DLUP: A Deep Learning Utility Prediction Scheme for Solid-State Fermentation Services in IIoT
IEEE Transactions on Industrial Informatics(2022)
摘要
At present, solid-state fermentation (SSF) is mainly controlled by artificial experience, and the product quality and yield are not stable. Therefore, predicting the quality and yield of SSF is of great significance for improving the utility of SSF. In this article, we propose a deep learning utility prediction (DLUP) scheme for the SSF in the Industrial Internet of Things, including parameters collection and utility prediction of the SSF process. Furthermore, we propose a novel edge-rewritable Petri net to model the parameters collection and utility prediction of the SSF process and further verify their soundness. More importantly, DLUP combines the generating ability of least squares generative adversarial network with the predicting ability of fully connected neural network to realize the utility prediction (usually use the alcohol concentration) of SSF. Experiments show that the proposed method predicts the alcohol concentration more accurately than the other joint prediction methods. In addition, the method in our article provides evidences for setting the ratio of raw materials and proper temperature through numerical analysis.
更多查看译文
关键词
Fully connected neural network,least squares generative adversarial network,Petri net,solid-state fermentation,utility prediction
AI 理解论文
溯源树
样例
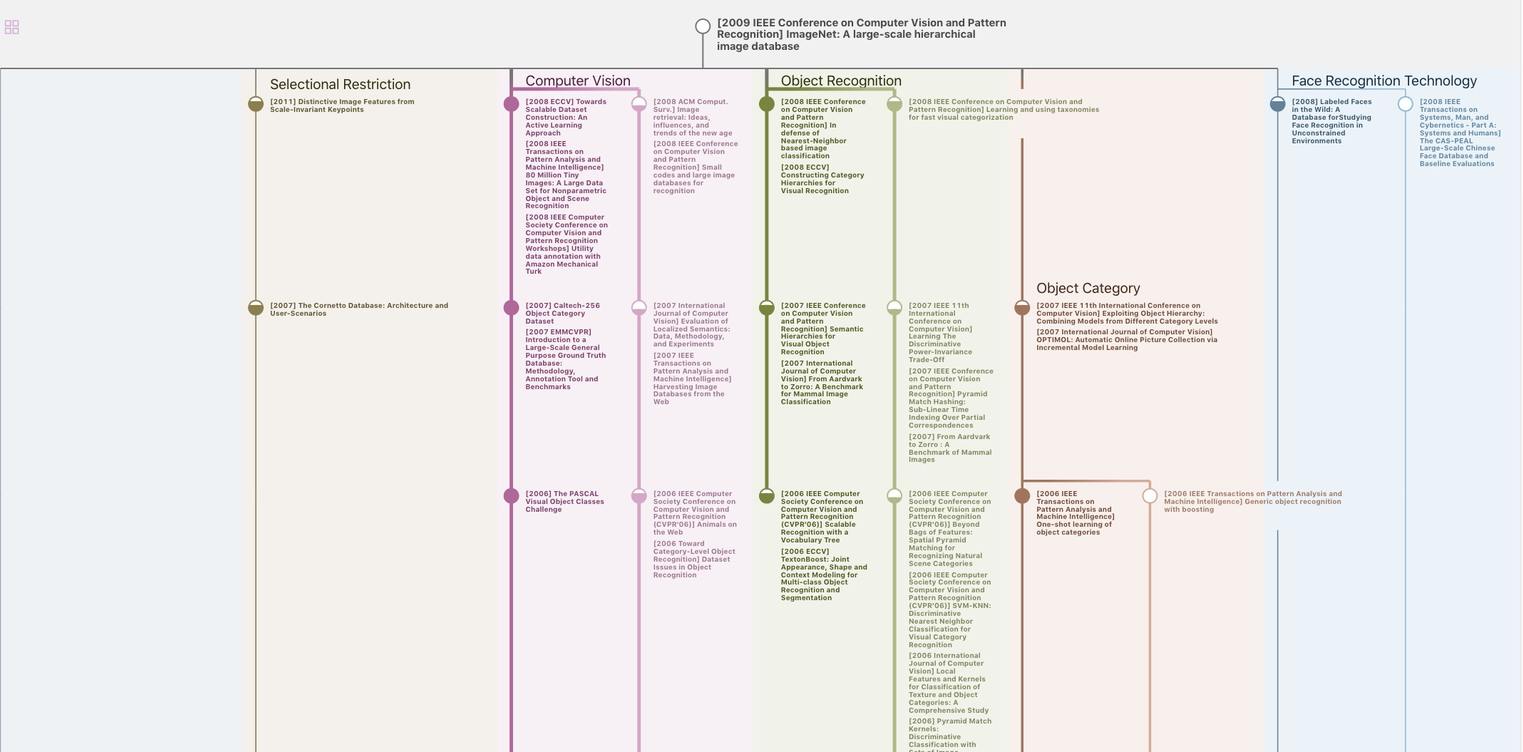
生成溯源树,研究论文发展脉络
Chat Paper
正在生成论文摘要