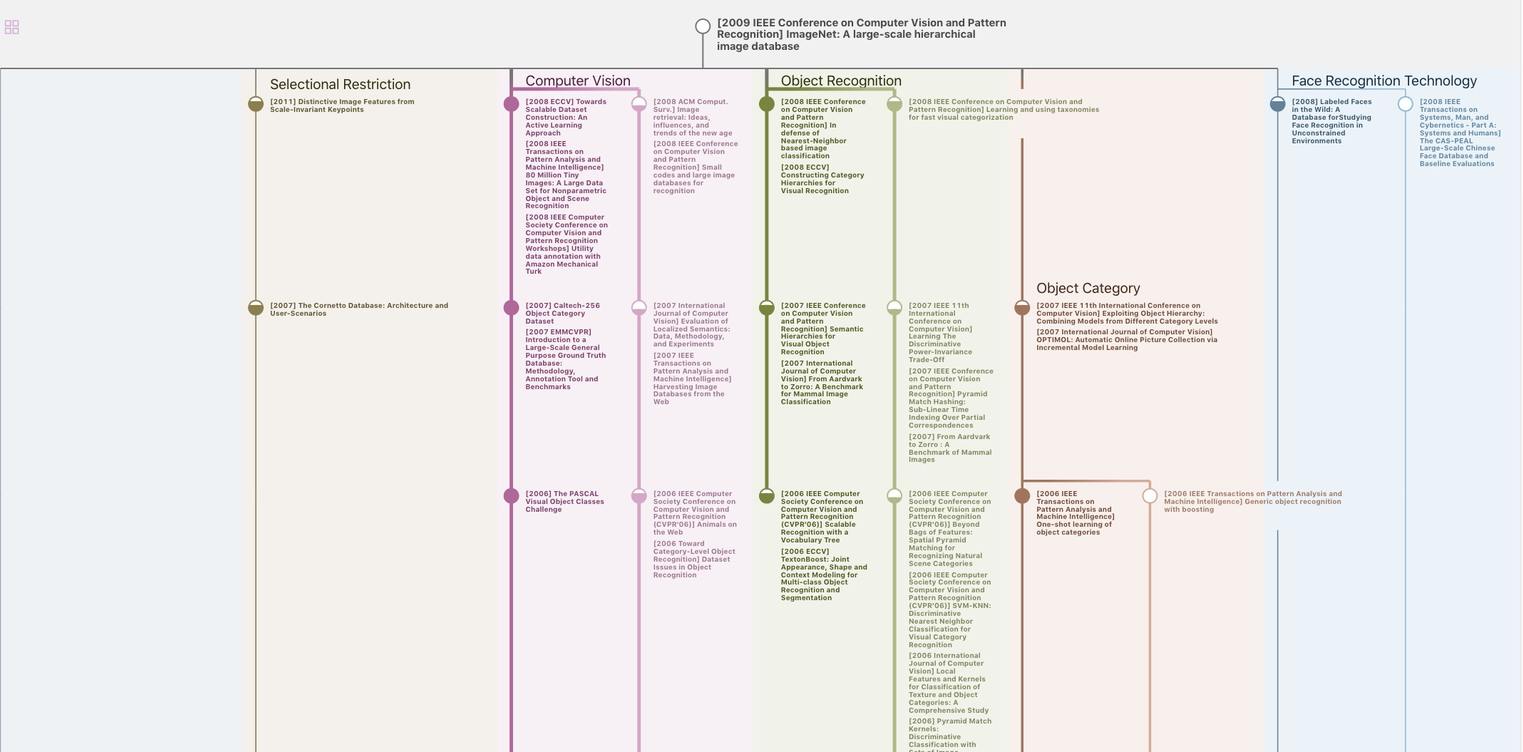
生成溯源树,研究论文发展脉络
Chat Paper
正在生成论文摘要