Cluster-Aware Heterogeneous Information Network Embedding
WSDM(2022)
摘要
ABSTRACTHeterogeneous Information Network (HIN) embedding refers to the low-dimensional projections of the HIN nodes that preserve the HIN structure and semantics. HIN embedding has emerged as a promising research field for network analysis as it enables downstream tasks such as clustering and node classification. In this work, we propose VaCA-HINE for joint learning of cluster embeddings as well as cluster-aware HIN embedding. We assume that the connected nodes are highly likely to fall in the same cluster, and adopt a variational approach to preserve the information in the pairwise relations in a cluster-aware manner. In addition, we deploy contrastive modules to simultaneously utilize the information in multiple meta-paths, thereby alleviating the meta-path selection problem - a challenge faced by many of the famous HIN embedding approaches. The HIN embedding, thus learned, not only improves the clustering performance but also preserves pairwise proximity as well as the high-order HIN structure. We show the effectiveness of our approach by comparing it with many competitive baselines on three real-world datasets on clustering and downstream node classification.
更多查看译文
关键词
Heterogeneous Information Network, Variational methods, Network Clustering, Network Embedding
AI 理解论文
溯源树
样例
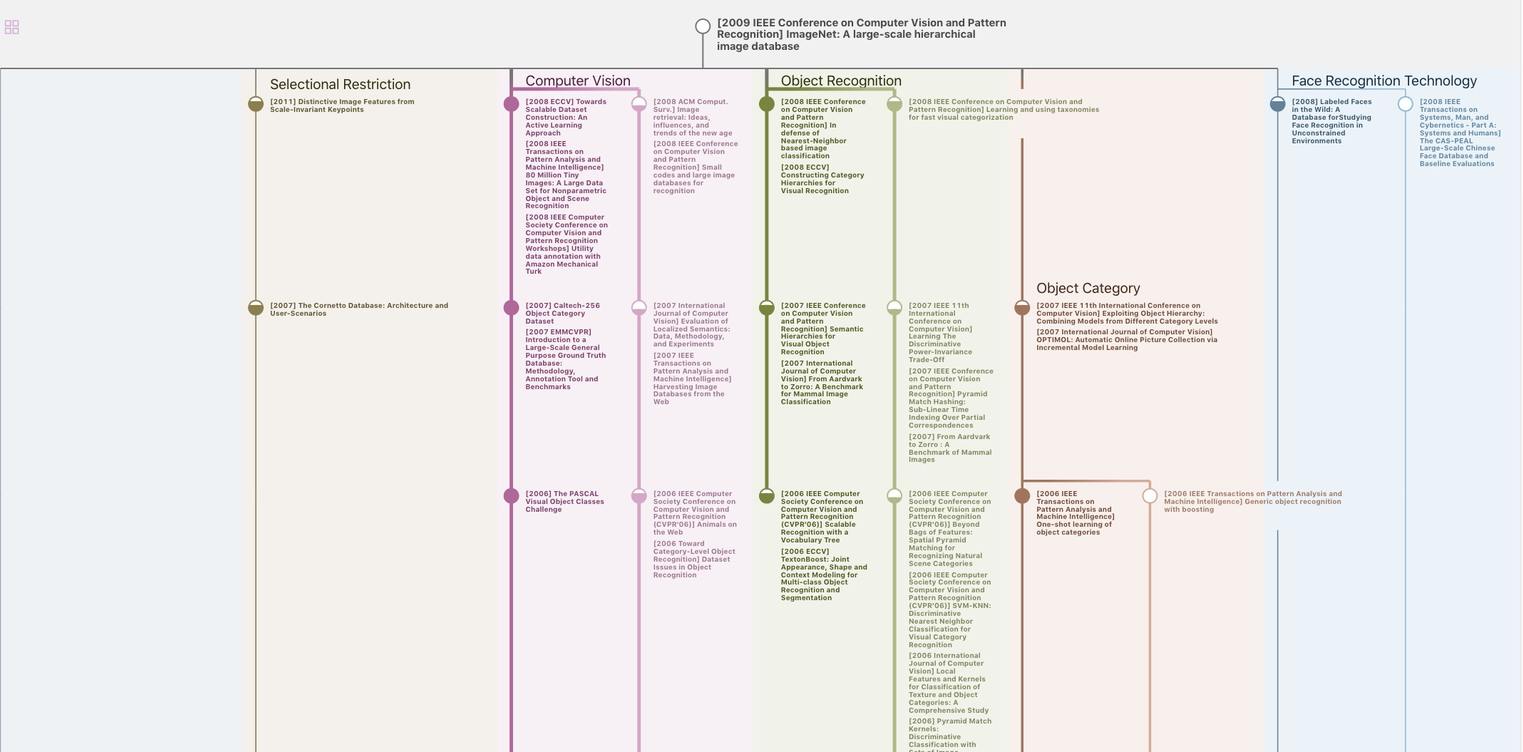
生成溯源树,研究论文发展脉络
Chat Paper
正在生成论文摘要