Utilizing Half Convolutional Autoencoder to Generate User and Item Vectors for Initialization in Matrix Factorization
FUTURE INTERNET(2022)
摘要
Recommendation systems based on convolutional neural network (CNN) have attracted great attention due to their effectiveness in processing unstructured data such as images or audio. However, a huge amount of raw data produced by data crawling and digital transformation is structured, which makes it difficult to utilize the advantages of CNN. This paper introduces a novel autoencoder, named Half Convolutional Autoencoder, which adopts convolutional layers to discover the high-order correlation between structured features in the form of Tag Genome, the side information associated with each movie in the MovieLens 20 M dataset, in order to generate a robust feature vector. Subsequently, these new movie representations, along with the introduction of users' characteristics generated via Tag Genome and their past transactions, are applied into well-known matrix factorization models to resolve the initialization problem and enhance the predicting results. This method not only outperforms traditional matrix factorization techniques by at least 5.35% in terms of accuracy but also stabilizes the training process and guarantees faster convergence.
更多查看译文
关键词
autoencoder, collaborative filtering, convolutional neural network, matrix factorization, recommendation system
AI 理解论文
溯源树
样例
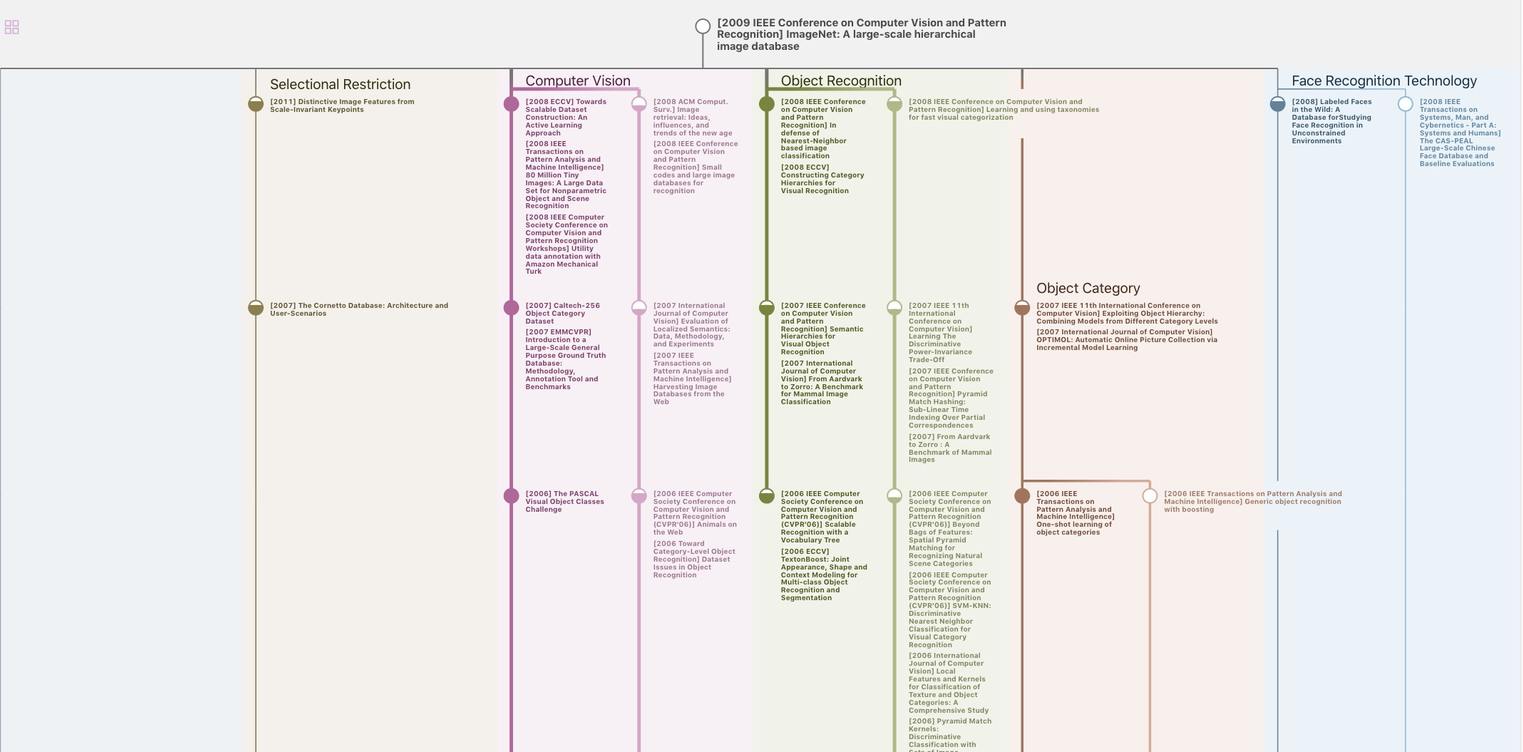
生成溯源树,研究论文发展脉络
Chat Paper
正在生成论文摘要