Scope-aware Re-ranking with Gated Attention in Feed
WSDM(2022)
摘要
ABSTRACTModern recommendation systems introduce the re-ranking stage to optimize the entire list directly. This paper focuses on the design of re-ranking framework in feed to optimally model the mutual influence between items and further promote user engagement. On mobile devices, users browse the feed almost in a top-down manner and rarely compare items back and forth. Besides, users often compare item with its adjacency based on their partial observations. Given the distinct user behavior patterns, the modeling of mutual influence between items should be carefully designed. Existing re-ranking models encode the mutual influence between items with sequential encoding methods. However, previous works may be dissatisfactory due to the ignorance of connections between items on different scopes. In this paper, we first discuss Unidirectivity and Locality on the impacts and consequences, then report corresponding solutions in industrial applications. We propose a novel framework based on the empirical evidence from user analysis. To address the above problems, we design a \underlineS cope-aware \underlineR e-ranking with \underlineG ated \underlineA ttention model (SRGA ) to emulate the user behavior patterns from two aspects: 1) we emphasize the influence along the user's common browsing direction; 2) we strength the impacts of pivotal adjacent items within the user visual window. Specifically, we design a global scope attention to encode inter-item patterns unidirectionally from top to bottom. Besides, we devise a local scope attention sliding over the recommendation list to underline interactions among neighboring items. Furthermore, we design a learned gate mechanism to aggregating the information dynamically from local and global scope attention. Extensive offline experiments and online A/B testing demonstrate the benefits of our novel framework. The proposed SRGA model achieves the best performance in offline metrics compared with the state-of-the-art re-ranking methods. Further, empirical results on live traffic validate that our recommender system, equipped with SRGA in the re-ranking stage, improves significantly in user engagement.
更多查看译文
关键词
Recommender System, Learing To Rank, Re-ranking
AI 理解论文
溯源树
样例
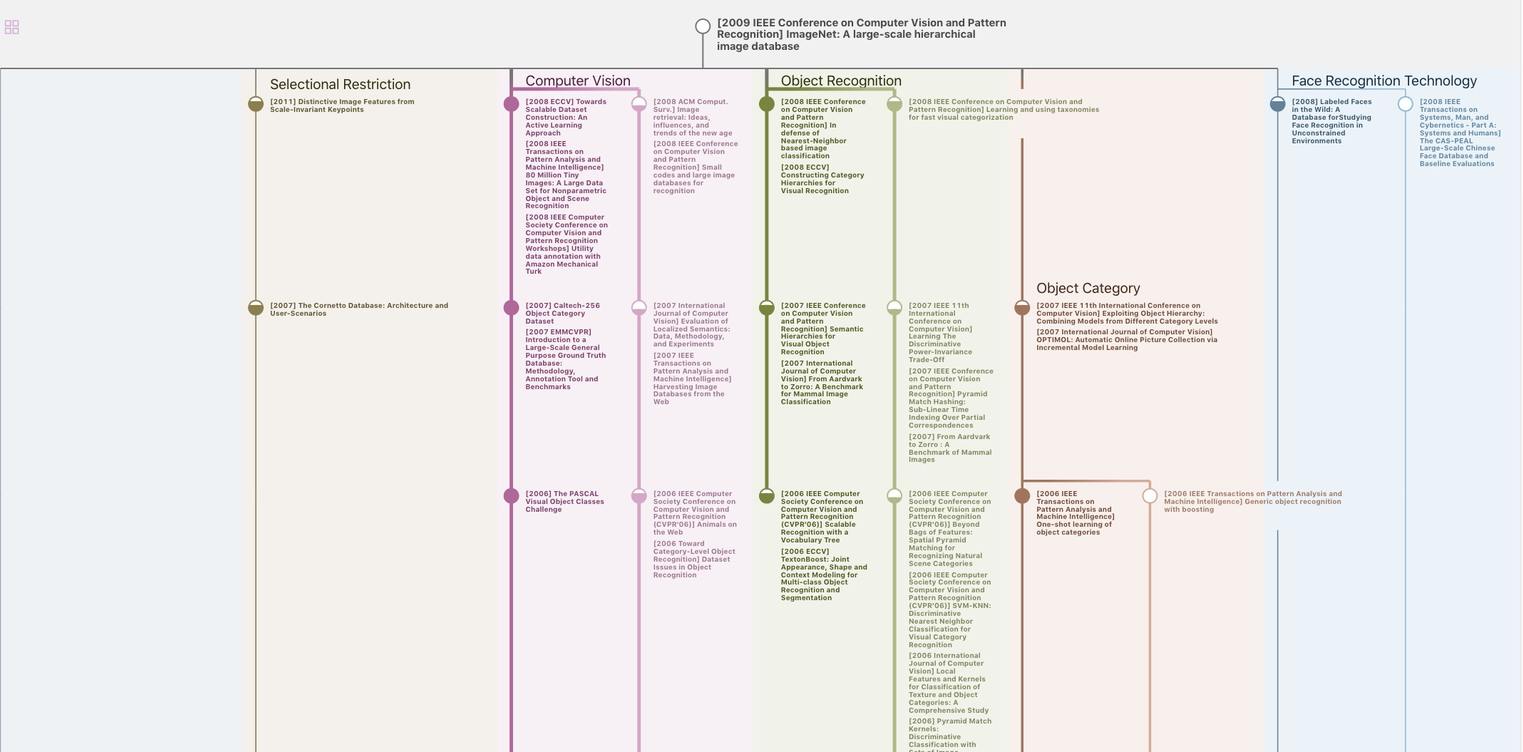
生成溯源树,研究论文发展脉络
Chat Paper
正在生成论文摘要