Three-Dimensional Sparse SAR Imaging with Generalized L-q Regularization
REMOTE SENSING(2022)
摘要
Three-dimensional (3D) synthetic aperture radar (SAR) imaging provides complete 3D spatial information, which has been used in environmental monitoring in recent years. Compared with matched filtering (MF) algorithms, the regularization technique can improve image quality. However, due to the substantial computational cost, the existing observation-matrix-based sparse imaging algorithm is difficult to apply to large-scene and 3D reconstructions. Therefore, in this paper, novel 3D sparse reconstruction algorithms with generalized L-q-regularization are proposed. First, we combine majorization-minimization (MM) and L-1 regularization (MM-L-1) to improve SAR image quality. Next, we combine MM and L-1/2 regularization (MM-L-1/2) to achieve high-quality 3D images. Then, we present the algorithm which combines MM and L-0 regularization (MM-L-0) to obtain 3D images. Finally, we present a generalized MM-L-q algorithm (GMM-L-q) for sparse SAR imaging problems with arbitrary q(0 & LE;q & LE;1) values. The proposed algorithm can improve the performance of 3D SAR images, compared with existing regularization techniques, and effectively reduce the amount of calculation needed. Additionally, the reconstructed complex image retains the phase information, which makes the reconstructed SAR image still suitable for interferometry applications. Simulation and experimental results verify the effectiveness of the algorithms.
更多查看译文
关键词
three-dimensional (3D) sparse imaging, synthetic aperture radar (SAR), majorization-minimization (MM), L-q-regularization
AI 理解论文
溯源树
样例
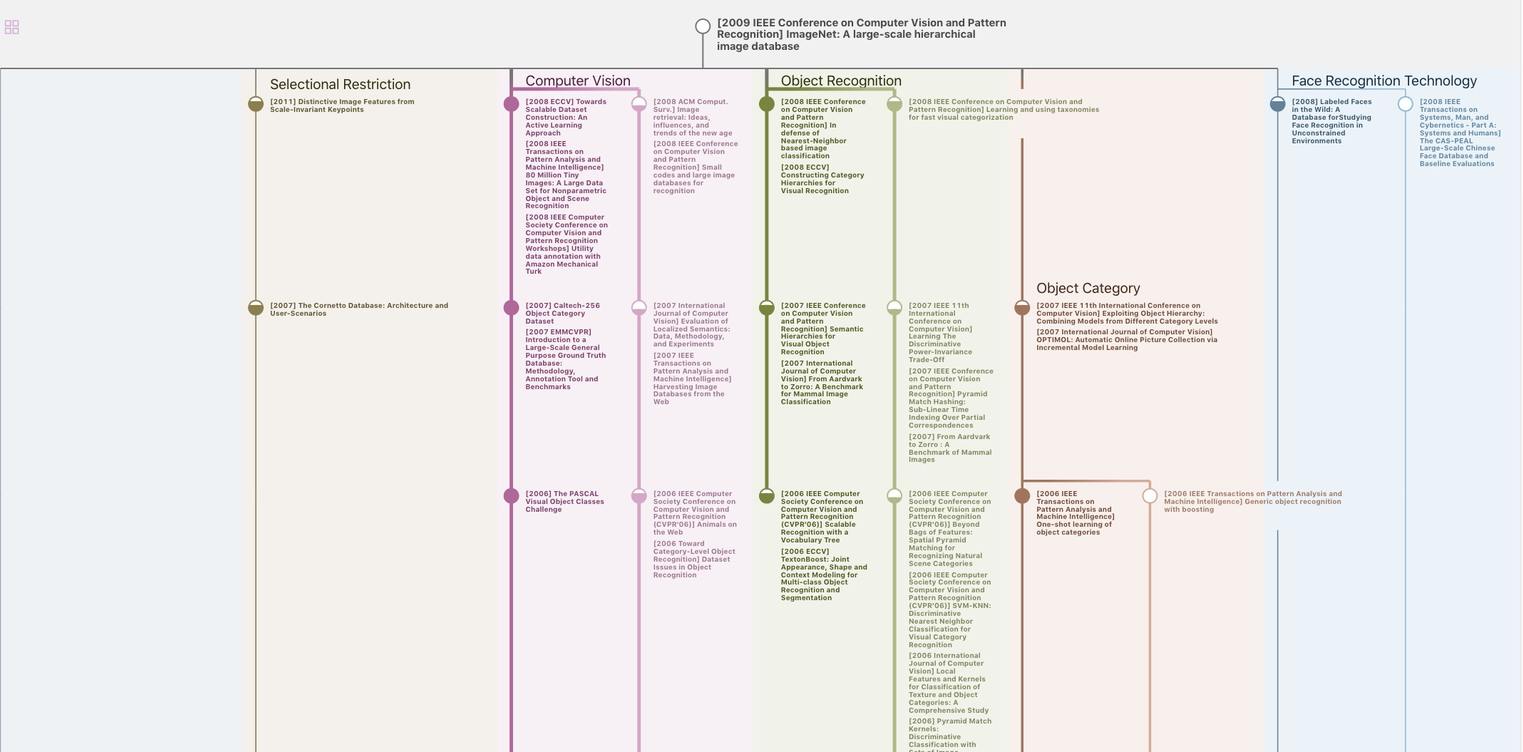
生成溯源树,研究论文发展脉络
Chat Paper
正在生成论文摘要