Combating Distribution Shift for Accurate Time Series Forecasting via Hypernetworks
arxiv(2022)
摘要
Time series forecasting has widespread applications in urban life ranging from air quality monitoring to traffic analysis. However, accurate time series forecasting is challenging because real-world time series suffer from the distribution shift problem, where their statistical properties change over time. Despite extensive solutions to distribution shifts in domain adaptation or generalization, they fail to function effectively in unknown, constantly-changing distribution shifts, which are common in time series. In this paper, we propose Hyper Time- Series Forecasting (HTSF), a hypernetwork-based framework for accurate time series forecasting under distribution shift. HTSF jointly learns the time-varying distributions and the corresponding forecasting models in an end-to-end fashion. Specifically, HTSF exploits the hyper layers to learn the best characterization of the distribution shifts, generating the model parameters for the main layers to make accurate predictions. We implement HTSF as an extensible framework that can incorporate diverse time series forecasting models such as RNNs and Transformers. Extensive experiments on 9 benchmarks demonstrate that HTSF achieves state-of-the-art performances.
更多查看译文
关键词
hypernetworks, time series forecasting, distribution shift
AI 理解论文
溯源树
样例
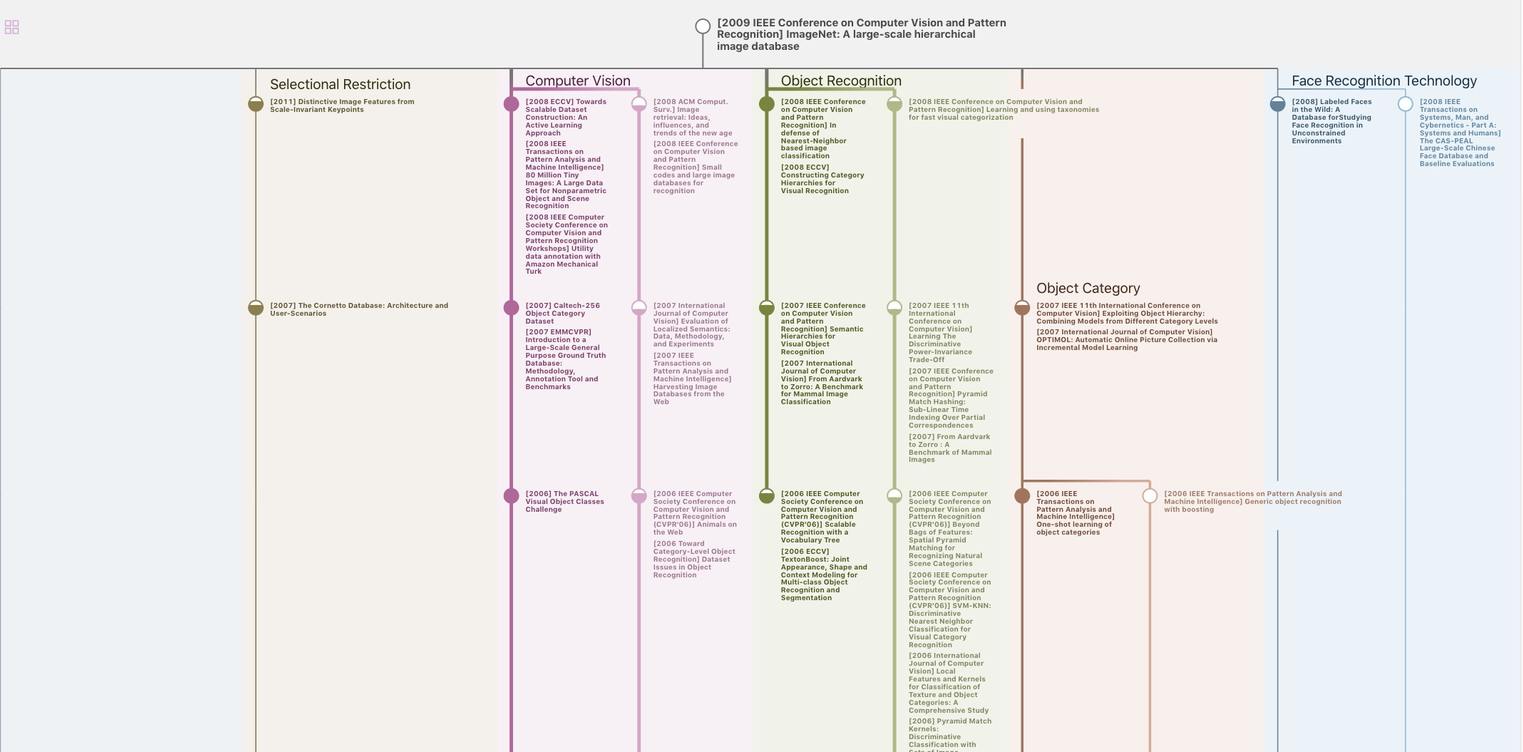
生成溯源树,研究论文发展脉络
Chat Paper
正在生成论文摘要