Multi-modal Classification Using Domain Adaptation for Automated Defect Detection Based on the Hammering Test
2022 IEEE/SICE INTERNATIONAL SYMPOSIUM ON SYSTEM INTEGRATION (SII 2022)(2022)
摘要
Inspecting concrete structures such as tunnels is very important to keep them safe and durable. Due to the shortage of human inspectors, automated system for inspection is highly required. Hammering test is one of the popular inspection methods, and previous studies proposed automated systems for hammering test. Most works based on machine learning models to train a classifier to recognize hammering sounds suffer when the training data is not adequate for the considered data during deployment. This problem is also known as domain gap problem. In this paper, a methodology for concrete defect detection even when the available training data was collected from a tunnel that differs from the actually inspected tunnel is proposed. The proposed method selects part of the data from the inspection target tunnel, for which labels are not available, to use along traditional labeled training data in the training of a classifier within the semi-supervised support vector machine framework. This selection is conducted using the integration of visual information from an ordinary camera and acoustic information obtained using the hammering test. Experimental results showed that the proposed method yielded satisfying results in the laboratory conditions.
更多查看译文
关键词
hammering test,multimodal classification,domain adaptation,automated defect detection,concrete structures,automated system,popular inspection methods,hammering sounds,considered data,domain gap problem,concrete defect detection,available training data,actually inspected tunnel,inspection target tunnel,traditional labeled training data
AI 理解论文
溯源树
样例
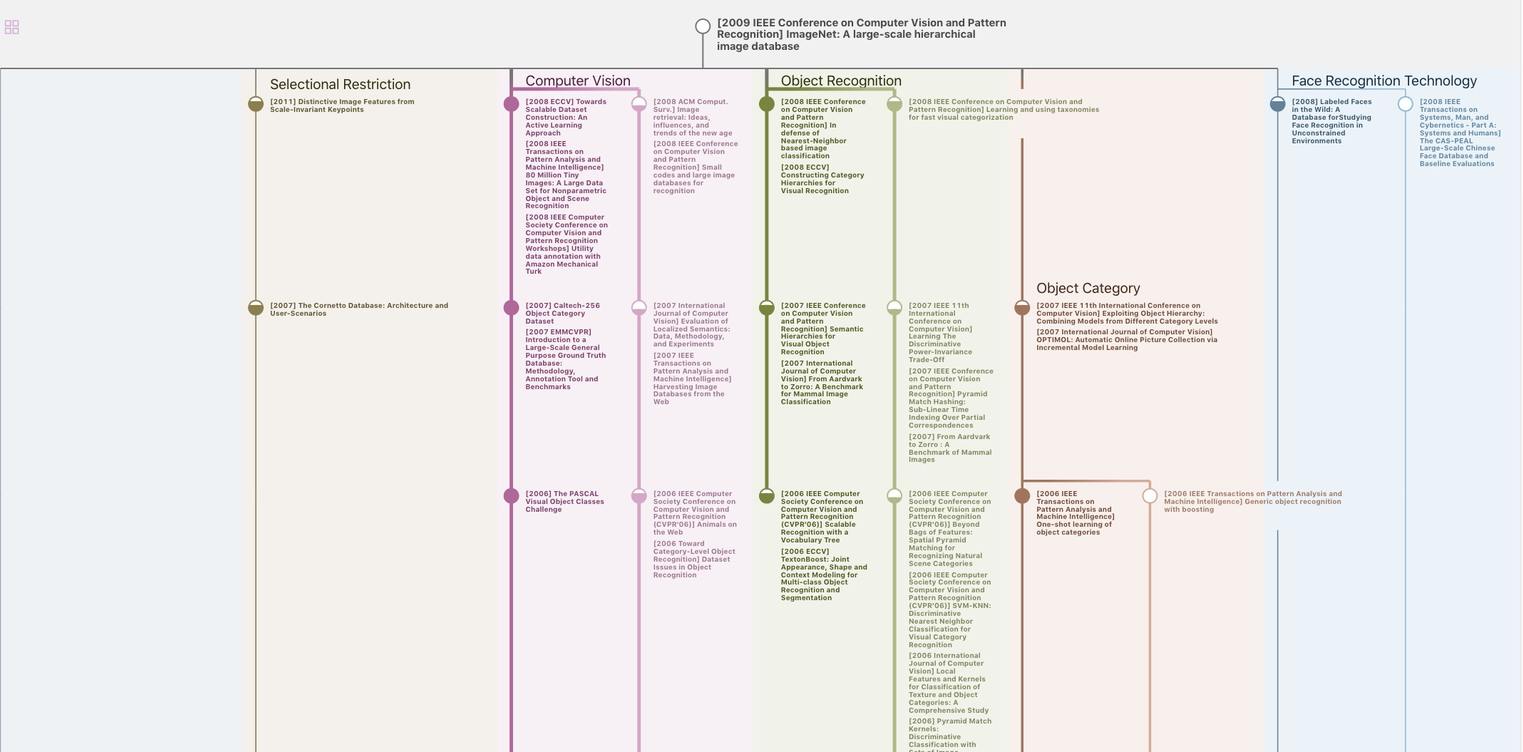
生成溯源树,研究论文发展脉络
Chat Paper
正在生成论文摘要