Improving Knowledge Tracing with Collaborative Information
WSDM(2022)
摘要
ABSTRACTKnowledge tracing, which estimates students' knowledge states by predicting the probability that they correctly answer questions, is an essential task for online learning platforms. It has gained much attention in the decades due to its importance to downstream tasks like learning material arrangement, etc. The previous deep learning-based methods trace students' knowledge states with the explicitly intra-student information, i.e., they only consider the historical information of individuals to make predictions. However, they neglect the inter-student information, which contains the response correctness of other students who have similar question-answering experiences, may offer some valuable clues. Based on this consideration, we propose a method called Collaborative Knowledge Tracing (CoKT) in this paper, which sufficiently exploits the inter-student information in knowledge tracing. It retrieves the sequences of peer students who have similar question-answering experiences to obtain the inter-student information, and integrates the inter-student information with the intra-student information to trace students' knowledge states and predict their correctness in answering questions. We validate the effectiveness of our method on four real-world datasets and compare it with 11 baselines. The experimental results reveal that CoKT achieves the best performance.
更多查看译文
关键词
knowledge tracing, sequence retrieval, correctness prediction
AI 理解论文
溯源树
样例
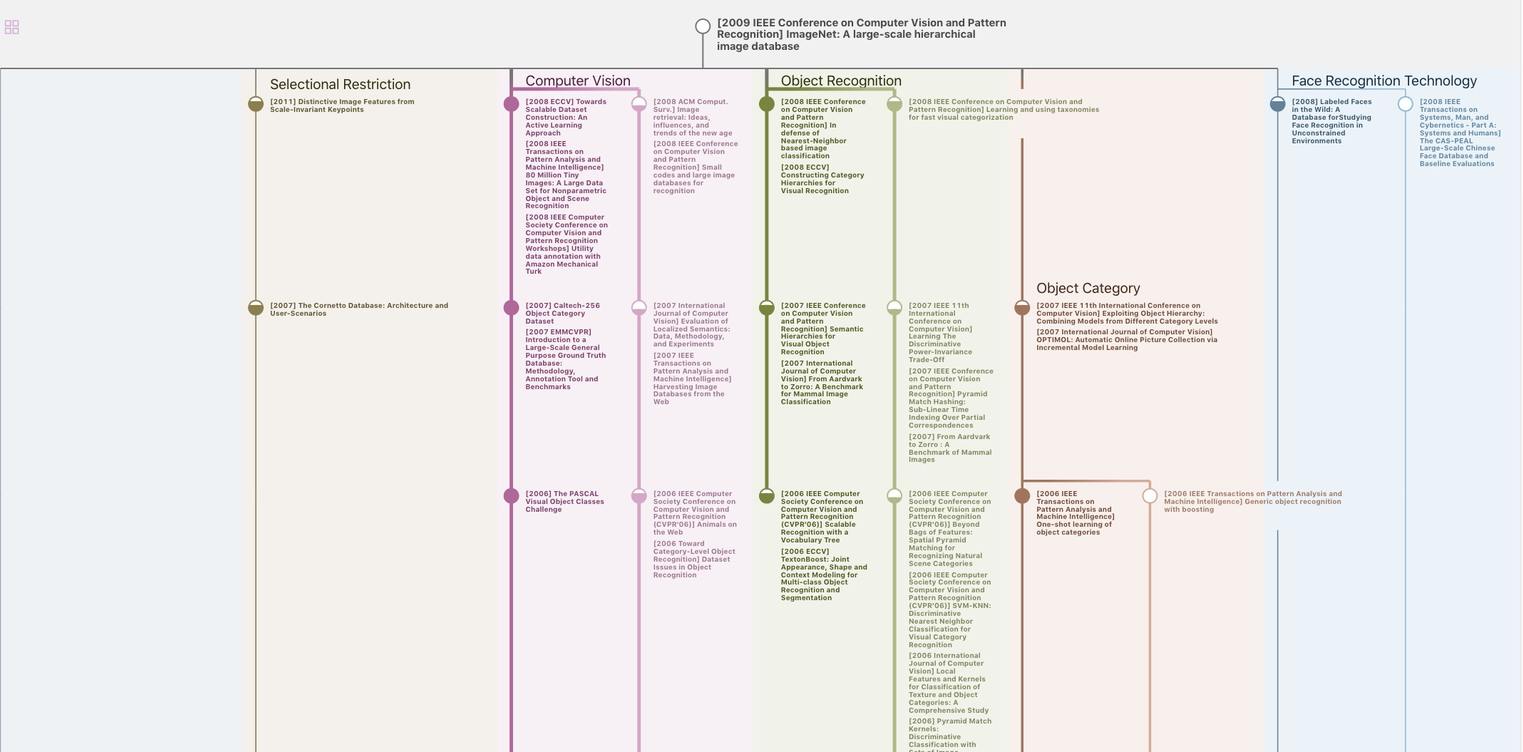
生成溯源树,研究论文发展脉络
Chat Paper
正在生成论文摘要