Self-Optimization of Cellular Networks Using Deep Reinforcement Learning with Hybrid Action Space
2022 IEEE 19th Annual Consumer Communications & Networking Conference (CCNC)(2022)
摘要
Wireless networks have been going through tremendous proliferation recently. As a result, a continuous configuration and management are necessary to sustain a balanced performance while facing such continued growth and endless changes. A self-managed network is required to replace manual management, which is costly, time-consuming, and error-prone. In this paper, we propose a machine-learning-based cellular network management system. The proposed system aims to enhance the network stability and adaptability to temporal changes (e.g., load imbalances across cells). The presented approach is a deep reinforcement learning scheme that enables a network manager to learn a policy that maximizes the network average sum throughput while trying to minimize the consumed energy and the number of blocked users. In addition to controlling the transmitted power and the cell individual offset, MIMO can be switched ON and OFF to control the consumed energy without affecting the quality of service. This results in a hybrid action space, i.e., our action vector has some binary actions as well as continuous actions. We present a novel algorithm to deal with this hybrid action space. Our results reveal that our proposed algorithm is flexible, efficient, and reliable. We report significant performance gains compared to some baselines (without self-management) and previously proposed algorithms.
更多查看译文
关键词
Reinforcement learning,mobile networks,MIMO,Machine Learning
AI 理解论文
溯源树
样例
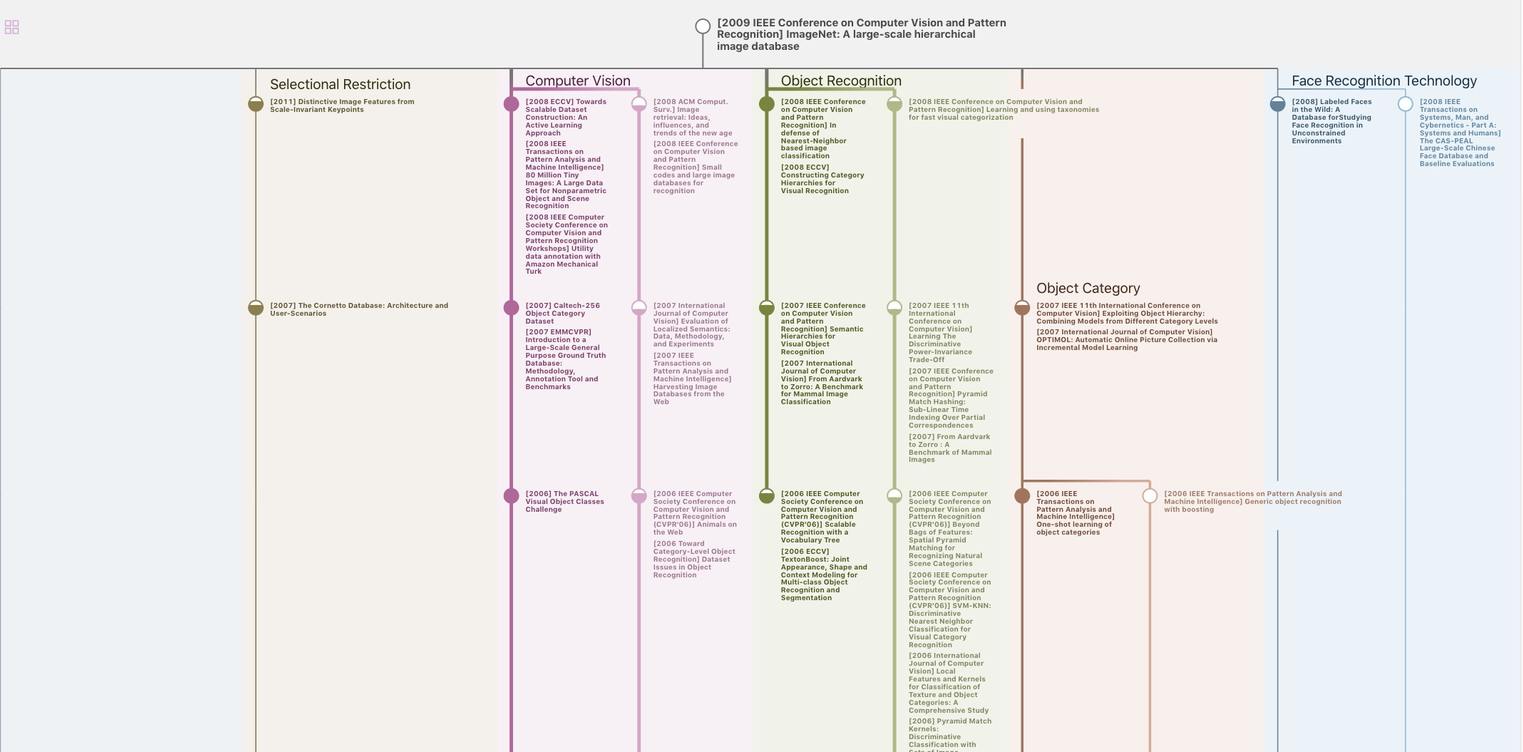
生成溯源树,研究论文发展脉络
Chat Paper
正在生成论文摘要