Adapt-Kcr: a novel deep learning framework for accurate prediction of lysine crotonylation sites based on learning embedding features and attention architecture
BRIEFINGS IN BIOINFORMATICS(2022)
摘要
Protein lysine crotonylation (Kcr) is an important type of posttranslational modification that is associated with a wide range of biological processes. The identification of Kcr sites is critical to better understanding their functional mechanisms. However, the existing experimental techniques for detecting Kcr sites are cost-ineffective, to a great need for new computational methods to address this problem. We here describe Adapt-Kcr, an advanced deep learning model that utilizes adaptive embedding and is based on a convolutional neural network together with a bidirectional long short-term memory network and attention architecture. On the independent testing set, Adapt-Kcr outperformed the current state-of-the-art Kcr prediction model, with an improvement of 3.2% in accuracy and 1.9% in the area under the receiver operating characteristic curve. Compared to other Kcr models, Adapt-Kcr additionally had a more robust ability to distinguish between crotonylation and other lysine modifications. Another model (Adapt-ST) was trained to predict phosphorylation sites in SARS-CoV-2, and outperformed the equivalent state-of-the-art phosphorylation site prediction model. These results indicate that self-adaptive embedding features perform better than handcrafted features in capturing discriminative information; when used in attention architecture, this could be an effective way of identifying protein Kcr sites. Together, our Adapt framework (including learning embedding features and attention architecture) has a strong potential for prediction of other protein posttranslational modification sites.
更多查看译文
关键词
protein lysine crotonylation, phosphorylation, learning embedding features, convolutional neural networks, bidirectional LSTM, attention mechanism, PTMs prediction
AI 理解论文
溯源树
样例
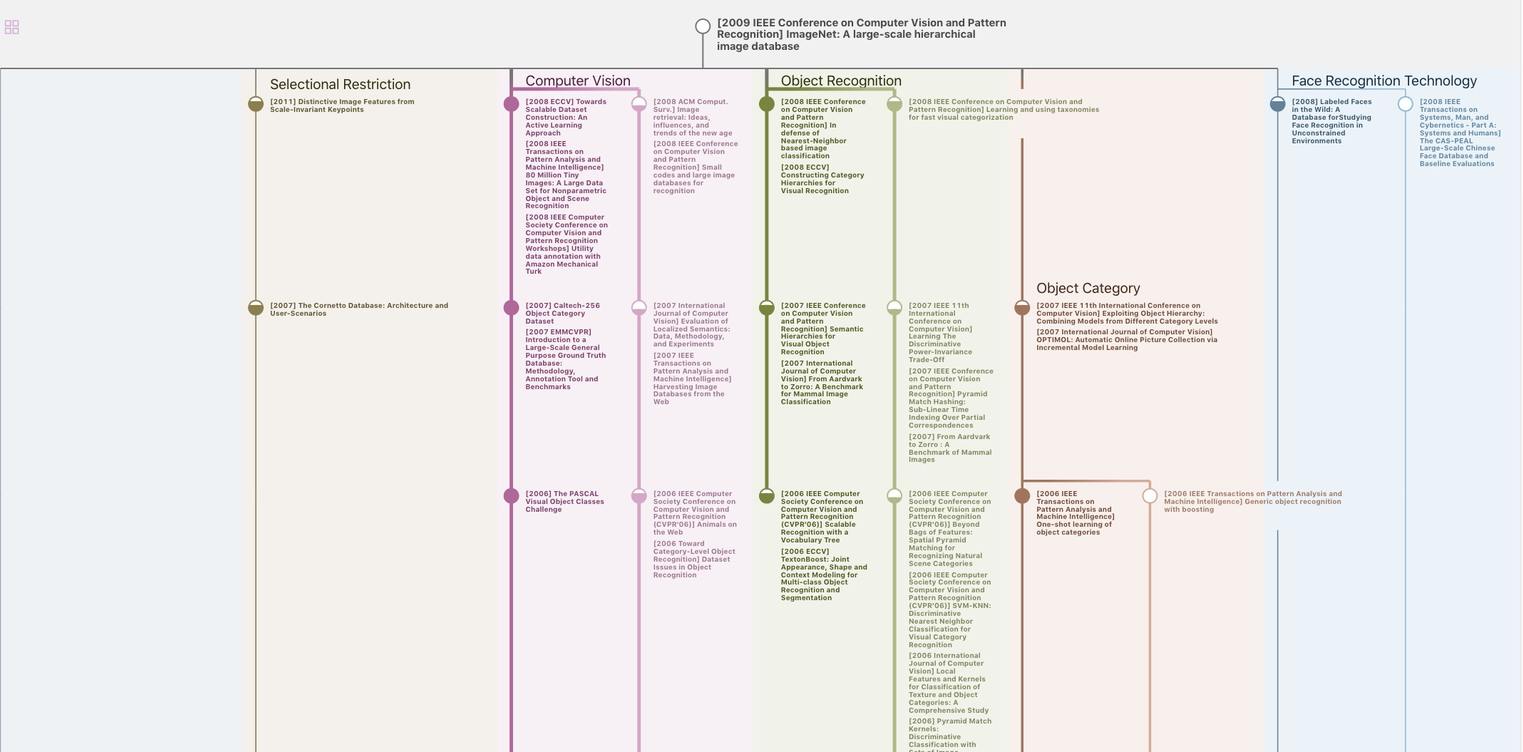
生成溯源树,研究论文发展脉络
Chat Paper
正在生成论文摘要