Improving Session Search by Modeling Multi-Granularity Historical Query Change
WSDM(2022)
摘要
ABSTRACTIn session search, it's important to utilize historical interactions between users and the search engines to improve document retrieval. However, not all historical information contributes to document ranking. Users often express their preferences in the process of modifying the previous query, which can help us catch useful information in the historical interactions. Inspired by it, we propose to model historical query change to improve document ranking performance. Especially, we characterize multi-granularity query change between each pair of adjacent queries at both term level and semantic level. For term level query change, we calculate three types of term weights, including the retained term weights, added term weights and removed term weights. Then we perform term-based interaction between the candidate document and historical queries based on the term weights. For semantic level query change, we calculate an overall representation of user intent by integrating the representations of each historical query obtained by different types of term weights. Then we adopt representation-based matching between this representation and the candidate document. To improve the effect of query change modeling, we introduce query change classification as an auxiliary task. Experimental results on AOL and TianGong-ST search logs show that our model outperforms most existing models for session search.
更多查看译文
关键词
Session Search, Query Change, Document Ranking
AI 理解论文
溯源树
样例
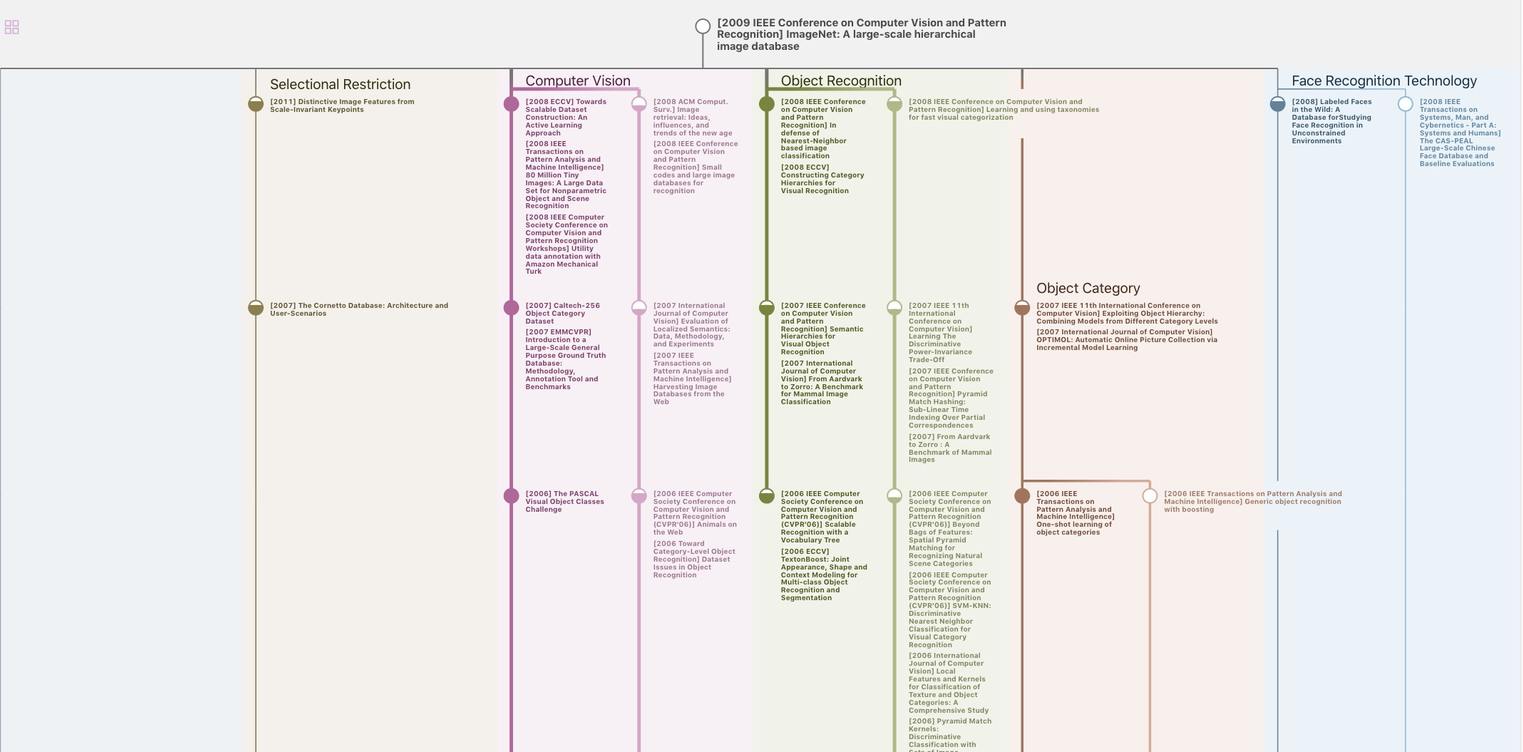
生成溯源树,研究论文发展脉络
Chat Paper
正在生成论文摘要