Online feature selection for multi-source streaming features
Information Sciences(2022)
摘要
Multi-source streaming feature selection in an online manner has attracted considerable attention, from researchers because it can reduce the dimensionality of heterogeneous big data. However, traditional online algorithms such as Alpha-investing, Online Streaming Feature Selection (OSFS), Online Group Feature Selection (OGFS) and Scalable and Accurate OnLine Approach (SAOLA) consider only a single data source with fixed instances, and are not directly applicable to multi-source data. Multi-source Causal Feature Selection (MCFS) can search for an invariant set in multiple interventional datasets. However, fixed feature spaces are restrained, and exactly these same features are required among multi-source data. To overcome these limitations, we propose a novel method known as Multi-source Streaming Feature Selection (MSFS) to tackle the feature selection problem for multi-source streaming features. The MSFS algorithm addresses a new feature from a random source in three phases: relevance, intra-source redundancy, and inter-source redundancy analyses. That is, MSFS attempts to mine the potential relationships among different data sources rather than only independently consider each data source. In particular, each new feature is analyzed online using the overlapping instances from all data sources, and the Markov blanket (MB) of the target variable is dynamically adjusted. To evaluate the performance of the MSFS algorithm, we compare it with that of the abovementioned algorithms on 14 datasets and two real-world scenarios. The results demonstrate that MSFS outperforms the existing algorithms in classification accuracy and number of selected features.
更多查看译文
关键词
Multi-source,Streaming features,Feature selection,Markov blanket
AI 理解论文
溯源树
样例
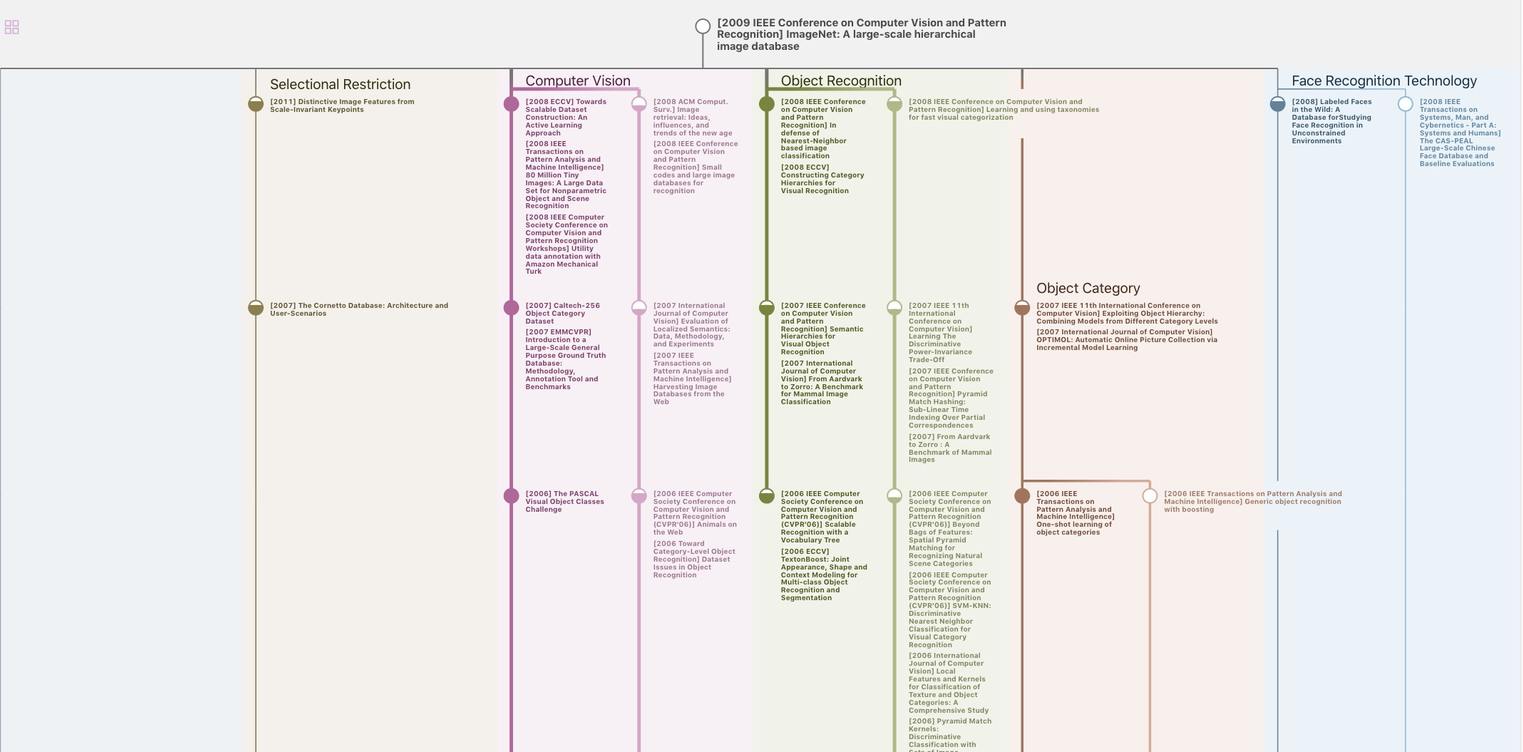
生成溯源树,研究论文发展脉络
Chat Paper
正在生成论文摘要