TOWARDS USING HETEROGENEOUS RELATION GRAPHS FOR END-TO-END TTS
2021 IEEE AUTOMATIC SPEECH RECOGNITION AND UNDERSTANDING WORKSHOP (ASRU)(2021)
摘要
Neural models for end-to-end text-to-speech (TTS) synthesis are increasingly outperforming traditional approaches in statistical parametric speech synthesis. Speech generation in these neural models predominantly relies on using free-form text as the input modality. However, the earlier statistical parametric models were built on encoded phonetic and syntactic features. In this work, we explore the possibility of explicitly feeding deterministic linguistic structure to a neural TTS system in the form of Heterogeneous Relational Graphs (HRGs), an expressive formalism capable of representing phonetic and syntactic information. Specifically, we use Graph Convolutional Networks to learn structurally informed continuous representations of the HRGs, which can be seamlessly passed to the encoders of popular neural TTS models like TransformerTTS or Tacotron. Furthermore, our simple HRG based text-to-speech synthesis leverages the syntactic bias in HRGs as demonstrated by improvements in automated metrics and human evaluation on i) the single speaker dataset LJSpeech; ii) the multi-speaker dataset Arctic; and iii) out-of-domain test sets from the Blizzard challenge.
更多查看译文
关键词
text-to-speech, end-to-end neural TTS, Graph Convolutional Networks, Heterogeneous Relation Graphs
AI 理解论文
溯源树
样例
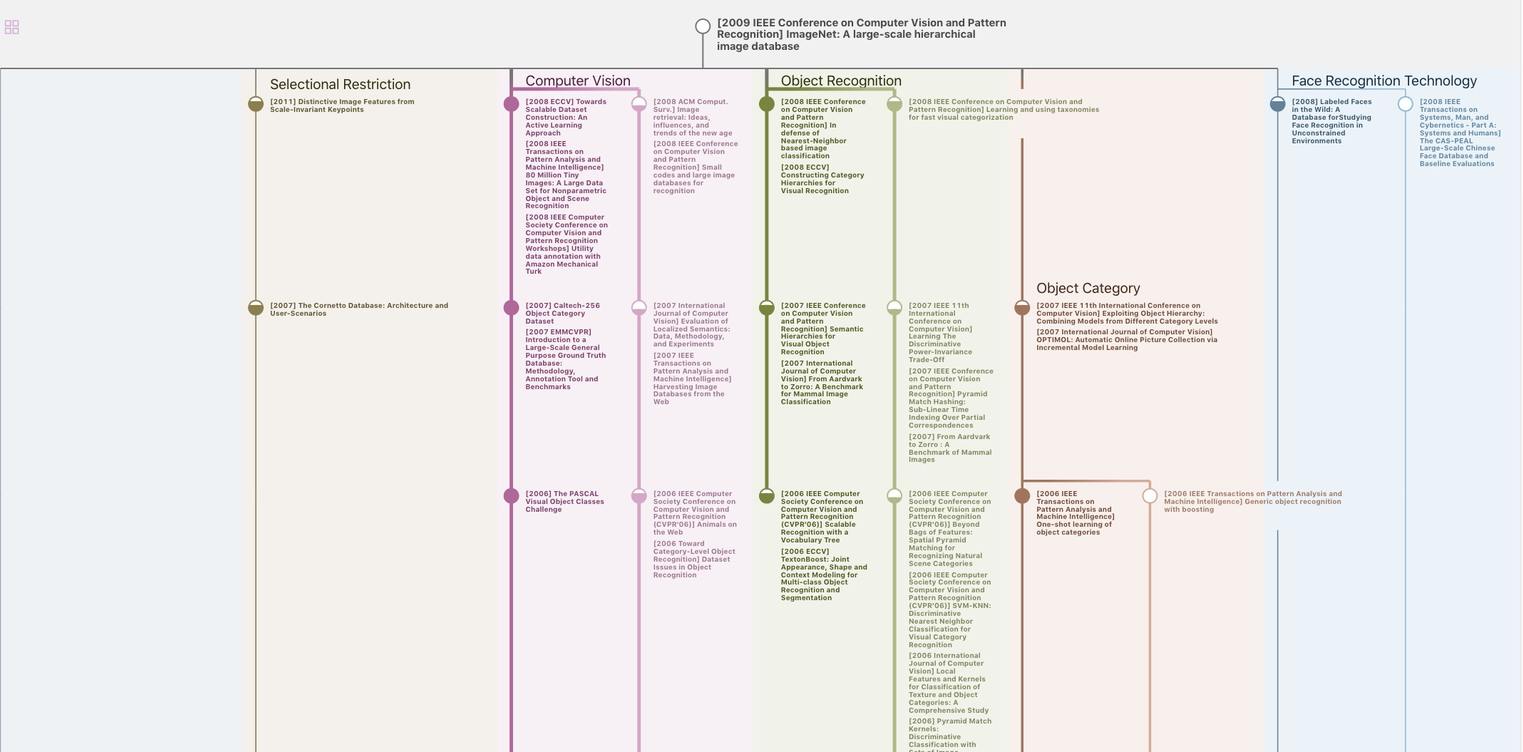
生成溯源树,研究论文发展脉络
Chat Paper
正在生成论文摘要