Error bounds of adversarial bipartite ranking
Neurocomputing(2022)
摘要
Generalization analysis for learning models with adversarial examples has attracted increasing attentions recently. However, the previous theoretical results are usually limited to learning models with the pointwise loss. In this paper, we beyond this restriction by investigating generalization ability of bipartite ranking associated with adversarial perturbations. The upper bounds of adversarial ranking risk are established by formulating the pairwise learning in a minimax framework and introducing the transfer mapping to relate data distributions. In particular, our results are suitable to general loss satisfying Lipschitz conditions, e.g, the logistic loss and the least squared loss.
更多查看译文
关键词
Adversarial examples,bipartite ranking,Error analysis,Rademacher complexity,Reproducing kernel Hilbert space
AI 理解论文
溯源树
样例
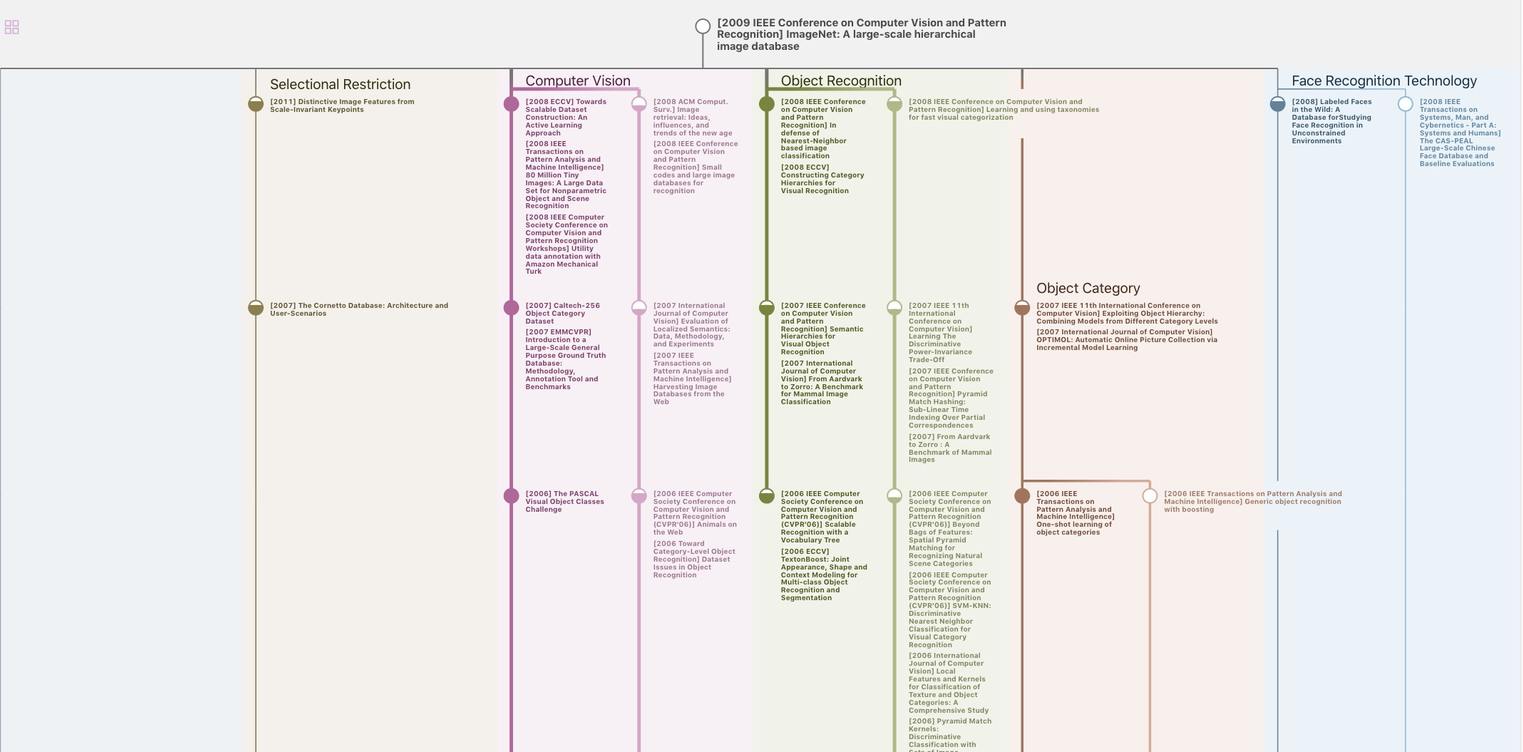
生成溯源树,研究论文发展脉络
Chat Paper
正在生成论文摘要