Identifying Cost-effective Debunkers for Multi-stage Fake News Mitigation Campaigns
WSDM(2022)
摘要
ABSTRACTOnline social networks have become a fertile ground for spreading fake news. Methods to automatically mitigate fake news propagation have been proposed. Some studies focus on selecting top k influential users on social networks as debunkers, but the social influence of debunkers may not translate to wide mitigation information propagation as expected. Other studies assume a given set of debunkers and focus on optimizing intensity for debunkers to publish true news, but as debunkers are fixed, even if with high social influence and/or high intensity to post true news, the true news may not reach users exposed to fake news and therefore mitigation effect may be limited. In this paper, we propose the multi-stage fake news mitigation campaign where debunkers are dynamically selected within budget at each stage. We formulate it as a reinforcement learning problem and propose a greedy algorithm optimized by predicting future states so that the debunkers can be selected in a way that maximizes the overall mitigation effect. We conducted extensive experiments on synthetic and real-world social networks and show that our solution outperforms state-of-the-art baselines in terms of mitigation effect.
更多查看译文
关键词
Fake News Mitigation, Social Network, Reinforcement Learning, Multivariate Hawkes Process
AI 理解论文
溯源树
样例
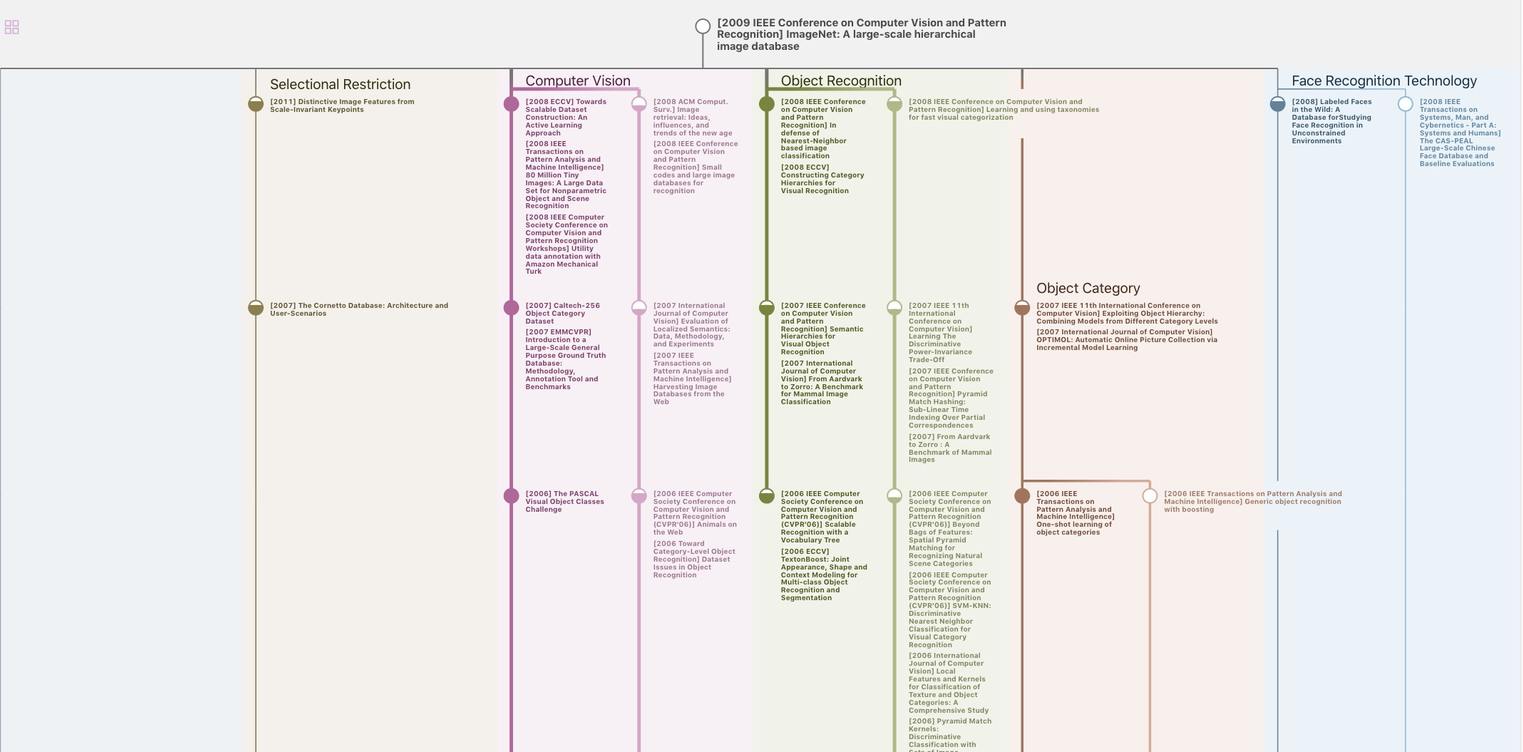
生成溯源树,研究论文发展脉络
Chat Paper
正在生成论文摘要