USING SELF ATTENTION DNNS TO DISCOVER PHONEMIC FEATURES FOR AUDIO DEEP FAKE DETECTION
2021 IEEE AUTOMATIC SPEECH RECOGNITION AND UNDERSTANDING WORKSHOP (ASRU)(2021)
摘要
With the advancement in natural-sounding speech production models, it is becoming important to develop models that can detect spoofed audios. Synthesized speech models do not explicitly account for all factors affecting speech production, such as the shape, size and structure of a speaker's vocal tract. In this paper, we hypothesize that due to practical limitations of audio corpora (including size, distribution, and balance of variables like gender, age, and accents), there exist certain phonemes that synthesized models are not able to replicate as well as the human articulation system and such phonemes differ in their spectral characteristics from bonafide speech. To discover such phonemes and quantify their effectiveness in distinguishing between spoofed and bonafide speech, we use a deep learning model with self-attention, and analyze the attention weights of the trained model. We use the ASVSpoof2019 dataset for our analysis and find that the attention mechanism picks most on fricatives: /S/,/SH/, nasals: /M/,/N/, vowels: /Y/, and stops: /D/. Furthermore, we obtain 7.54% EER on train and 11.98% on dev data when using only the top-16 most attended phonemes from input audio, better than when any other phoneme classes are used.
更多查看译文
关键词
spoof, bonafide, countermeasure, attention, phonemes, deep neural network, senet, explainable, fair, small datasets, forensics, deepfake
AI 理解论文
溯源树
样例
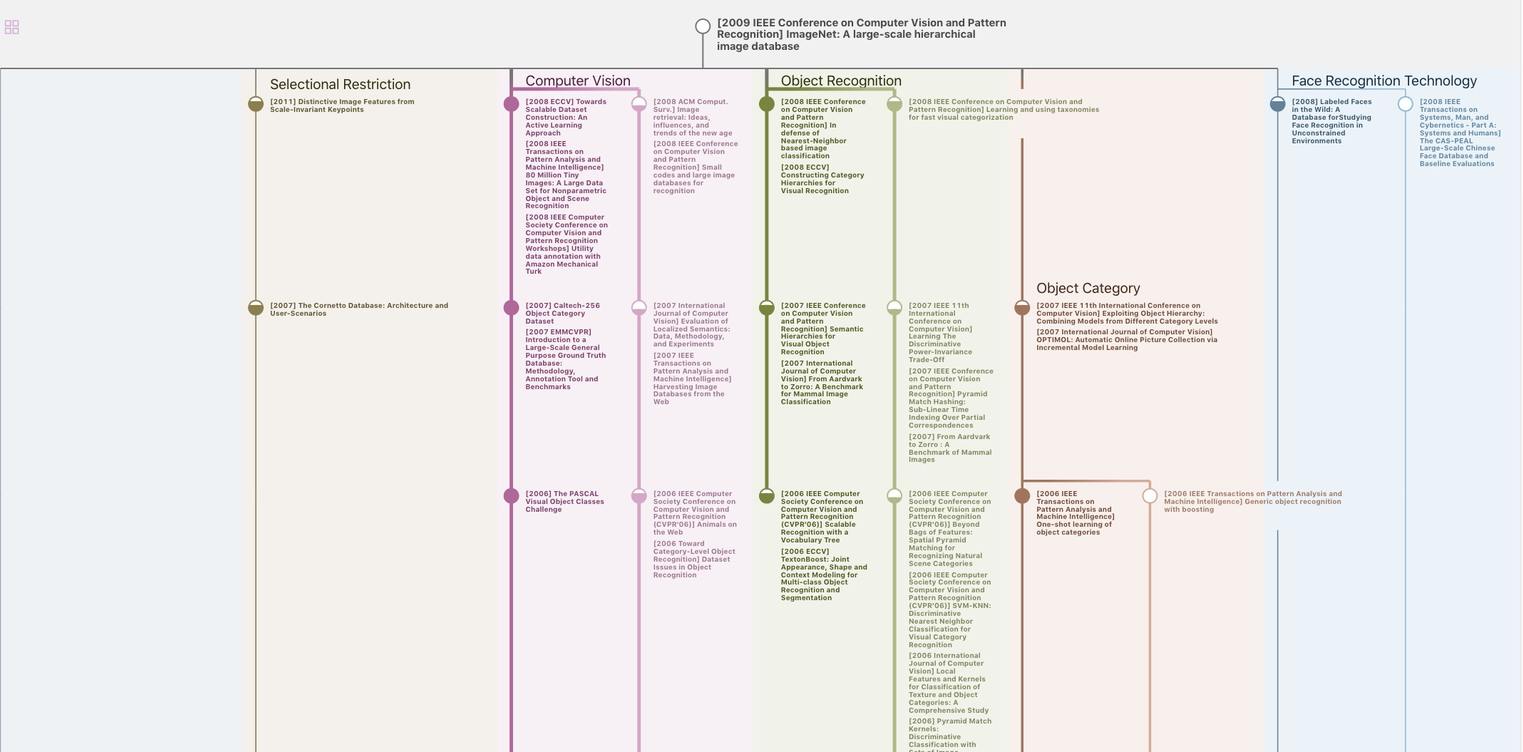
生成溯源树,研究论文发展脉络
Chat Paper
正在生成论文摘要