An ASR N-Best Transcript Neural Ranking Model for Spoken Content Retrieval
2021 IEEE AUTOMATIC SPEECH RECOGNITION AND UNDERSTANDING WORKSHOP (ASRU)(2021)
摘要
Spoken Content Retrieval (SCR) using ASR transcripts is in-creasingly important for multimedia content archives. How-ever, SCR is often impacted by ASR errors. In recent years novel neural ranking methods for information retrieval (IR) have achieved improved search effectiveness over established methods. In this study, we examine neural ranking methods in SCR. Specifically we introduce two new neural ranking methods designed for use with errorful ASR transcripts. In the first, we train a neural ranking model using both man-ual transcripts and ASR transcripts or manual transcripts and artificially created ASR transcripts. In the second, we use an ASR N-best extension of two existing neural ranking methods: Deep Relevance Matching Model (DRMM) and Position-Aware Convolutional Recurrent Matching (PACRR). We report two sets of SCR experiments which evaluate our neural ranking methods. In the first, we use ASR transcripts to retrieve documents from an archive of spoken instruction videos. In the second, we examine a known-item search on a collection of user-generated spoken videos. We find that training the neural ranking model on both ASR and man-ual transcripts improves Mean Reciprocal Rank (MRR). The N-best extension of PACRR is particularly effective in both experiments in comparison to a standard BM25 IR model.
更多查看译文
关键词
Spoken content retrieval, Neural ranking, Information Retrieval, ASR N-best
AI 理解论文
溯源树
样例
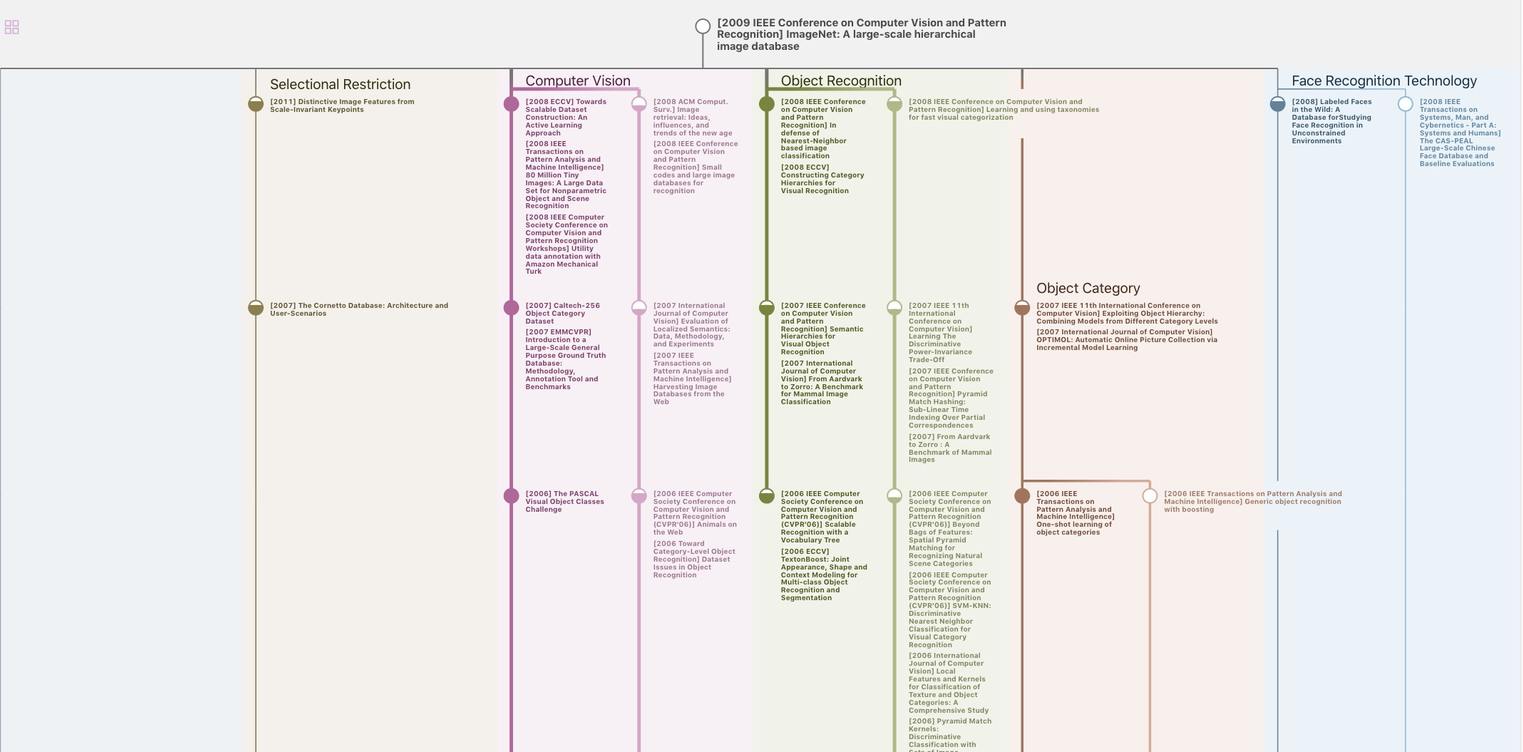
生成溯源树,研究论文发展脉络
Chat Paper
正在生成论文摘要