Automated Accelerator Optimization Aided by Graph Neural Networks
International Symposium on Field Programmable Gate Arrays(2022)
摘要
ABSTRACTHigh-level synthesis (HLS) has freed the computer architects from developing their designs in a very low-level language and needing to exactly specify how the data should be transferred in register-level. With the help of HLS, the hardware designers must describe only a high-level behavioral flow of the design. Despite this, it still can take weeks to develop a high-performance architecture mainly because there are many design choices at a higher level that requires more time to explore. It also takes several minutes to hours to get feedback from the HLS tool on the quality of each design candidate. We propose to solve this problem by modeling the HLS tool with a graph neural network (GNN) that is trained to be used for a wide range of applications [1]. The experimental results demonstrate that by employing the GNN-based model, we are able to estimate the quality of design in milliseconds with high accuracy which results in an average speedup of 55x for optimizing the design compared to the previous state-of-the-art work; hence, it can help us search through the solution space very quickly.
更多查看译文
AI 理解论文
溯源树
样例
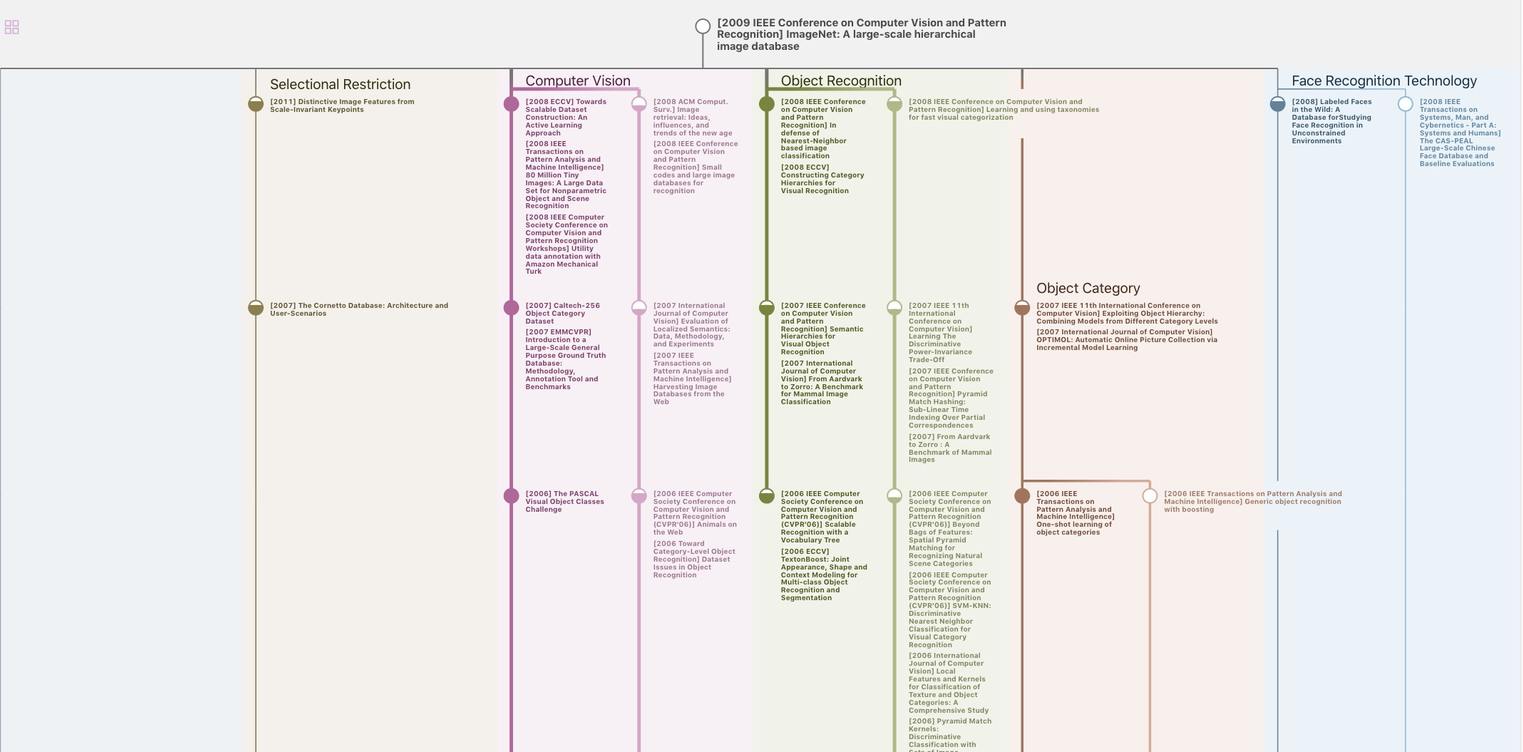
生成溯源树,研究论文发展脉络
Chat Paper
正在生成论文摘要