ComGA: Community-Aware Attributed Graph Anomaly Detection
WSDM(2022)
摘要
ABSTRACTGraph anomaly detection, here, aims to find rare patterns that are significantly different from other nodes. Attributed graphs containing complex structure and attribute information are ubiquitous in our life scenarios such as bank account transaction graph and paper citation graph. Anomalous nodes on attributed graphs show great difference from others in the perspectives of structure and attributes, and give rise to various types of graph anomalies. In this paper, we investigate three types of graph anomalies: local, global, and structure anomalies. And, graph neural networks (GNNs) based anomaly detection methods attract considerable research interests due to the power of modeling attributed graphs. However, the convolution operation of GNNs aggregates neighbors information to represent nodes, which makes node representations more similar and cannot effectively distinguish between normal and anomalous nodes, thus result in sub-optimal results. To improve the performance of anomaly detection, we propose a novel community-aware attributed graph anomaly detection framework (ComGA). We design a tailored deep graph convolutional network (tGCN) to anomaly detection on attributed graphs. Extensive experiments on eight real-life graph datasets demonstrate the effectiveness of ComGA.
更多查看译文
关键词
Anomaly Detection, Community Structure, Attributed Graphs, Graph Neural Networks
AI 理解论文
溯源树
样例
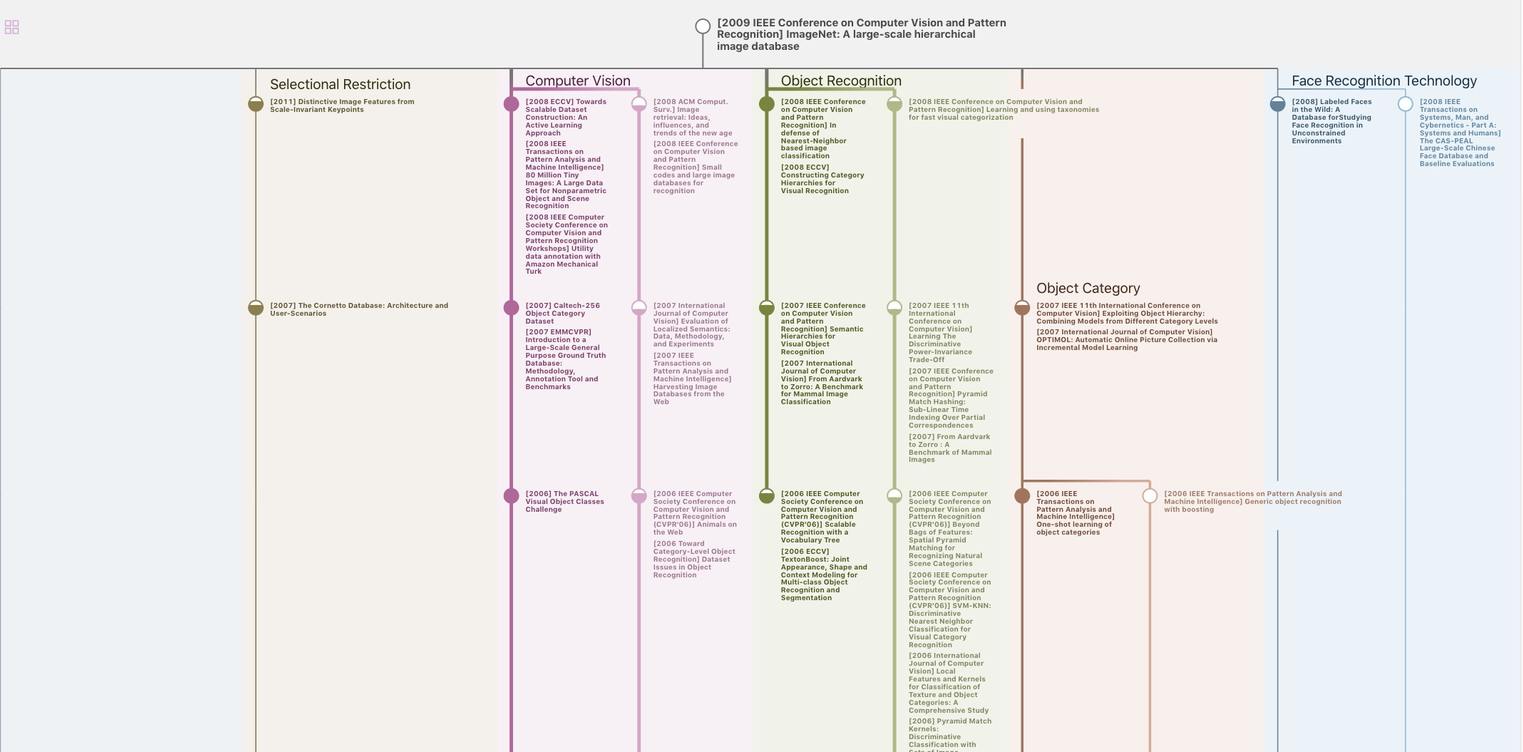
生成溯源树,研究论文发展脉络
Chat Paper
正在生成论文摘要