Remaining Useful Life Prediction of Lithium Battery with Enhanced Bi-LSTM Network.
CAA SAFEPROCESS(2021)
摘要
Aiming at the problem that the existing models only consider the effect of one internal state on the degradation performance of the lithium batteries, three models are simultaneously established to predict the trajectory of the three time-varying internal states (capacity, resistance, temperature), and the capacity is updated real-time based on the prediction of the internal resistance and temperature. At the same time, a new Mish activation function is introduced into the Bi-directional Long-Short Term Memory (Bi-LSTM) network and the fully connected network, which enable the models to extract higher quality features with a smooth gradient flow. And the features are utilized in the modeling analysis of Remaining Useful Life (RUL) prediction. Finally, the uncertainty of the RUL prediction results of lithium battery is analyzed based on the Monte Carlo-Dropout (MC-Dropout) technology. The effectiveness of our proposed model is verified through the lithium battery set of professor Kristen's research group.
更多查看译文
关键词
Remaining Useful Life prediction,three time-varying internal states,enhanced Bi-LSTM network,Monte Carlo-Dropout technology,uncertainty analysis
AI 理解论文
溯源树
样例
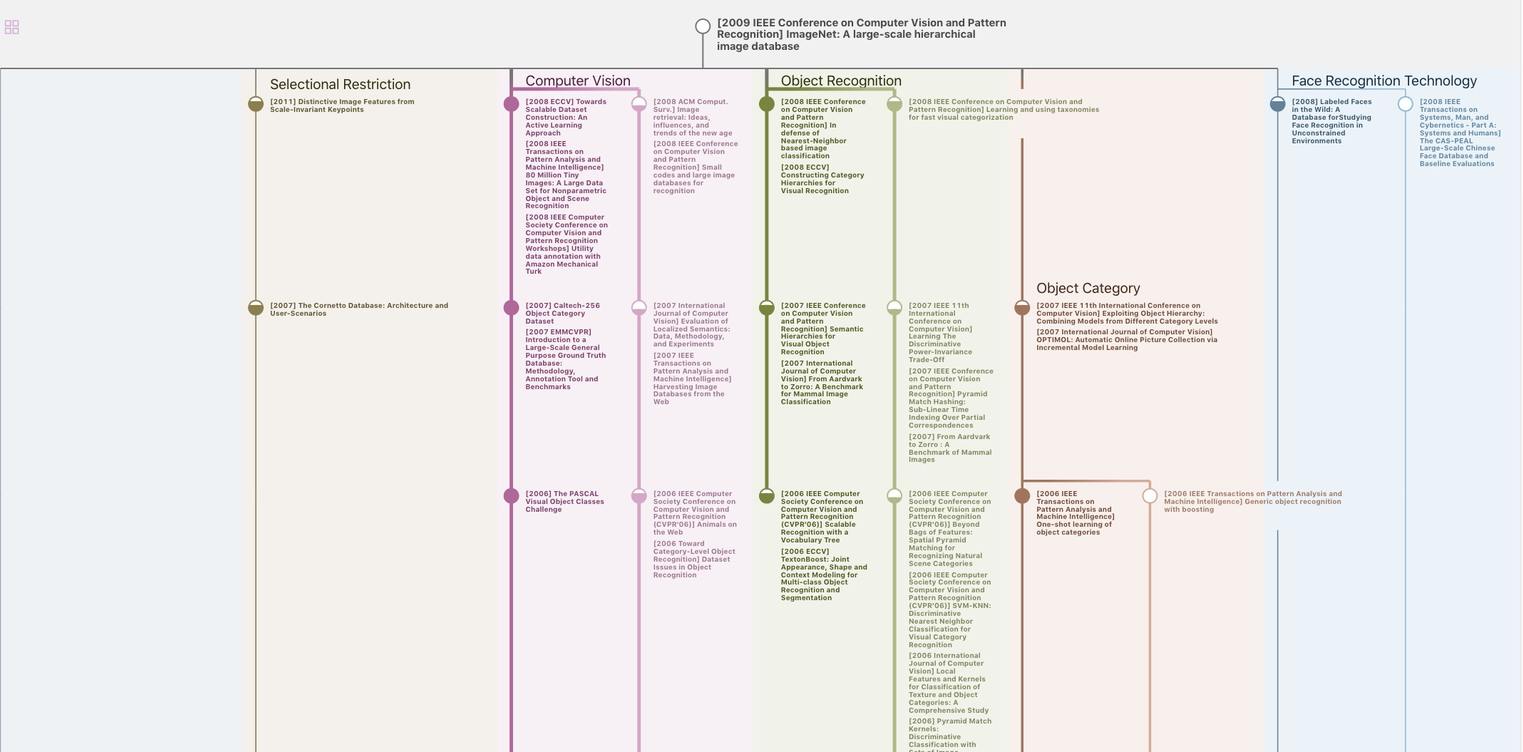
生成溯源树,研究论文发展脉络
Chat Paper
正在生成论文摘要