PPCD-GAN: Progressive Pruning and Class-Aware Distillation for Large-Scale Conditional GANs Compression
2022 IEEE WINTER CONFERENCE ON APPLICATIONS OF COMPUTER VISION (WACV 2022)(2022)
摘要
We push forward neural network compression research by exploiting a novel challenging task of large-scale conditional generative adversarial networks (GANs) compression. To this end, we propose a gradually shrinking GAN (PPCD-GAN) by introducing progressive pruning residual block (PP-Res) and class-aware distillation. The PP-Res is an extension of the conventional residual block where each convolutional layer is followed by a learnable mask layer to progressively prune network parameters as training proceeds. The class-aware distillation, on the other hand, enhances the stability of training by transferring immense knowledge from a well-trained teacher model through instructive attention maps. We train the pruning and distillation processes simultaneously on a well-known GAN architecture in an end-to-end manner. After training, all redundant parameters as well as the mask layers are discarded, yielding a lighter network while retaining the performance. We comprehensively illustrate, on ImageNet 128 x 128 dataset, PPCD-GAN reduces up to 5.2 x (81%) parameters against state-of-the-arts while keeping better performance.
更多查看译文
关键词
Large-scale Vision Applications Deep Learning -> Efficient Training and Inference Methods for Networks, Deep Learning -> Neural Generative Models, Autoencoders, GANs
AI 理解论文
溯源树
样例
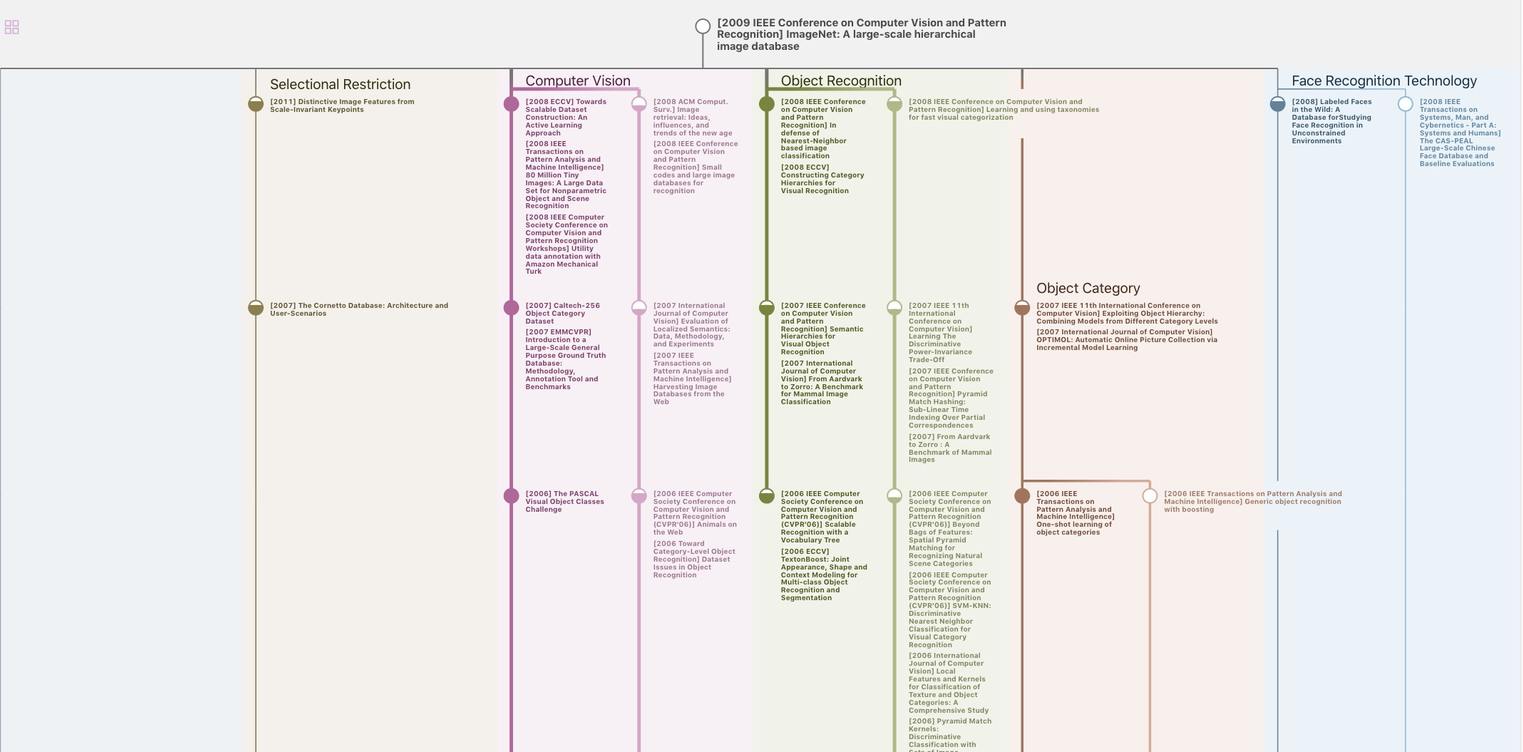
生成溯源树,研究论文发展脉络
Chat Paper
正在生成论文摘要