Stochastic Causal Programming for Bounding Treatment Effects
arxiv(2023)
摘要
Causal effect estimation is important for many tasks in the natural and social sciences. We design algorithms for the continuous partial identification problem: bounding the effects of multivariate, continuous treatments when unmeasured confounding makes identification impossible. Specifically, we cast causal effects as objective functions within a constrained optimization problem, and minimize/maximize these functions to obtain bounds. We combine flexible learning algorithms with Monte Carlo methods to implement a family of solutions under the name of stochastic causal programming. In particular, we show how the generic framework can be efficiently formulated in settings where auxiliary variables are clustered into pre-treatment and post-treatment sets, where no fine-grained causal graph can be easily specified. In these settings, we can avoid the need for fully specifying the distribution family of hidden common causes. Monte Carlo computation is also much simplified, leading to algorithms which are more computationally stable against alternatives.
更多查看译文
关键词
bounding treatment effects,treatment effects
AI 理解论文
溯源树
样例
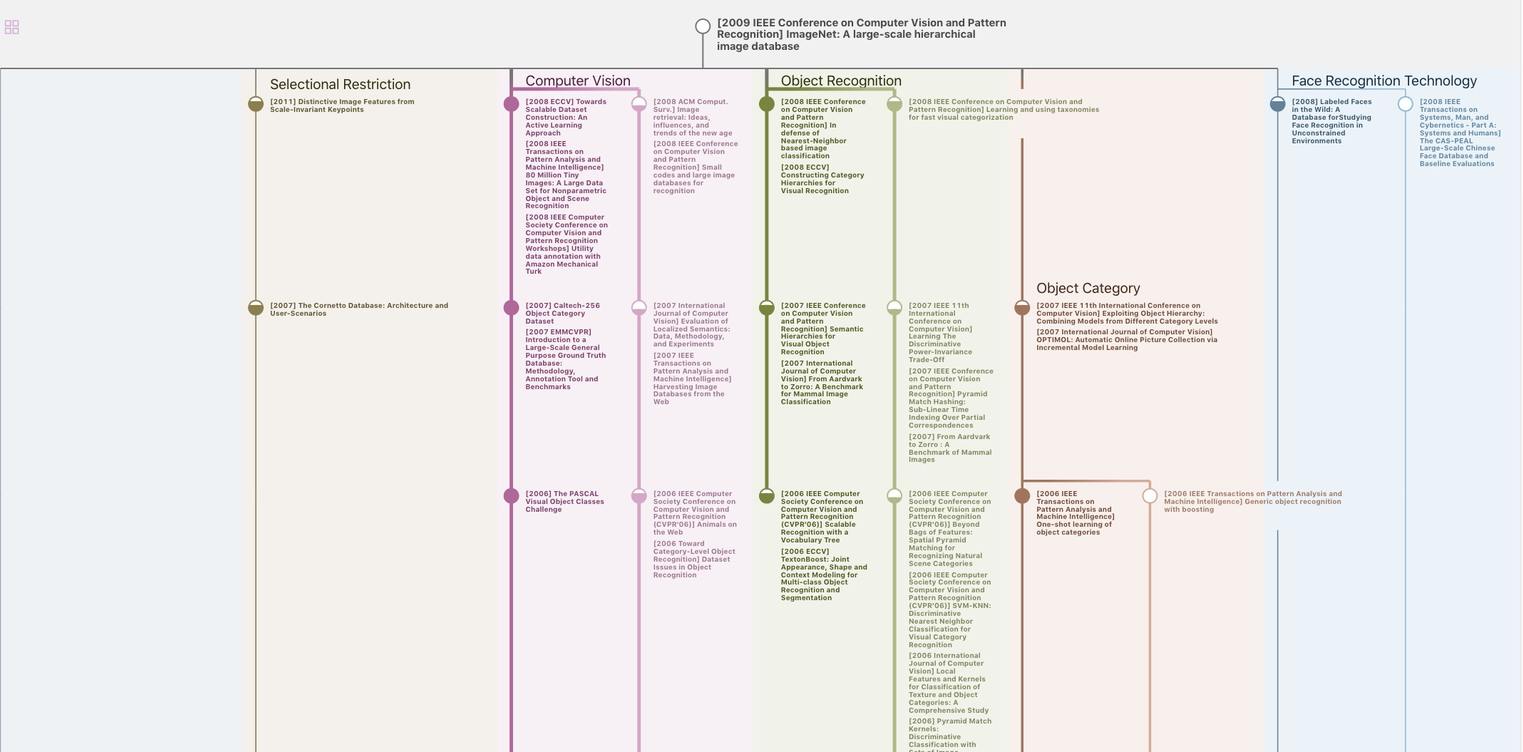
生成溯源树,研究论文发展脉络
Chat Paper
正在生成论文摘要