KGNN: Harnessing Kernel-based Networks for Semi-supervised Graph Classification
WSDM(2022)
摘要
ABSTRACTThis paper studies semi-supervised graph classification, which is an important problem with various applications in social network analysis and bioinformatics. This problem is typically solved by using graph neural networks (GNNs), which yet rely on a large number of labeled graphs for training and are unable to leverage unlabeled graphs. We address the limitations by proposing the Kernel-based Graph Neural Network (KGNN). A KGNN consists of a GNN-based network as well as a kernel-based network parameterized by a memory network. The GNN-based network performs classification through learning graph representations to implicitly capture the similarity between query graphs and labeled graphs, while the kernel-based network uses graph kernels to explicitly compare each query graph with all the labeled graphs stored in a memory for prediction. The two networks are motivated from complementary perspectives, and thus combing them allows KGNN to use labeled graphs more effectively. We jointly train the two networks by maximizing their agreement on unlabeled graphs via posterior regularization, so that the unlabeled graphs serve as a bridge to let both networks mutually enhance each other. Experiments on a range of well-known benchmark datasets demonstrate that KGNN achieves impressive performance over competitive baselines.
更多查看译文
关键词
Graph Classification, Graph Neural Networks, Graph Kernels, Semi-supervised Learning
AI 理解论文
溯源树
样例
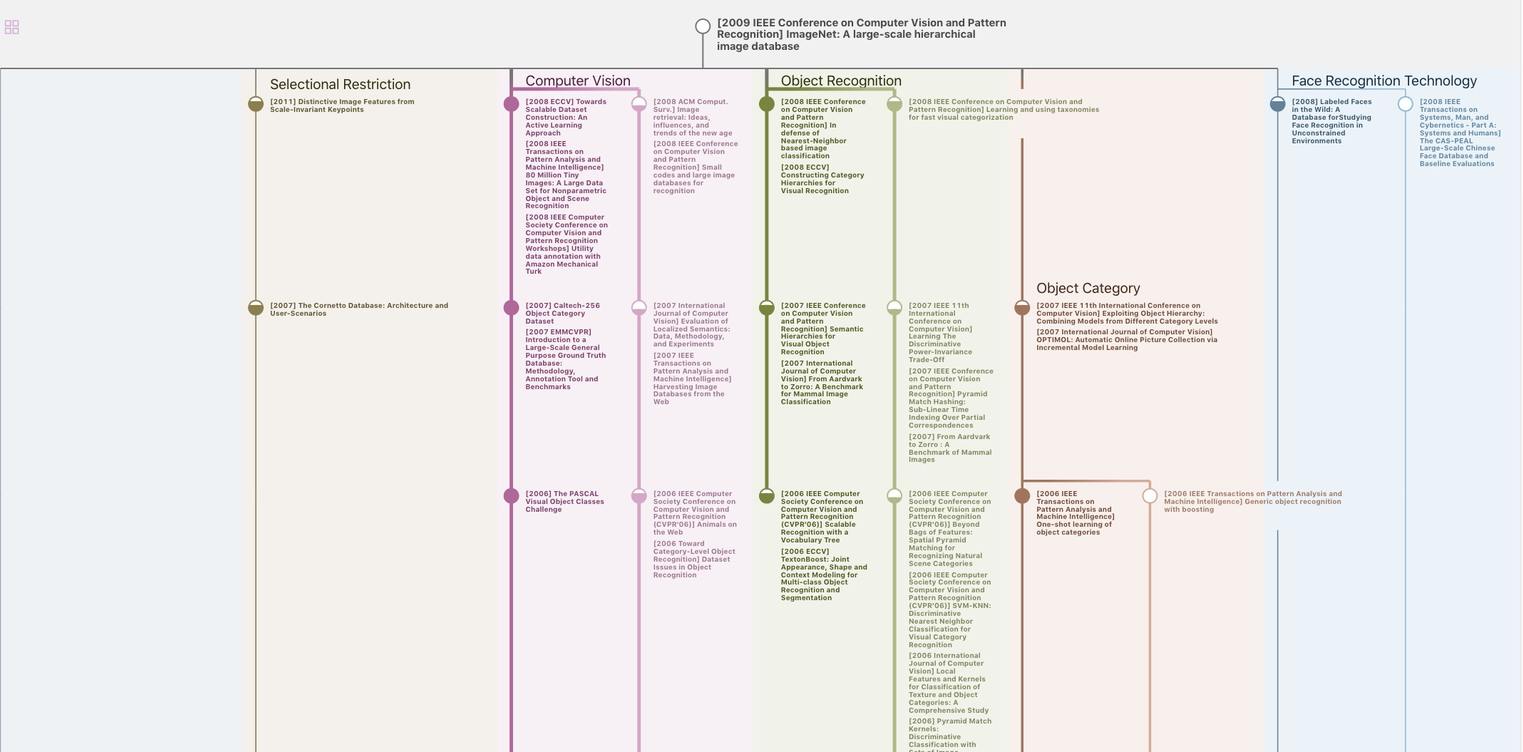
生成溯源树,研究论文发展脉络
Chat Paper
正在生成论文摘要