A hybrid model with novel feature selection method and enhanced voting method for credit scoring
JOURNAL OF INTELLIGENT & FUZZY SYSTEMS(2022)
摘要
With the in-depth application of artificial intelligence technology in the financial field, credit scoring models constructed by machine learning algorithms have become mainstream. However, the high-dimensional and complex attribute features of the borrower pose challenges to the predictive competence of the model. This paper proposes a hybrid model with a novel feature selection method and an enhanced voting method for credit scoring. First, a novel feature selection combined method based on a genetic algorithm (FSCM-GA) is proposed, in which different classifiers are used to select features in combination with a genetic algorithm and combine them to generate an optimal feature subset. Furthermore, an enhanced voting method (EVM) is proposed to integrate classifiers, with the aim of improving the classification results in which the prediction probability values are close to the threshold. Finally, the predictive competence of the proposed model was validated on three public datasets and five evaluation metrics (accuracy, AUC, F-score, Log loss and Brier score). The comparative experiment and significance test results confirmed the good performance and robustness of the proposed model.
更多查看译文
关键词
Credit scoring, hybrid model, feature selection, machine learning, ensemble learning
AI 理解论文
溯源树
样例
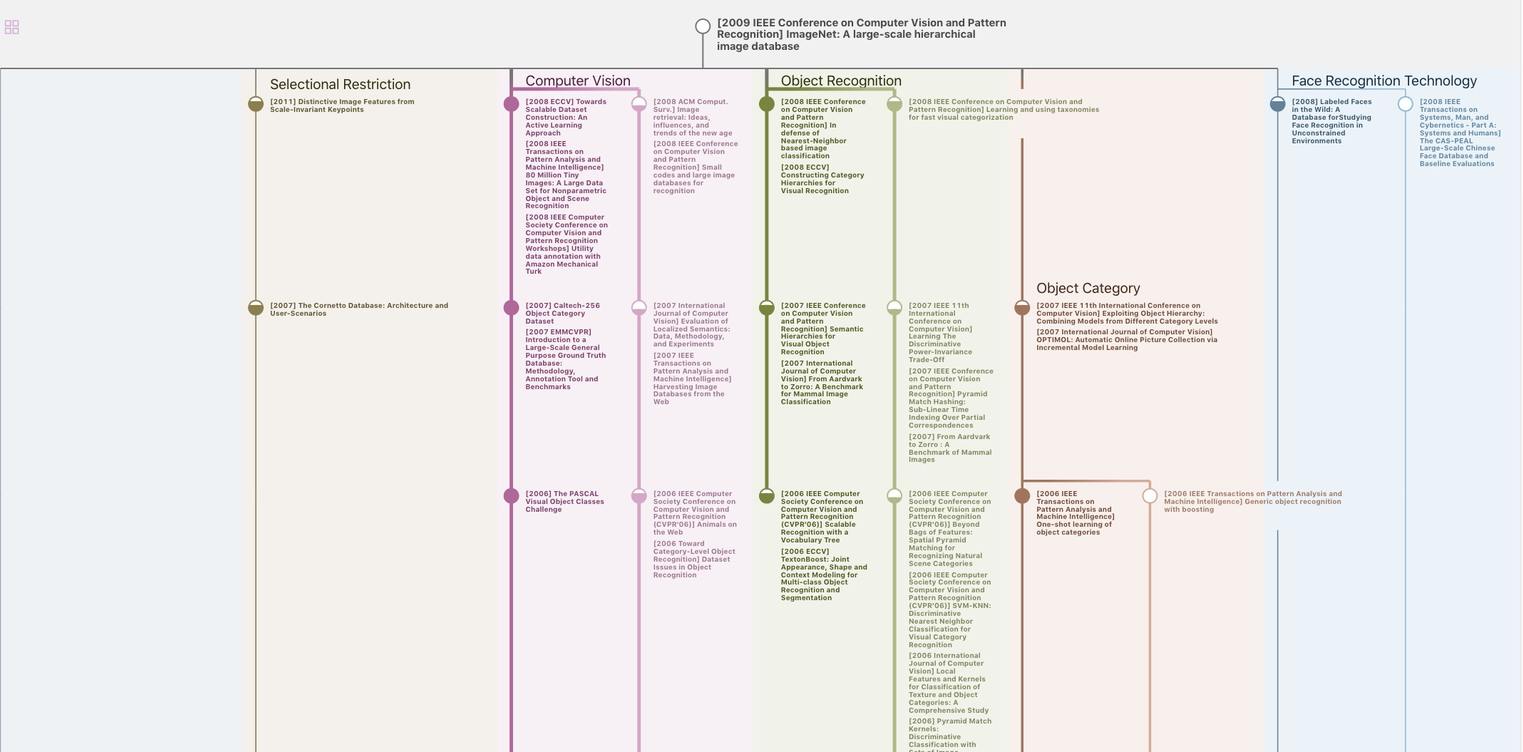
生成溯源树,研究论文发展脉络
Chat Paper
正在生成论文摘要