Generalized Classification of DNS over HTTPS Traffic with Deep Learning
2021 ASIA-PACIFIC SIGNAL AND INFORMATION PROCESSING ASSOCIATION ANNUAL SUMMIT AND CONFERENCE (APSIPA ASC)(2021)
摘要
Network anomaly detection has been a challenge for both industry and academia. The alarming situation of network attacks is a worrisome problem for many Internet services. Machine learning techniques are widely investigated to detect suspicious events from network traffic flow. In this paper, we investigate the DNS over HTTPS traffic classification. The majority of related works use a variety of features from datasets. However, some of the adopted features are specific to some networking environments, and those features make the trained models not generalized to other network environments. The generalization of a machine learning model is of critical importance, since it would affect the effectiveness when the model is applied to other network environments. We design an appropriate data processing pipeline to process the CIRA-CICDoHBrw-2020 time series dataset, including feature selection and data imbalance handling, in order to facilitate the generalization of deep learning models. We develop truly generalized deep learning models, including the LSTM model and the BiLSTM model, to classify DoH traffic with high accuracy and low latency. While both models achieve good performance, the BiLSTM model performs better than the LSTM model does for both the accuracy and the computation time.
更多查看译文
关键词
Deep learning, network attack, anomaly detection, machine learning, neural network, DNS over Hypertext Transfer Protocol Secure, DoH
AI 理解论文
溯源树
样例
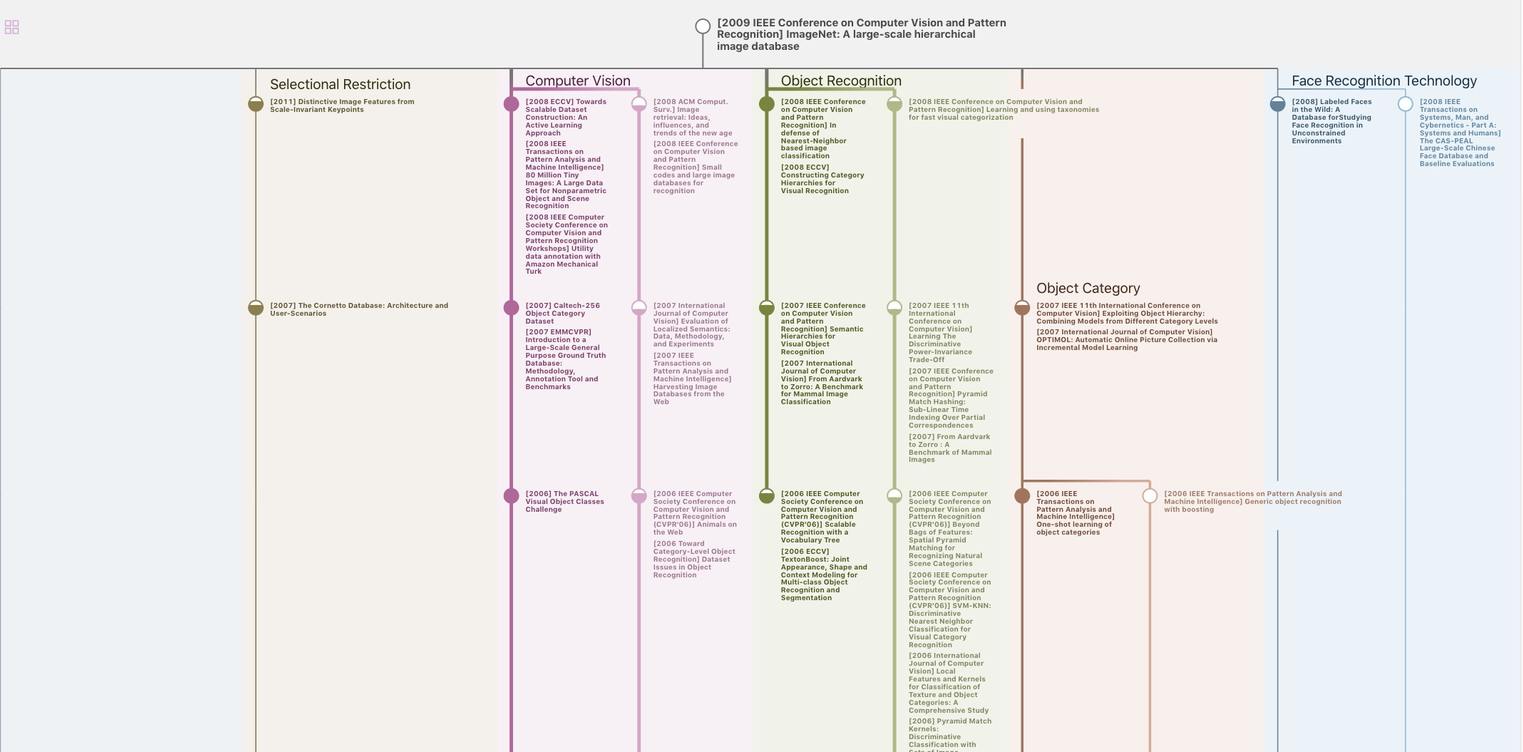
生成溯源树,研究论文发展脉络
Chat Paper
正在生成论文摘要